Constrained clustering with weak label prior
Frontiers of Computer Science(2024)
摘要
Clustering is widely exploited in data mining. It has been proved that embedding weak label prior into clustering is effective to promote its performance. Previous researches mainly focus on only one type of prior. However, in many real scenarios, two kinds of weak label prior information, e.g., pairwise constraints and cluster ratio, are easily obtained or already available. How to incorporate them to improve clustering performance is important but rarely studied. We propose a novel constrained Clustering with Weak Label Prior method (CWLP), which is an integrated framework. Within the unified spectral clustering model, the pairwise constraints are employed as a regularizer in spectral embedding and label proportion is added as a constraint in spectral rotation. To approximate a variant of the embedding matrix more precisely, we replace a cluster indicator matrix with its scaled version. Instead of fixing an initial similarity matrix, we propose a new similarity matrix that is more suitable for deriving clustering results. Except for the theoretical convergence and computational complexity analyses, we validate the effectiveness of CWLP through several benchmark datasets, together with its ability to discriminate suspected breast cancer patients from healthy controls. The experimental evaluation illustrates the superiority of our proposed approach.
更多查看译文
关键词
clustering,weak label prior,cluster ratio,pairwise constraints
AI 理解论文
溯源树
样例
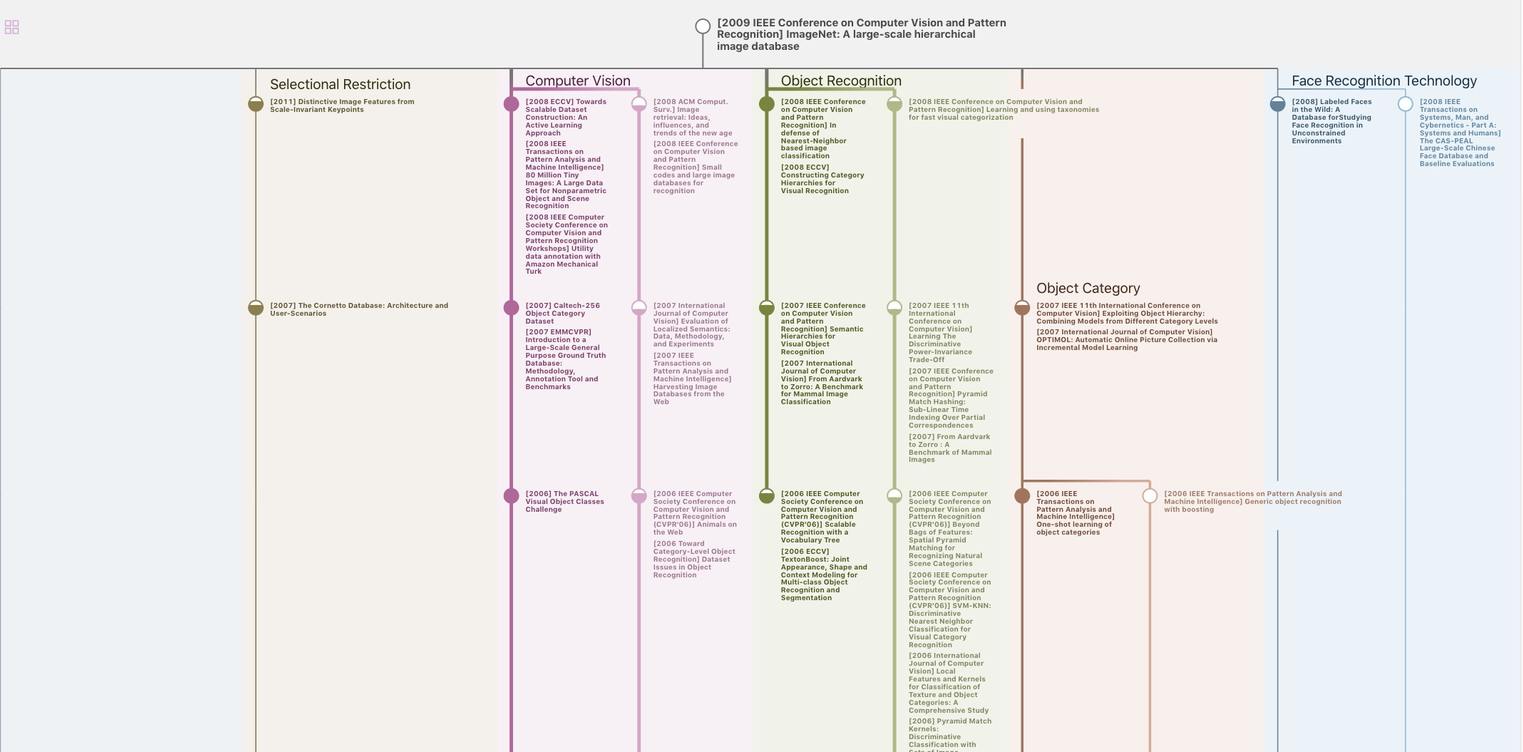
生成溯源树,研究论文发展脉络
Chat Paper
正在生成论文摘要