Abstract 5379: Deep learning enables label-free tracking of heterogeneous subpopulations
Cancer Research(2023)
摘要
Abstract Observing and quantifying the proliferation of subpopulations in cancer is key to understanding how heterogeneous groups of cells interact and respond to therapy, their environment, and each other. Prior research has demonstrated that cell-state properties such as metastatic potential and genotype perturbation are encoded in cellular morphology and can be identified with various machine-learning approaches. This encoding spans multiple imaging modalities such as brightfield, phase contrast, and stained whole-slide images, and phenotype prediction can be accomplished using both classical and deep machine learning methods. Here we show that not only do these prediction capabilities extend to transcriptomic subpopulations, but that they can be used to track these populations in high throughput longitudinal live-cell imaging experiments. Using single-cell RNA sequencing, we observed that, among untreated MDA-MB-231 triple negative breast cancer cells, there exist two transcriptomically distinct populations of cells. Using fluorescence-activated cell sorting based on the differentially expressed surface marker ESAM, we isolated these subpopulations and fluorescently labeled them with mCherry or GFP depending on their transcriptomic cluster. Cells were then grown in monoculture or coculture and imaged every 4 hours at 20x resolution. To identify the associated transcriptomic cluster, we trained an instance segmentation algorithm, Mask R-CNN, to both segment and classify cells. We find that, despite being derived from the same cell line, these phenotypes can be predicted using phase contrast images alone. These results demonstrate that cellular phenotypes manifested by distinctive RNA expression signatures can now be surveilled in a high-throughput manner across multiple samples and conditions without further RNA sequencing or biomarker labeling. We anticipate that this methodology of high throughput tracking will be applicable to other heterogeneous subpopulations, such as isolated therapy-resistant or sensitive cells. Tracking transcriptomically distinct populations using only high-throughput imaging will increase the granularity of population analysis and enable more rapid assessment of cancer cell evolutionary dynamics. Citation Format: Tyler Jost, Andrea Gardner, Amy Brock. Deep learning enables label-free tracking of heterogeneous subpopulations. [abstract]. In: Proceedings of the American Association for Cancer Research Annual Meeting 2023; Part 1 (Regular and Invited Abstracts); 2023 Apr 14-19; Orlando, FL. Philadelphia (PA): AACR; Cancer Res 2023;83(7_Suppl):Abstract nr 5379.
更多查看译文
关键词
heterogeneous subpopulations,deep learning,label-free
AI 理解论文
溯源树
样例
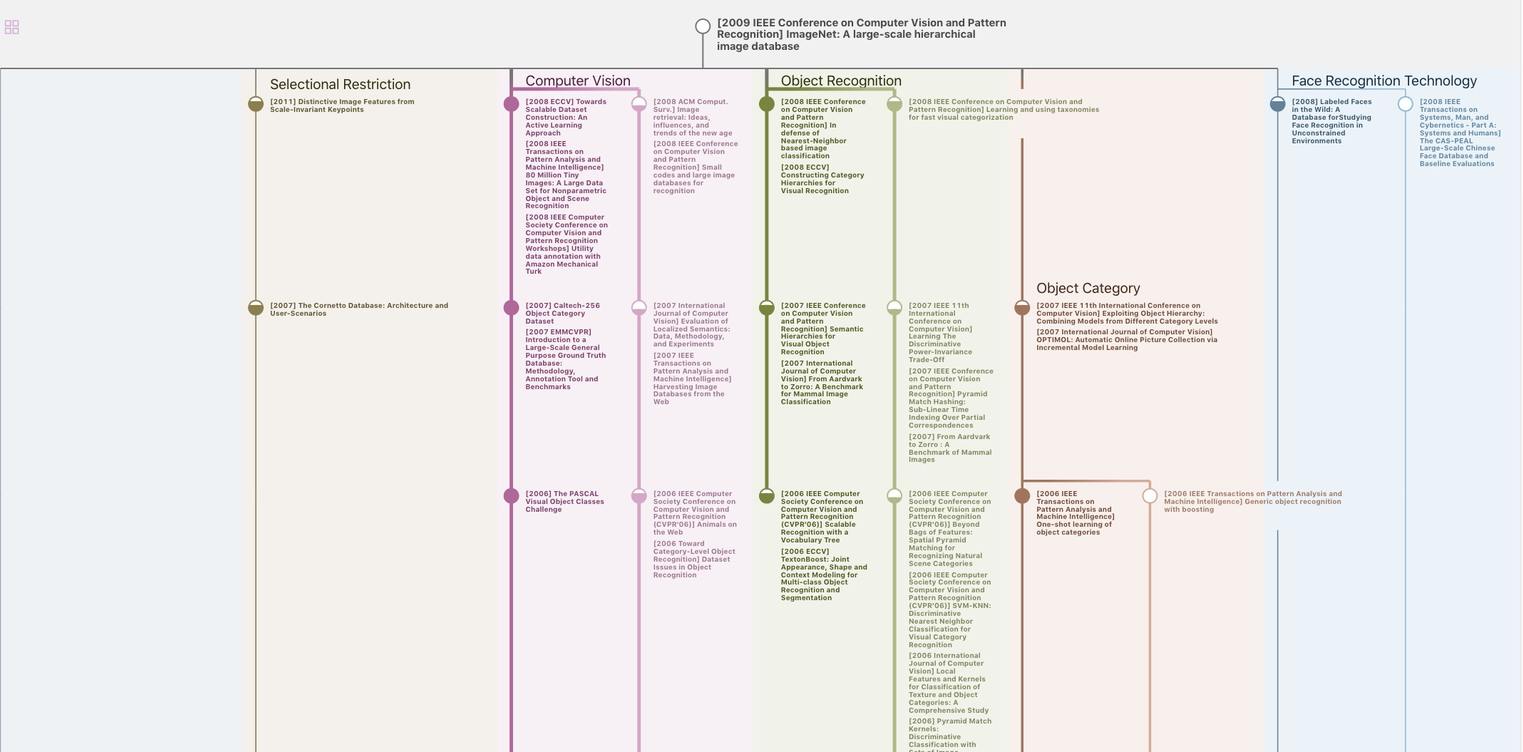
生成溯源树,研究论文发展脉络
Chat Paper
正在生成论文摘要