A Transfer Learning Method to Generate Synthetic Synoptic Magnetograms
SPACE WEATHER-THE INTERNATIONAL JOURNAL OF RESEARCH AND APPLICATIONS(2024)
摘要
Current magnetohydrodynamics (MHD) models largely rely on synoptic magnetograms, such as the ones produced by the Global Oscillation Network Group (GONG). Magnetograms are currently available mostly from the front side of the Sun, which significantly reduces the accuracy of MHD modeling. Extreme Ultraviolet (EUV) images can instead be obtained from other vantage points. To investigate the potential, we explore the possibility of using EUV information from the Atmospheric Imaging Assembly (AIA) to directly generate the input for the state-of-the-art 3D MHD model European Heliospheric FORecasting Information Asset (EUHFORIA). Toward this goal, we develop a method called Transfer-Solar-GAN which combines a conditional generative adversarial network with a transfer learning approach to overcome training data set limitations. The source domain data set is constructed from multiple pairs of the central portion of co-registered AIA and Helioseismic and Magnetic Imager (HMI) line of sight (LOS) full-disk images, while the target domain is constructed from pairs of portions of AIA and GONG sine-latitude synoptic maps that we call segments. We evaluate Transfer-Solar-GAN by comparing modeled and measured solar wind velocity and magnetic field density parameters at the L1 Lagrange point and along the Parker Solar Probe (PSP) trajectory which were determined with EUHFORIA using both empirical GONG and artificial-intelligence (AI)-synthetic synoptic magnetograms as inputs. Our results demonstrate that the Transfer-Solar-GAN model can provide the necessary information to run solar physics models by EUV information. Our proposed model is trained with only 528 paired image segments and enforces a reliable data division strategy. Space weather predictions are strongly dependent on the different models of the solar wind and Coronal Mass Ejections (CMEs). Presently, most coronal models are initialized with magnetograms which are obtained through a complex inversion process and are available only from a limited number of sources. On the other hand, Extreme Ultraviolet (EUV) images are available from several vantage points. We develop a deep learning method called Transfer-Solar-GAN that can generate approximate GONG synoptic magnetograms directly from only hundreds of EUV synoptic maps. We tested the model on the state-of-the-art magnetohydrodynamics (MHD) model called the European Heliospheric FORecasting Information Asset (EUHFORIA) by comparing its outputs at the distance of Lagrange point L1 and along the PSP trajectory using both real Global Oscillation Network Group (GONG) magnetograms and artificial-intelligence (AI)-synthetic synoptic maps. The results show that the simulated outputs using AI-synthetic magnetograms are comparable with the values calculated from real GONG magnetograms and the related observations, demonstrating the possibility of leveraging EUV information to generate the input of MHD models. We use a conditional generative adversarial network and transfer learning approach to synthesize synoptic magnetogram maps using EUV imagesThe model can generate synthetic magnetograms similar to empirical GONG maps with a limited number of paired AIA304 angstrom and GONG segmentsThe EUFORIA model was able to reproduce the solar wind with comparable fidelity using either a synthetic or standard synoptic magnetogram
更多查看译文
关键词
synoptic magnetogram,solar wind model,EUV to magnetogram image translation,transfer learning
AI 理解论文
溯源树
样例
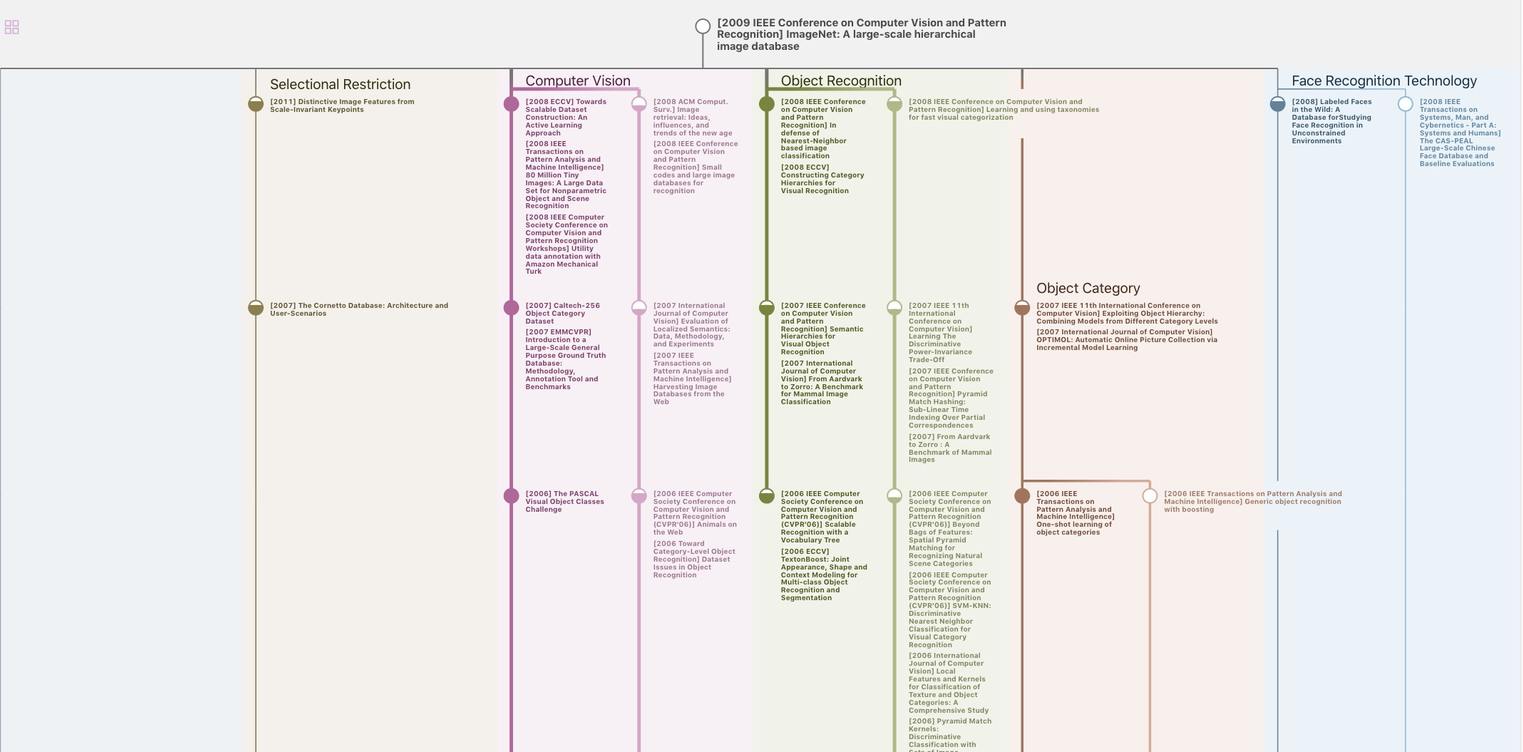
生成溯源树,研究论文发展脉络
Chat Paper
正在生成论文摘要