BCNN: Backpropagation CNN-Based fully unsupervised skull stripping for accurate brain segmentation
BIOMEDICAL SIGNAL PROCESSING AND CONTROL(2024)
摘要
Brain skull stripping is an essential step before performing the segmentation. It leads to better performance and less computational load on the model due to the elimination of redundant features from the image. However, the preparation of the skull-stripped ground truth brain images by the experts is a very tedious task and may lead to human errors. In this article, a fully unsupervised approach to brain extraction has been proposed. The cascaded loss function is used and is tuned for better segmentation. The number of connected components is also tuned in each type of dataset. The cascaded loss function is a combination of focal loss (FL) and dice loss (DL). To address the class imbalance issue, the leaky ReLU activation function is used. Enhancement of the brain image has been performed before extraction which yielded better performance. In comparison with other methods, the proposed backpropagation-based convolutional neural network (BCNN) work gives better qualitative and quantitative outcomes on four out of seven parameters. The dice similarity coefficient (DSC) of the proposed model is 0.89 which is the highest as compared to the other models for brain extraction. The specificity of the model is 0.998 with 97.21 % accuracy. The undersegmentation error is reduced to 1.2002 using the cascaded loss function. The proposed work has been evaluated on four brain image datasets. It has been found that the proposed model extracts the brain from the skull and also the white matter, gray matter, and cerebrospinal fluid. Therefore, it has been noted that the proposed model will be beneficial for efficient brain skull stripping without the availability of ground truth data.
更多查看译文
关键词
Brain extraction,Brain tissues,Focal loss,Skull stripping,Unsupervised image segmentation
AI 理解论文
溯源树
样例
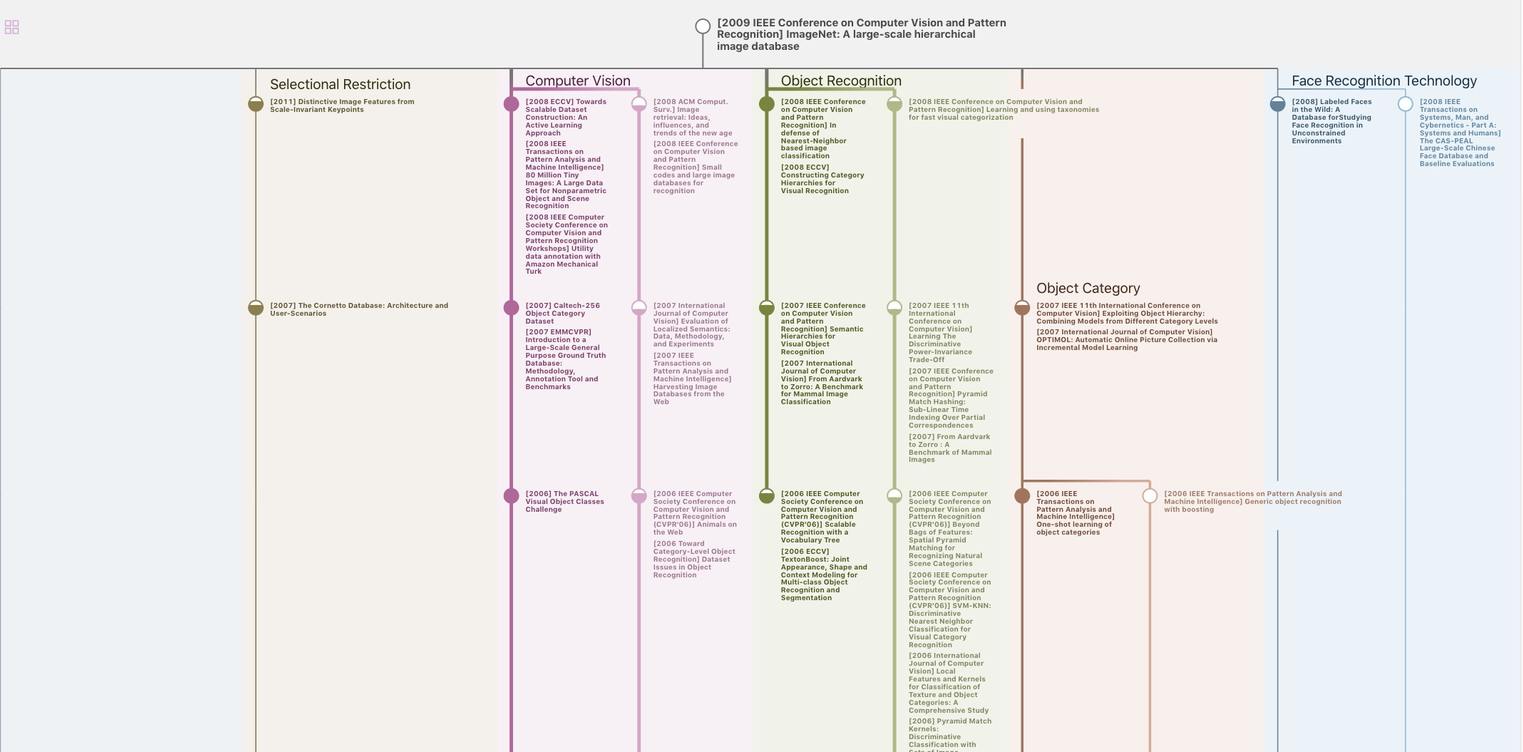
生成溯源树,研究论文发展脉络
Chat Paper
正在生成论文摘要