Nonlocal Hybrid Network for Long-tailed Image Classification
ACM TRANSACTIONS ON MULTIMEDIA COMPUTING COMMUNICATIONS AND APPLICATIONS(2024)
摘要
It is a significant issue to deal with long-tailed data when classifying images. A nonlocal hybrid network (NHN) that takes into account both feature learning and classifier learning is proposed. The NHN can capture the existence of dependencies between two locations that are far away from each other as well as alleviate the impact of long-tailed data on the model to some extent. The dependency relationship between distant pixels is obtained first through a nonlocal module to extract richer feature representations. Then, a learnable soft class center is proposed to balance the supervised contrastive loss and reduce the impact of long-tailed data on feature learning. For efficiency, a logit adjustment strategy is adopted to correct the bias caused by the different label distributions between the training and test sets and obtain a classifier that is more suitable for long-tailed data. Finally, extensive experiments are conducted on two benchmark datasets, the long-tailed CIFAR and the large-scale real-world iNaturalist 2018, both of which have imbalanced label distributions. The experimental results show that the proposed NHN model is efficient and promising.
更多查看译文
关键词
Nonlocal module,balanced contrastive loss,logits adjustment,long-tailed classification
AI 理解论文
溯源树
样例
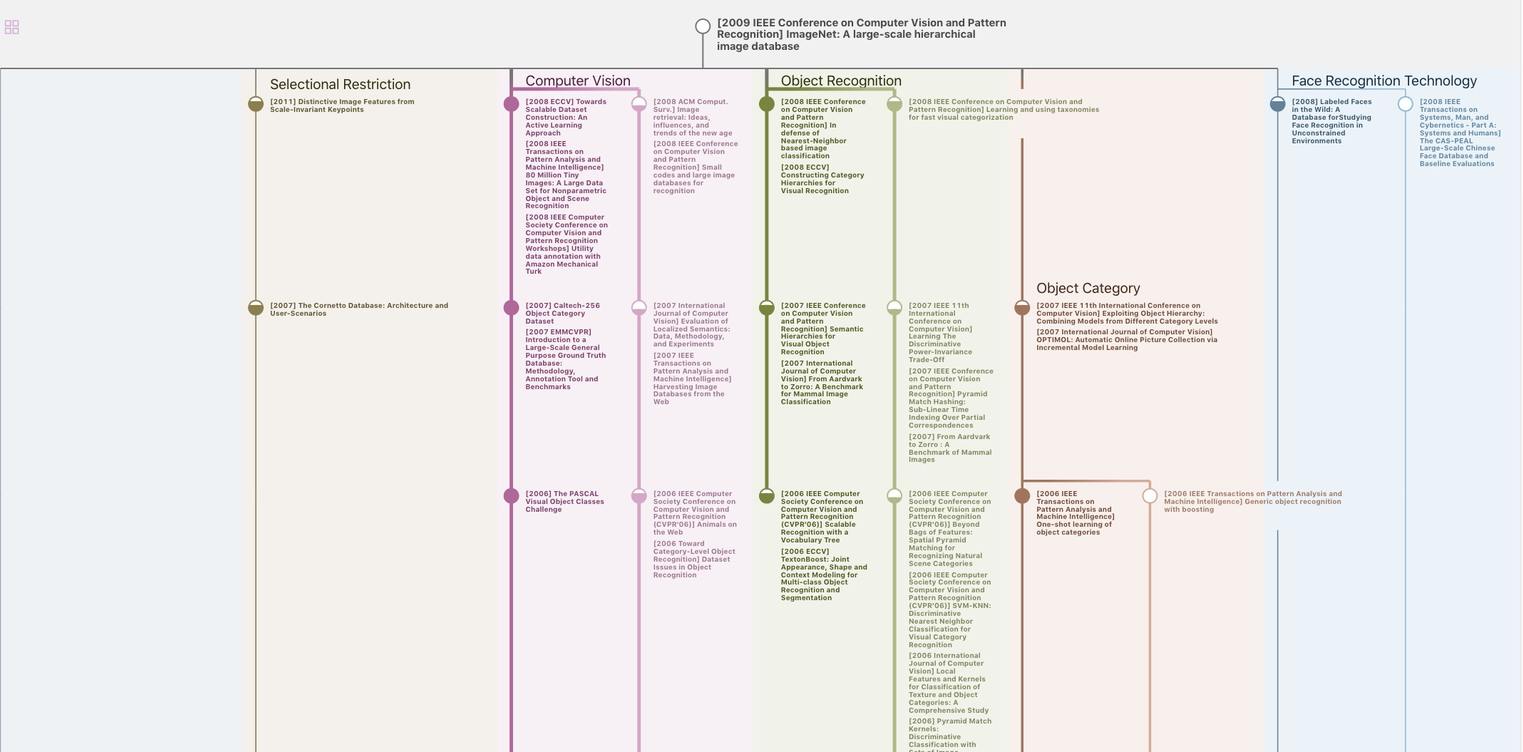
生成溯源树,研究论文发展脉络
Chat Paper
正在生成论文摘要