State Machine-Based Approach for Estimating the Capacity Loss of Lithium-Ion Batteries
Proceedings of the Annual Conference of the Prognostics and Health Management Society(2023)
摘要
The use of Lithium-Ion Batteries (LIBs) have increased in recent years in many applications such as hybrid electrical vehicles (HEV), consumer electronic equipment, and electricity grid. The batteries undergo degradation during usage due to material aging and electrochemical processes, leading to efficiency reduction of battery-powered systems as well as catastrophic events. Several stress factors such as battery temperature, ambient temperature, and C-rate in the loading profiles influence the degradation. Therefore, predicting the health of the battery has gained attention. The service life can be extended or a system failure can be avoided by maintenance measures precisely matched to the function loss or by changing usage strategies. The State-of Health (SoH) condition of the battery can be determined by the application of lifetime models. Various health indicators such as remaining useful lifetime (RuL) and capacity fade are determined by the models based on the stress factors (utilization variables). For optimal use of the battery, it is helpful to develop an accurate lifetime model to represent the dynamic properties. However, models developed are less computationally efficient and unable to represent the non-linear degradation behavior well. The development of a precise model with correct parameterization is also costly. This is particularly true for models developed based on physical and chemical properties of the battery. In this contribution, an artificial neural network (ANN)-based state machine approach is introduced for capacity fade estimation. The degradation process is represented using three states modeling three different levels and the progression from the first state to the last. Capacity associated with each state is described using the non-linear auto regressive neural network with external input (NARX). The NARX is selected due to its ability to accurately model non linear behavior and time series data. Unlike known models, which are developed using analytical mathematical equations related to the battery properties, a combined machine learning approach is used here instead to learn the capacity behavior from historical data. Battery data sets from NASA are used for experimental verification. Based on the results, the estimated capacity fade show close proximity to actual capacity fade, with a low mean square error for different data sets. In addition, the estimated state progression follows the actual state progression.
更多查看译文
关键词
capacity loss,batteries,machine-based,lithium-ion
AI 理解论文
溯源树
样例
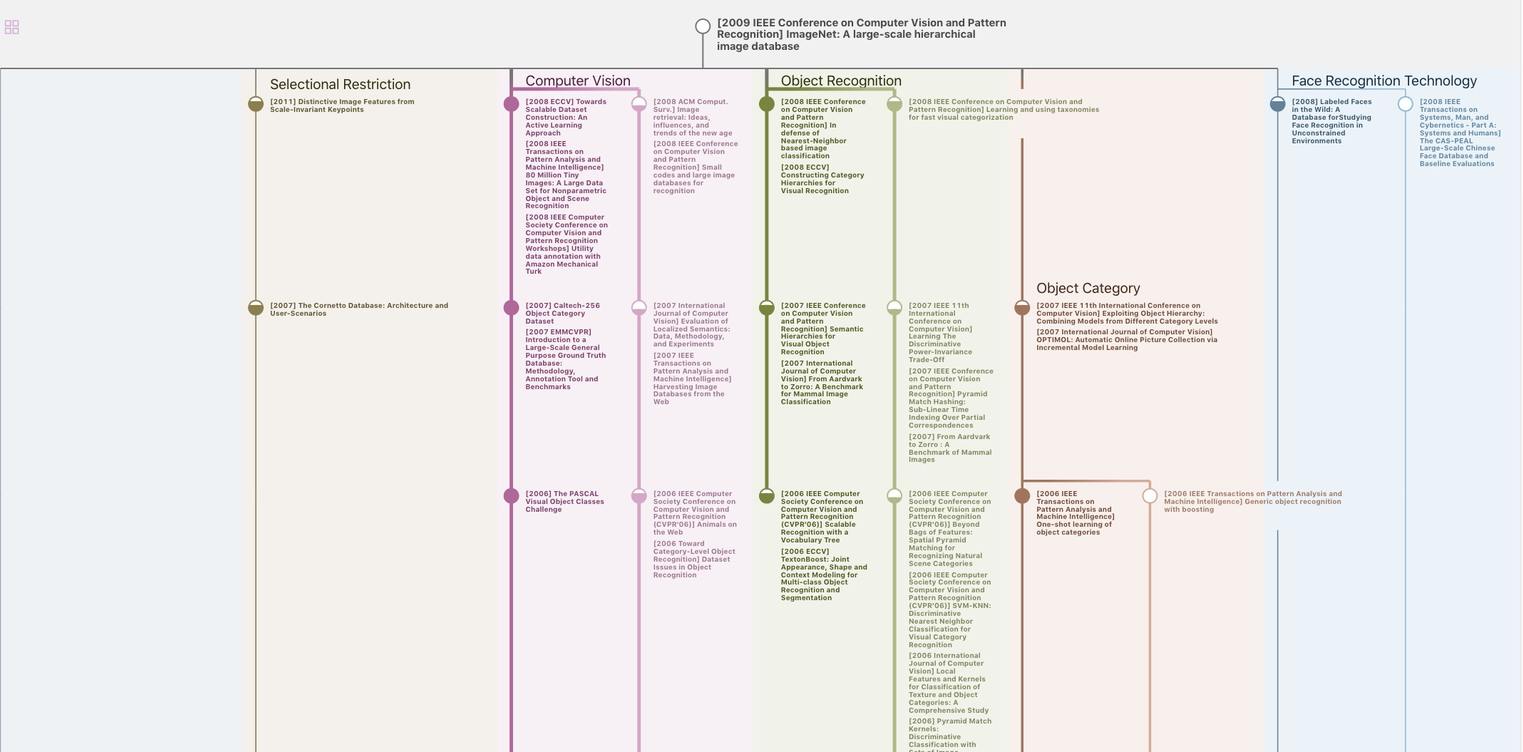
生成溯源树,研究论文发展脉络
Chat Paper
正在生成论文摘要