Narrowing Domain Gaps With Bridging Samples for Generalized Face Forgery Detection.
IEEE Trans. Multim.(2024)
摘要
Face forgery technology has developed rapidly, causing severe security issues in society. Recently, with the continuous emergence of forgery techniques and types, most forensics methods suffer from the generalization problem. In particular, it is difficult for existing generalized methods to detect fake faces with unseen fake types. The reason is that the distribution gaps among cross-forgery types are too large. In this paper, we propose a novel generalized framework to narrow large gaps based on bridging cross-domain alignment to solve this problem. Specifically, our framework consists of three key steps: preventing, bridging and aligning distribution gaps. Firstly, in the feature mining stage, taking advantage of the ability of Instance Normalization (IN) to better tolerate domain gaps, we design Adaptive Batch and Instance Normalization (ABIN) to replace the commonly used BN to adaptively extract features to preliminarily prevent domain gaps. Secondly, we propose to generate bridging samples distributed among the inter-domains to fill large gaps based on progressive linear interpolation operation. Finally, with the help of bridging samples, the cross-domain alignment is performed to better narrow distribution gaps to refine data distribution, which helps to learn a more generalized framework. Extensive experiments show that our proposed framework achieves the state-of-the-art generalized performance.
更多查看译文
关键词
Generalized face forgery detection,domain gaps prevention,bridging samples generation,cross-domain alignment
AI 理解论文
溯源树
样例
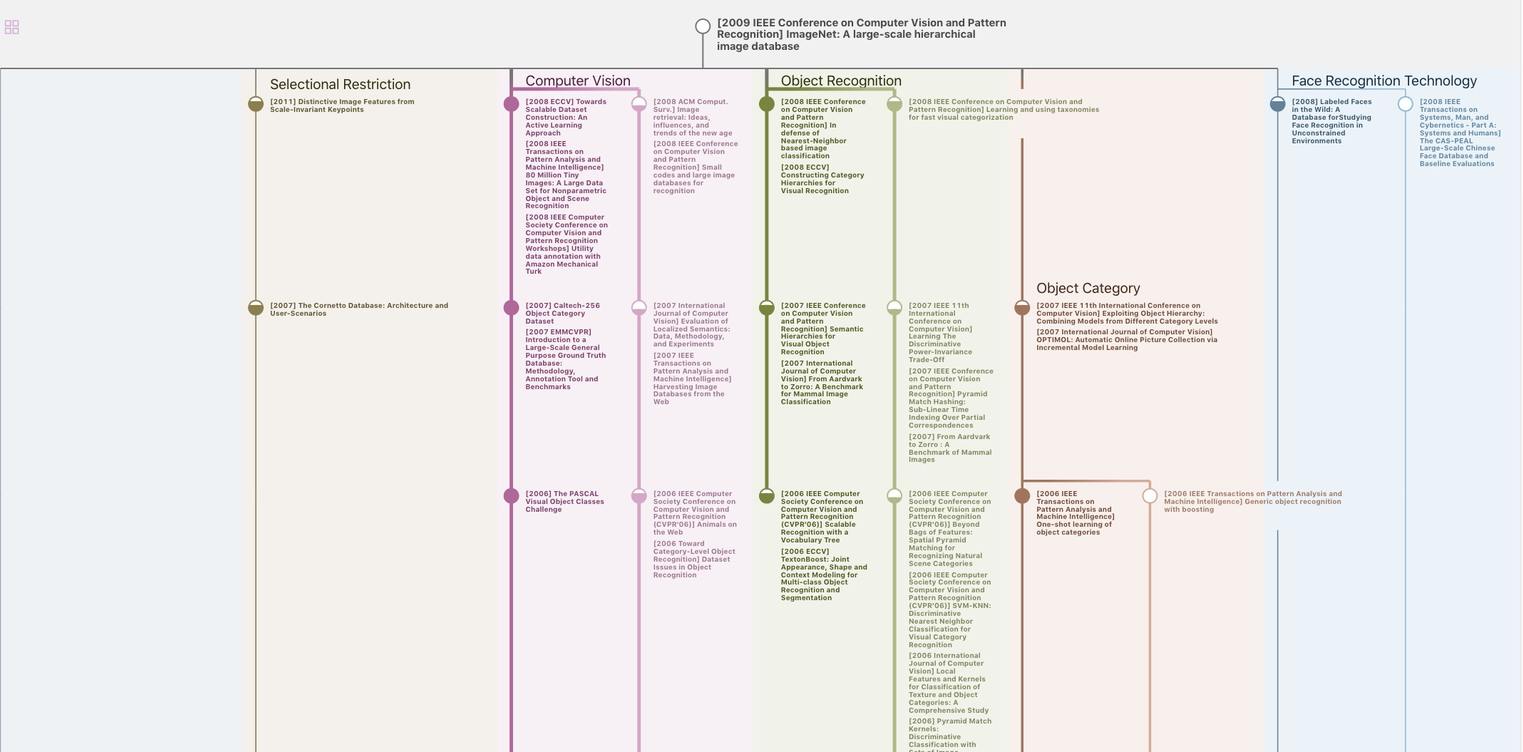
生成溯源树,研究论文发展脉络
Chat Paper
正在生成论文摘要