HairManip: High quality hair manipulation via hair element disentangling
PATTERN RECOGNITION(2024)
摘要
Hair editing is challenging due to the complexity and variety of hair materials and shapes. Existing methods employ reference images or user-painted masks to edit hair and have achieved promising results. However, discrepancies in color and shape between the source and target hair can occasionally result in unrealistic results. Therefore, we propose a new hair editing method named HairManip, which decouples the hair information from the input source image into shape and color components. We then train hairstyle and hair color editing sub-networks to handle this complex information independently. To further enhance editing efficiency and accuracy, we introduce a latent code preprocessing module that effectively extracts meaningful features from hair regions, thereby improving the model's editing capabilities. The experimental results demonstrate that our method achieves significant results in editing accuracy and authenticity, thanks to the carefully designed network structure and loss functions. Code can be found at https://github.com/Zlin0530/ HairManip.
更多查看译文
关键词
Hair editing networks,Image generation,Hair manipulation,Generative adversarial networks,Deep learning
AI 理解论文
溯源树
样例
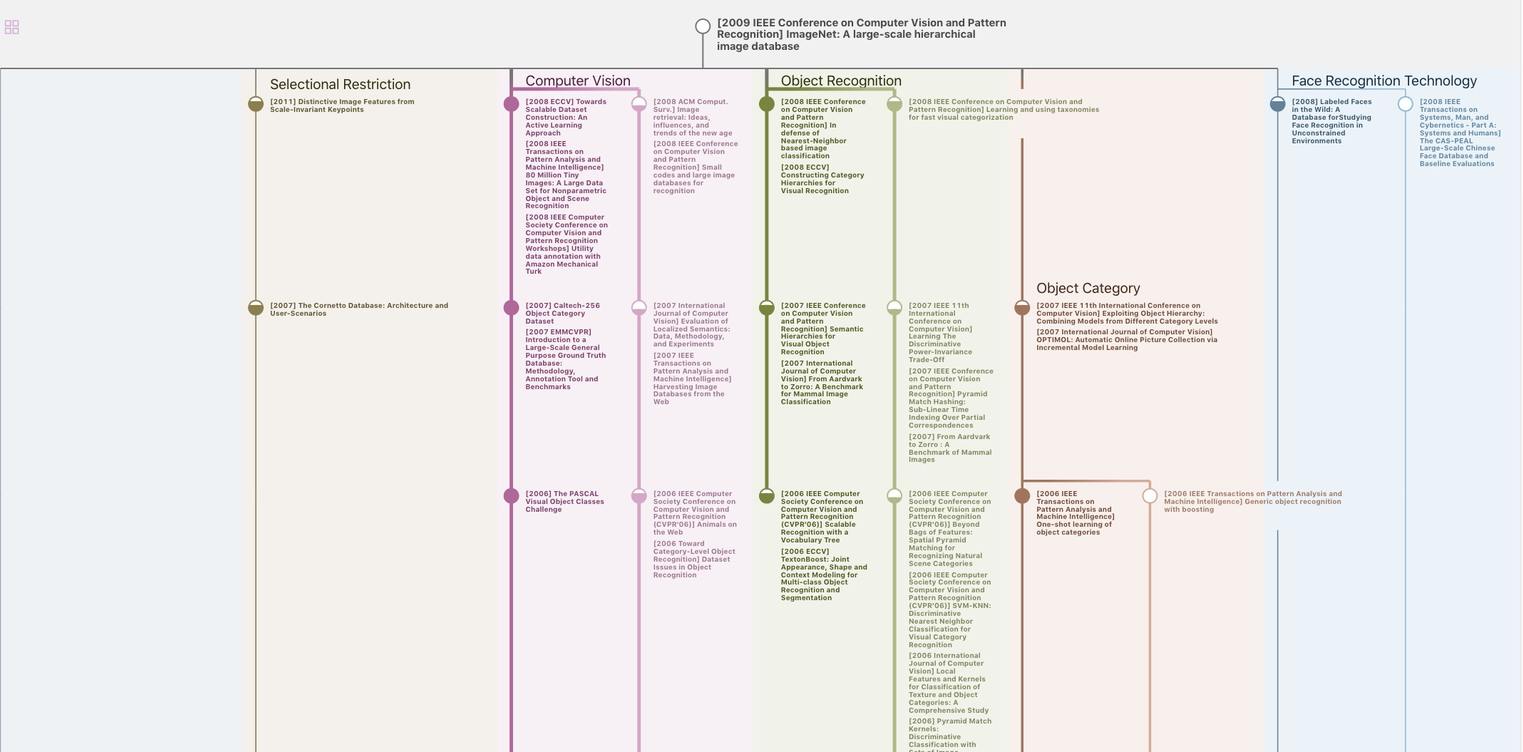
生成溯源树,研究论文发展脉络
Chat Paper
正在生成论文摘要