Emerging Synergies in Causality and Deep Generative Models: A Survey
arXiv (Cornell University)(2023)
摘要
In the field of artificial intelligence (AI), the quest to understand and model data-generating processes (DGPs) is of paramount importance. Deep generative models (DGMs) have proven adept in capturing complex data distributions but often fall short in generalization and interpretability. On the other hand, causality offers a structured lens to comprehend the mechanisms driving data generation and highlights the causal-effect dynamics inherent in these processes. While causality excels in interpretability and the ability to extrapolate, it grapples with intricacies of high-dimensional spaces. Recognizing the synergistic potential, we delve into the confluence of causality and DGMs. We elucidate the integration of causal principles within DGMs, investigate causal identification using DGMs, and navigate an emerging research frontier of causality in large-scale generative models, particularly generative large language models (LLMs). We offer insights into methodologies, highlight open challenges, and suggest future directions, positioning our comprehensive review as an essential guide in this swiftly emerging and evolving area.
更多查看译文
关键词
deep generative models,causality,synergies
AI 理解论文
溯源树
样例
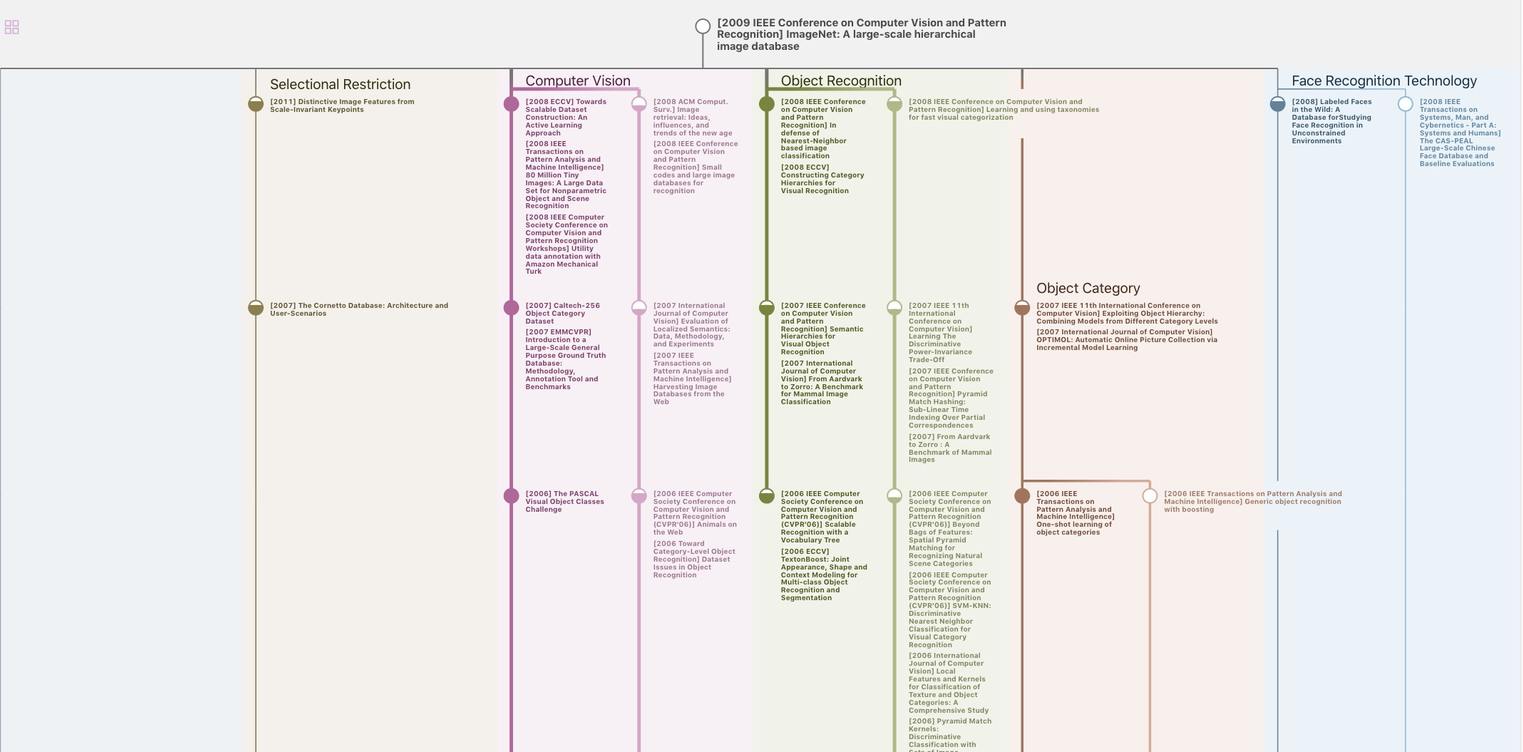
生成溯源树,研究论文发展脉络
Chat Paper
正在生成论文摘要