Performance Assessment of Chemical Kinetics Neural Ordinary Differential Equations in Pairwise Mixing Stirred Reactor
ASME open journal of engineering(2023)
摘要
Abstract The present study aims to assess the potential of the neural ordinary differential equations (NODE) network for reliable and computationally efficient implementation of chemistry in combustion simulations. Investigations are performed using a hydrogen-air pairwise mixing stirred reactor (PMSR). The PMSR is a zero-dimensional case affordable to study combustion chemistry entailing a similar numerical solution procedure as probability density function methods for turbulent combustion simulations. A systematic approach is presented to apply the NODE, solely trained on canonical constant pressure homogeneous reactor data, to predict complex chemistry and mixing interactions in PMSR. The reactor involves combustion of hydrogen in air described by a finite-rate mechanism with 9 chemical species and 21 reaction steps. The NODE network is shown to accurately capture the evolution of thermochemical variables for different mixing and chemical timescales. It also exhibits a significant reduction in numerical stiffness resulting in improving the computational efficiency and enabling the use of explicit solvers for the integration of chemical kinetics. The assessment results based on PMSR show that compared to direct integration of detailed kinetics, the NODE can achieve significant computational time speedup for a comparable accuracy.
更多查看译文
关键词
kinetics,differential equations
AI 理解论文
溯源树
样例
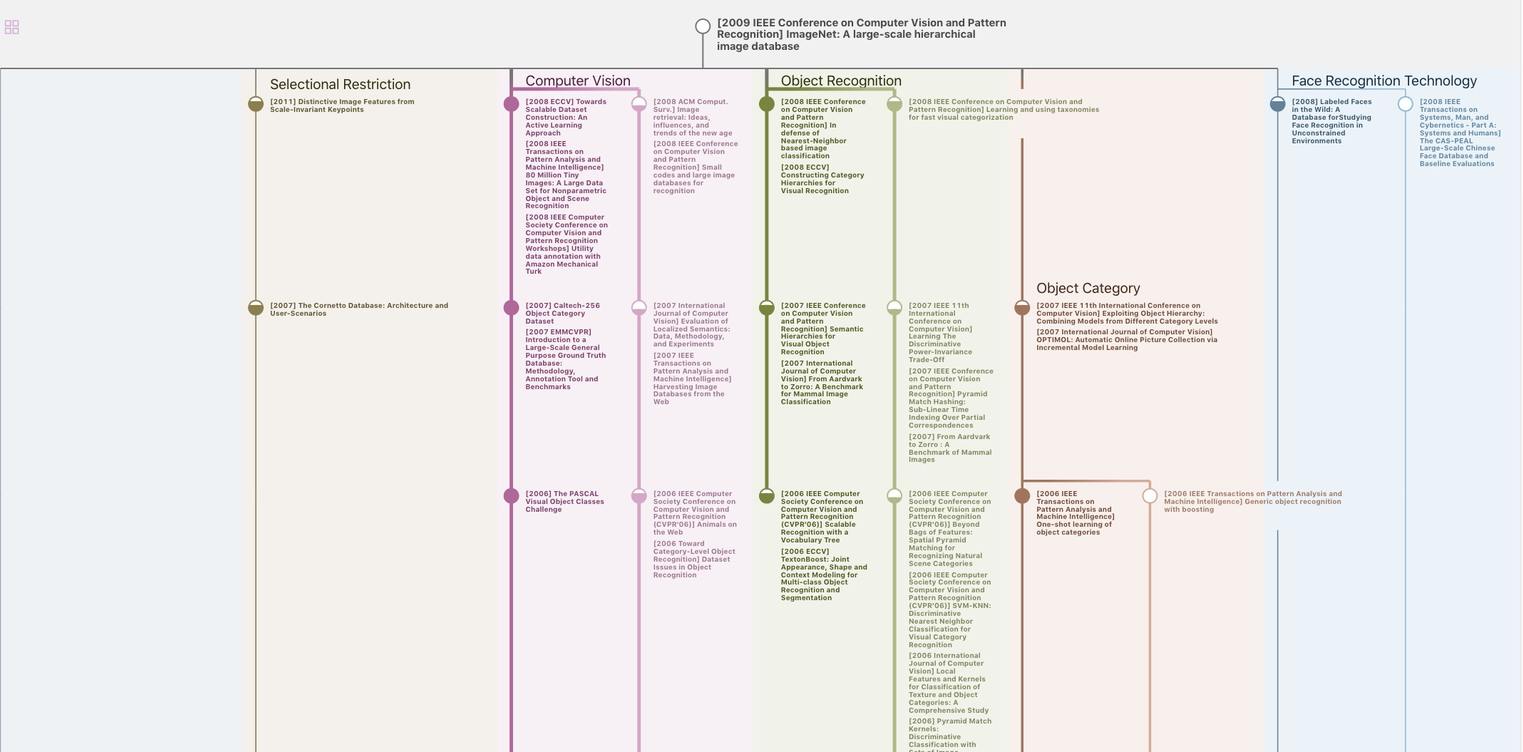
生成溯源树,研究论文发展脉络
Chat Paper
正在生成论文摘要