Genomic Prediction and Genetic Parameters of Residual Feed Intake Computed Using Linear and Non-Linear Regression Methods in Broiler Chickens
JOURNAL OF ANIMAL SCIENCE(2023)
摘要
Abstract Feed efficiency traits are important for reducing feeding costs while potentially decreasing the environmental impact of broiler production. This study aimed to compare the predictive ability of different genome-wide prediction models for residual feed intake (RFI) calculated with linear and non-linear regression techniques. We also evaluated the heritability of such RFI as well as their associations with body weight. Genotypes and phenotypes used in this study were recorded from 32,747 purebred broiler chickens of both sexes. The individual feed intake was monitored daily in floor-raised birds using a radio-frequency antenna system. RFI was computed as the residual term of total feed intake (FI) adjusted for the average metabolic weight (AMW), body weight gain (BWG), and sex. The following regression methods were used to compute RFI: multiple linear regression (MLR), generalized additive model using splines (GAM), support vector regression using a radial basis kernel (SVR), and two-hidden layer neural network (NN). The different RFI assessments were fitted jointly with body weight measured at the end of the feeding trial (FBW) using a two-trait mixed model. The effects of contemporary groups were fitted as fixed for both traits, whereas sex was also considered for FBW. RFI (computed with different regression techniques) was predicted using different genomic prediction models (BayesA, BayesB, BayesCπ, GBLUP, RKHS, and SVR). Genomic prediction accuracy (ACC) was assessed using a random forward validation scheme, in which data from previous generations were used for training the models while later generations were used to test predictions. The effect of the RFI computation method on ACC was tested using an unpaired Wilcoxon test. Sample variance observed for RFI ranged between 57,207.04 and 59,254.76, depending on the regression method used to compute it. Overall, the RFI sample variance was smaller for non-linear methods (NN, SVR, and GAM) compared with the linear model (MLR). The regression methods did not impact the genetic parameter estimates for RFI, which presented a heritability value around 0.32 ± 0.015 and genetic correlation with FBW ranging between 0.22 ± 0.04 and 0.25 ± 0.04. Prediction accuracy across genomic regression methods was slightly higher (P-value = 0.038) when RFI was computed with SVR (0.336 ± 0.023) compared with the MLR (0.329 ± 0.025), although this small difference would have little practical implications. No differences in terms of ACC were observed among the other regression methods used to compute RFI. Our preliminary results indicate that the regression technique used to compute RFI does not have major implications for this trait. Therefore, the MLR method is the preferred choice as it is simpler to implement, and it provides easy-to-interpret parameters.
更多查看译文
关键词
feed efficiency,genetic correlation,genomic selection,radio-frequency identification,non-linear methods
AI 理解论文
溯源树
样例
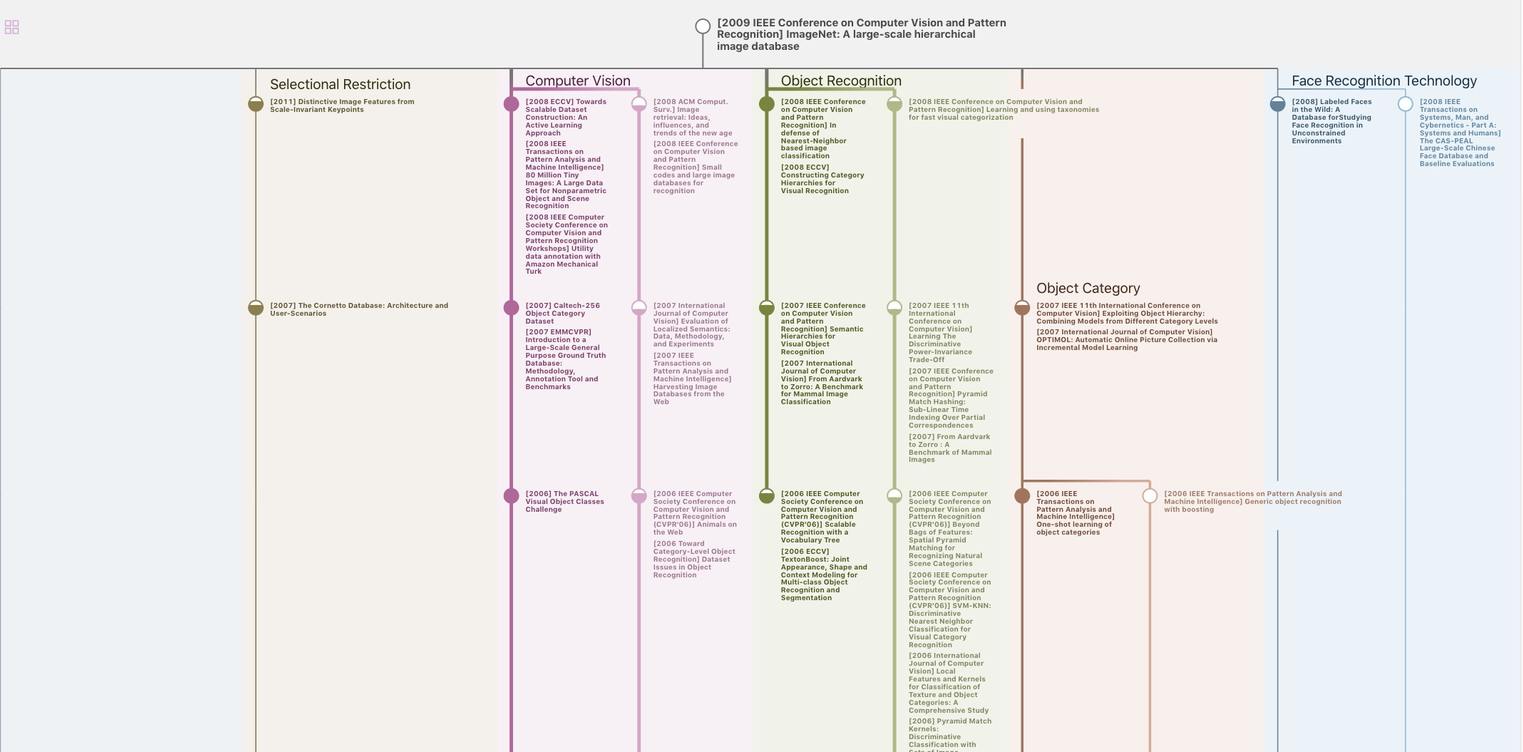
生成溯源树,研究论文发展脉络
Chat Paper
正在生成论文摘要