Sparse CNN and Deep Reinforcement Learning-Based D2D Scheduling in UAV-Assisted Industrial IoT Networks
IEEE TRANSACTIONS ON INDUSTRIAL INFORMATICS(2024)
摘要
Unmanned aerial vehicles (UAVs) have been widely applied in wireless communications because of its high flexibility and line-of-sight transmission. In this study, we develop low-complexity and robust device-to-device (D2D) link scheduling in UAV-assisted industrial-Internet-of-Things (IIoT) networks. First, we propose a sparse convolutional neural network (SCNN) model that uses the geographical map of transmission links as input. The model consists of three main blocks: 1) generic feature filtering, 2) speed-accuracy balancing, and 3) deep feature processing. Unlike other state-of-the-art methods, the proposed SCNN directly processes the geographical map collected using a connected UAV. Second, we propose a deep deterministic policy gradient-based reinforcement learning model that processes the output feature map from the SCNN to optimize the D2D scheduling decision and maximize the achievable system rate in the long run. Extensive simulations revealed that the proposed scheme significantly improved the achievable rate over other benchmark comparison schemes, such as transmitters and receivers density-based deep learning (DL), ResNet-based DL, VGGNet-based DL, random scheduling, and all-active schemes, respectively. The simulations also demonstrated that the proposed scheme reduces computational complexity. With reduced complexity and nearly optimal performance, the proposed solution can be more efficiently applied to large-scale and dense IIoT networks.
更多查看译文
关键词
Device-to-device communication,Industrial Internet of Things,Job shop scheduling,Interference,Neural networks,Deep learning,Computational complexity,Deep deterministic policy gradient (DDPG)-based reinforcement learning,geographical map,sparse convolutional neural network (SCNN),UAV-assisted industrial-Internet-of-Things (IIoT) networks,unmanned aerial vehicle (UAV)-assisted device-to-device (D2D) scheduling
AI 理解论文
溯源树
样例
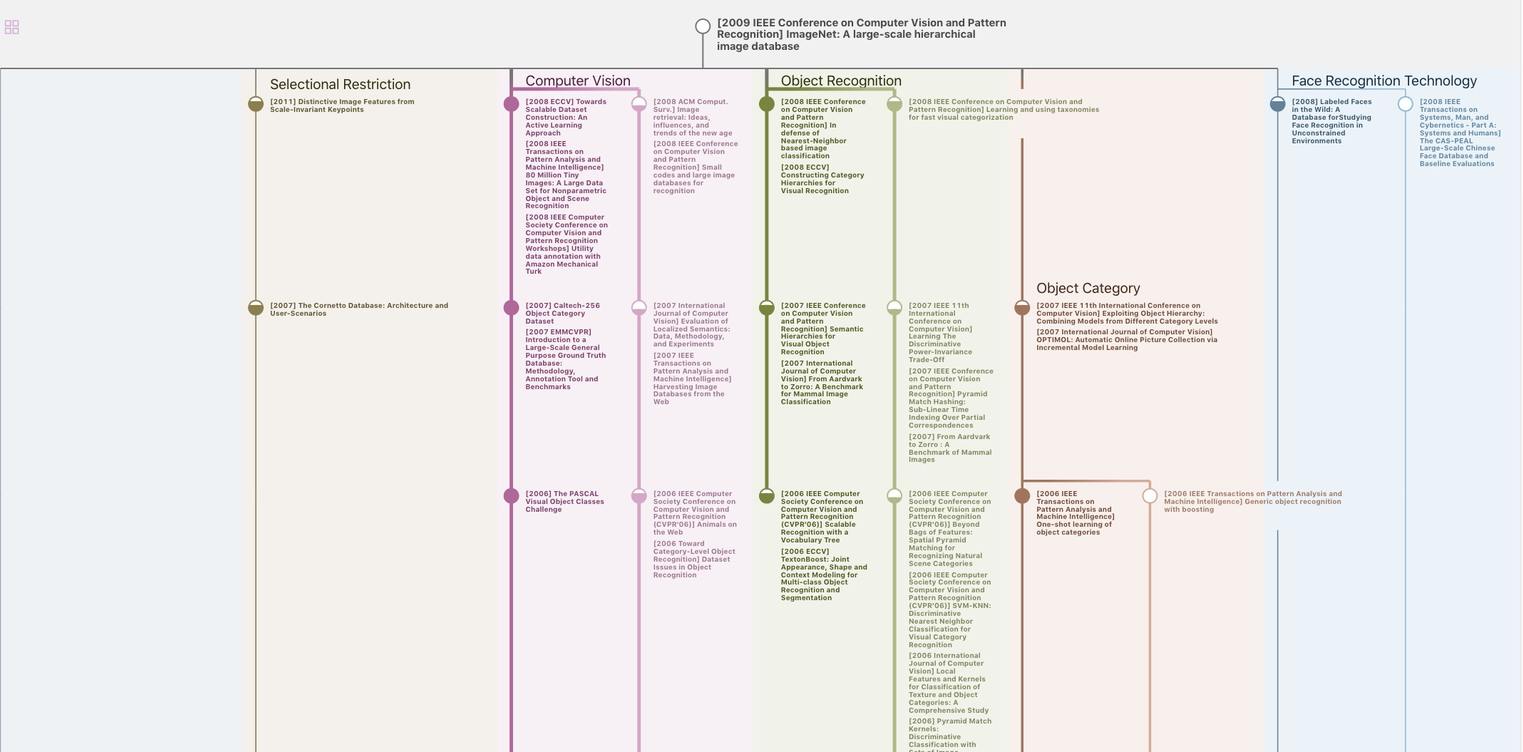
生成溯源树,研究论文发展脉络
Chat Paper
正在生成论文摘要