Using Theoretical and Realized Accuracies to Estimate Changes in Heritabilities
JOURNAL OF ANIMAL SCIENCE(2023)
摘要
Abstract Under genomic selection, heritabilities for strongly selected traits are reduced, and antagonism between production and fitness traits can intensify. However, estimating genetic parameters by established methods such as REML or Bayesian with large genomic data is computationally expensive or unfeasible. In this study, we investigated the use of theoretical and realized accuracies to estimate heritabilities. The first dataset for pigs included 161k phenotypic records for a growth trait (GT), 27k phenotypic records for a fitness trait (FT), and 55k animals were genotyped. The second dataset for broilers contained 820K phenotypes for a growth trait (GT), and 154k animals were genotyped. Theoretical accuracies (r) were calculated as r = sqrt(Nh2/(Nh2+Me)) assuming the number of independent chromosome segments (Me) of 5k and the number of observations (N) equal to the number of genotypes or phenotypes, whichever was smaller. Estimated accuracies were calculated by the linear regression (LR) method. Assumed heritabilities were recent estimates for pigs (0.21 for GT and 0.04 for FT) and an older estimate for broilers (0.30). For the pig dataset, the theoretical and realized accuracies were similar: 0.82 and 0.84 for GT, and 0.42 and 0.43 for FT, suggesting that the assumed heritabilities were realistic. For the broiler dataset, the theoretical and realized accuracies differed: 0.94 and 0.56, indicating possible large differences between real and assumed heritabilities. Both accuracies could be made equal at 0.89 by assuming a heritability of 0.13. Such a heritability was also found to provide a realistic estimate of the genetic gain with recent data. Comparisons of theoretical and realized accuracies may be used as a tool to estimate changing heritabilities over successive generations at a low cost. Similar formulas may enable low-cost estimation of genetic correlations.
更多查看译文
关键词
accuracy,genomic selection,parameter estimation
AI 理解论文
溯源树
样例
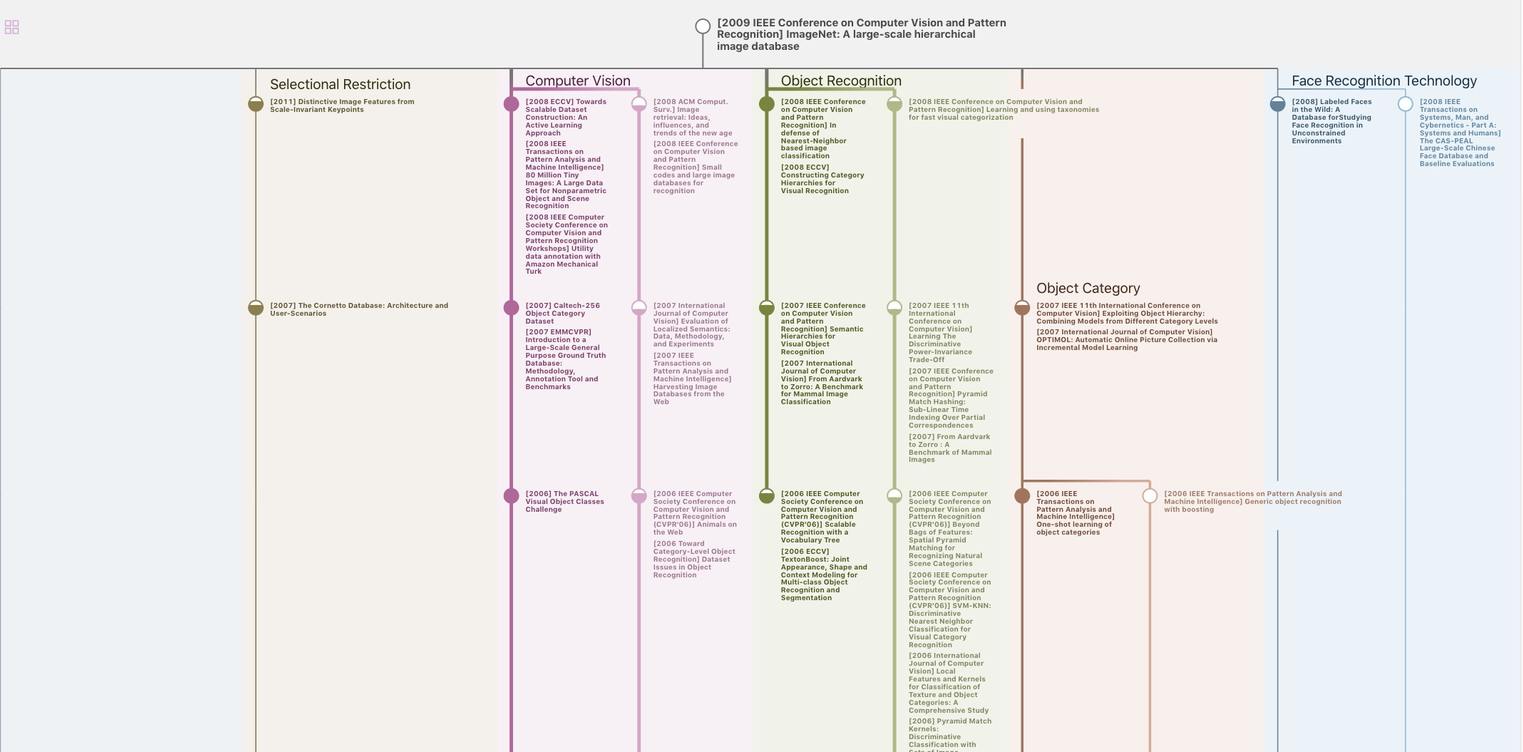
生成溯源树,研究论文发展脉络
Chat Paper
正在生成论文摘要