Inverse design of high entropy alloys using a deep interpretable scheme for materials attribution analysis
JOURNAL OF ALLOYS AND COMPOUNDS(2024)
摘要
High entropy alloys (HEAs) have gained significant attention due to their unique combinations of properties and extensive compositional space. However, designing HEAs with specific properties has become increasingly complex and challenging. To address this issue, a Deep Interpretable Scheme for Material Attribution Analysis (DISMAA) was developed to systematically uncover the composition-structure-property (CSP) relationships of HEAs and generate novel compositions. The approach combines a deep generative model, trained with limited data, to create a continuous composition space of AlCoCrFeMnNi high entropy alloys, and additional deep learning models, trained with more data, to predict the corresponding phase and properties. Attribution and sensitivity analysis techniques are used to analyze the contribution of each element to different materials properties and phases. The distributions of composition, phase, mechanical property, and contribution of elements are visualized in the latent space, providing a novel and intuitive approach to analyze CSP relationships using deep learning. The efficacy of the method was validated, demonstrating the interpretability and rationality of the deep neural network model for material inverse design applications. This approach can be applied to tailor multiple properties and manufacturing parameters in various materials systems, providing scientists with a comprehensive context for designing HEAs.
更多查看译文
关键词
Generative model,Attribution analysis,Latent space,High entropy alloy,Material design
AI 理解论文
溯源树
样例
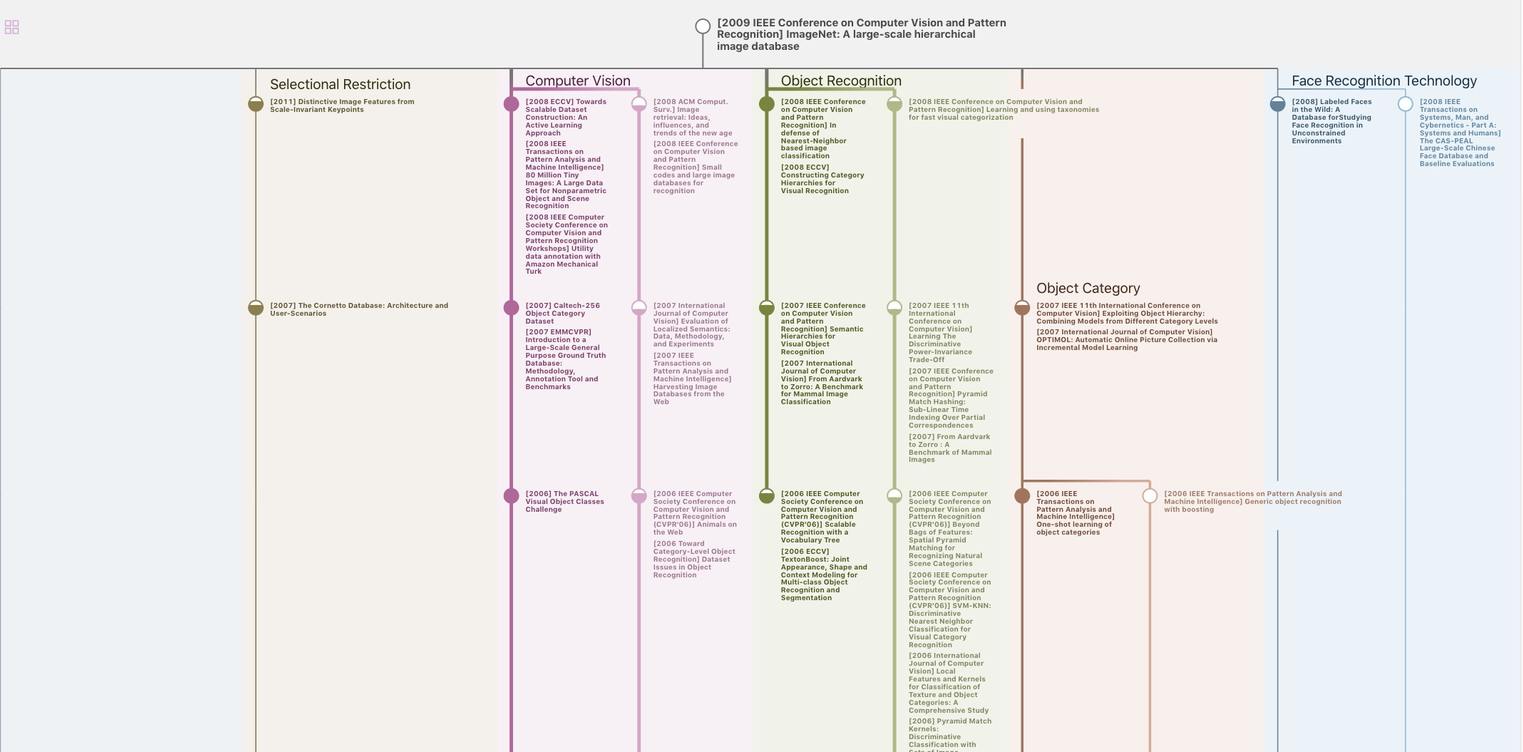
生成溯源树,研究论文发展脉络
Chat Paper
正在生成论文摘要