Improving Subseasonal-To-Seasonal Prediction of Summer Extreme Precipitation Over Southern China Based on a Deep Learning Method
GEOPHYSICAL RESEARCH LETTERS(2023)
摘要
The reliable Subseasonal-to-Seasonal (S2S) forecast of precipitation, particularly extreme precipitation, is critical for disaster prevention and mitigation, which however remains a great challenge for mission agencies and research communities. In this study, a deep learning method based on U-Net with additional atmospheric factor forecasts included is proposed to improve S2S quantitative forecasts of summer precipitation over Southern China. The weighted loss function integrated by mean square error and threat score is introduced to capture extreme precipitation more precisely. Generally, the U-Net model shows promising results in both general statistics and extreme events. Predictor importance analyses show that the U-Net forecast skills at the 1-week lead time mainly arise from synchronous precipitation forecasts, but the contributions made by atmospheric factor forecasts rise rapidly with increasing lead times. Therefore, the channel combining numerical weather prediction model and deep learning framework is demonstrated promising in S2S precipitation forecasts. The Subseasonal-to-Seasonal (S2S) forecast of precipitation, in particular of the extreme precipitation events, from 2 weeks to a season in advance is challenging despite increasing social demand and scientific interest for accurate and dependable predictions. In this study, the U-Net based deep learning method is employed with additional atmospheric variable forecasts (e.g., wind and specific humidity at multiple levels) included to correct the S2S forecasts of summer precipitation derived from a numerical weather prediction model over Southern China. It is demonstrated that the U-Net improves the forecast performance in both general statistics and extreme events and shows a pronounced superiority to the traditional statistical postprocessing method. Thus, combining numerical models and deep learning is very promising in subseasonal precipitation forecasts and can also be applied to the routine forecast of other atmospheric and ocean phenomena in the future. The Subseasonal-to-Seasonal prediction of summer precipitation over southern China is improved with a U-Net based deep learning methodThe U-Net demonstrated promising performance in both general statistics and extreme events and shows superiority to the quantile mapping benchmarkThe model skills arise from precipitation itself at the early stage, while atmospheric factors play important roles at longer lead times
更多查看译文
AI 理解论文
溯源树
样例
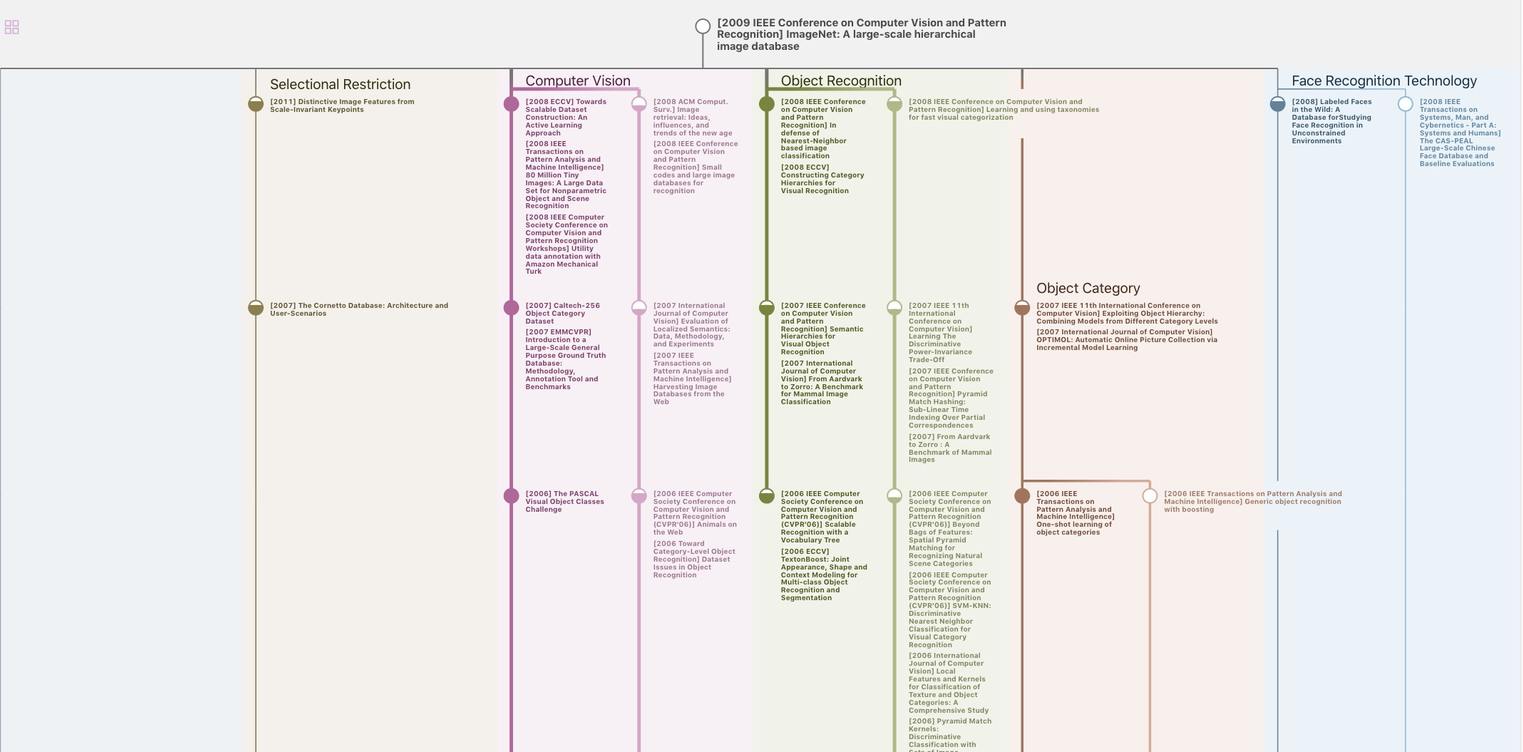
生成溯源树,研究论文发展脉络
Chat Paper
正在生成论文摘要