Multi-frame-based Cross-domain Image Denoising for Low-dose Computed Tomography
arXiv (Cornell University)(2023)
摘要
Computed tomography (CT) has been used worldwide for decades as one of the most important non-invasive tests in assisting diagnosis. However, the ionizing nature of X-ray exposure raises concerns about potential health risks such as cancer. The desire for lower radiation dose has driven researchers to improve the reconstruction quality, especially by removing noise and artifacts. Although previous studies on low-dose computed tomography (LDCT) denoising have demonstrated the potential of learning-based methods, most of them were developed on the simulated data collected using Radon transform. However, the real-world scenario significantly differs from the simulation domain, and the joint optimization of denoising with the modern CT image reconstruction pipeline is still missing. In this paper, for the commercially available third-generation multi-slice spiral CT scanners, we propose a two-stage method that better exploits the complete reconstruction pipeline for LDCT denoising across different domains. Our method makes good use of the high redundancy of both the multi-slice projections and the volumetric reconstructions while avoiding the collapse of information in conventional cascaded frameworks. The dedicated design also provides a clearer interpretation of the workflow. Through extensive evaluations, we demonstrate its superior performance against state-of-the-art methods.
更多查看译文
关键词
computed
AI 理解论文
溯源树
样例
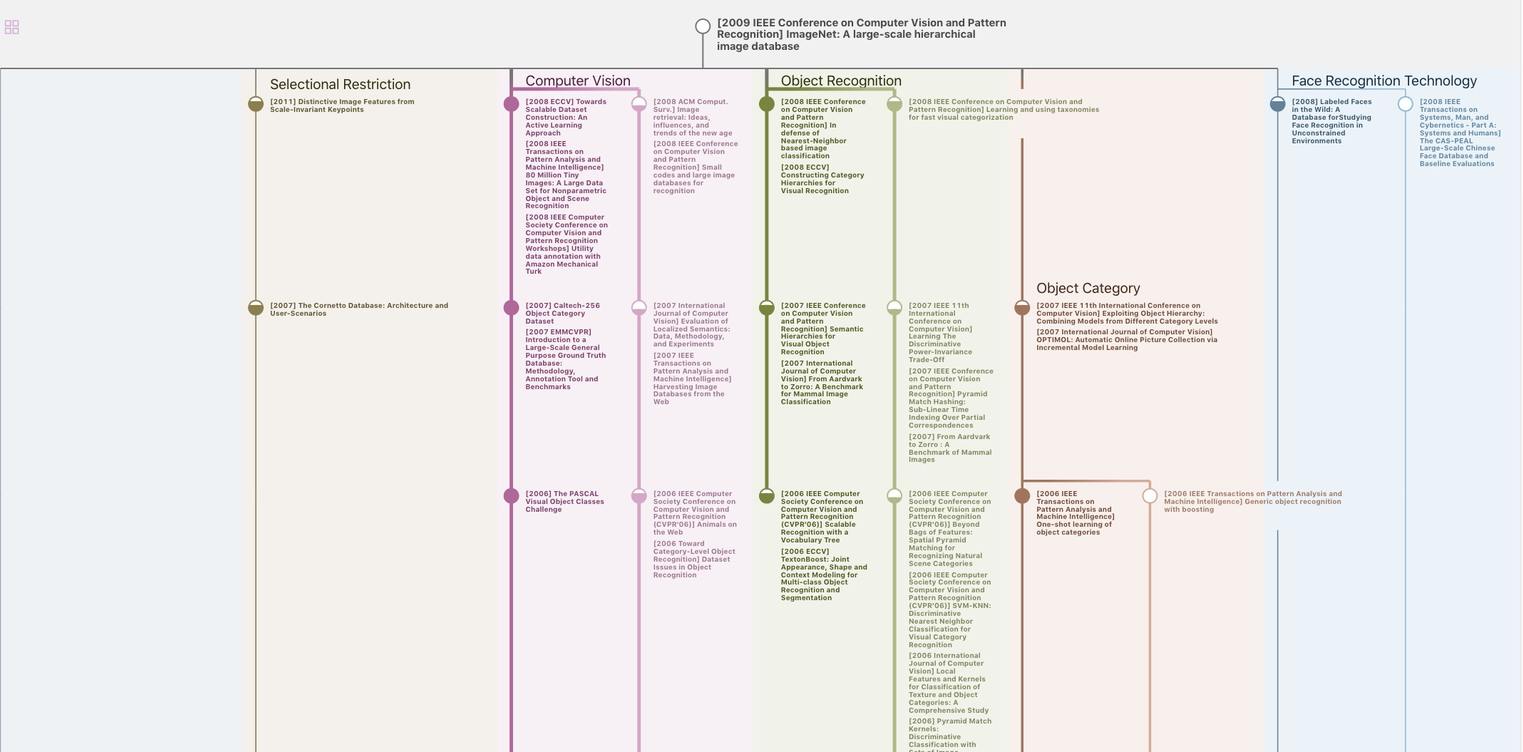
生成溯源树,研究论文发展脉络
Chat Paper
正在生成论文摘要