Enhancing Asynchronous Time Series Forecasting with Contrastive Relational Inference
2023 23RD IEEE INTERNATIONAL CONFERENCE ON DATA MINING WORKSHOPS, ICDMW 2023(2023)
摘要
Asynchronous time series, also known as temporal event sequences, are the basis of many applications throughout different industries. Temporal point processes(TPPs) are the standard method for modeling such data. Existing TPP models have focused on parameterizing the conditional distribution of future events instead of explicitly modeling event interactions, imposing challenges for event predictions. In this paper, we propose a novel approach that leverages Neural Relational Inference (NRI) to learn a relation graph that infers interactions while simultaneously learning the dynamics patterns from observational data. Our approach, the Contrastive Relational Inference-based Hawkes Process (CRIHP), reasons about event interactions under a variational inference framework. It utilizes intensity-based learning to search for prototype paths to contrast relationship constraints. Extensive experiments on three realworld datasets demonstrate the effectiveness of our model in capturing event interactions for event sequence modeling tasks. Code will be integrated into the EasyTPP* framework.
更多查看译文
关键词
asynchronous time series forecasting,relational inference,contrastive
AI 理解论文
溯源树
样例
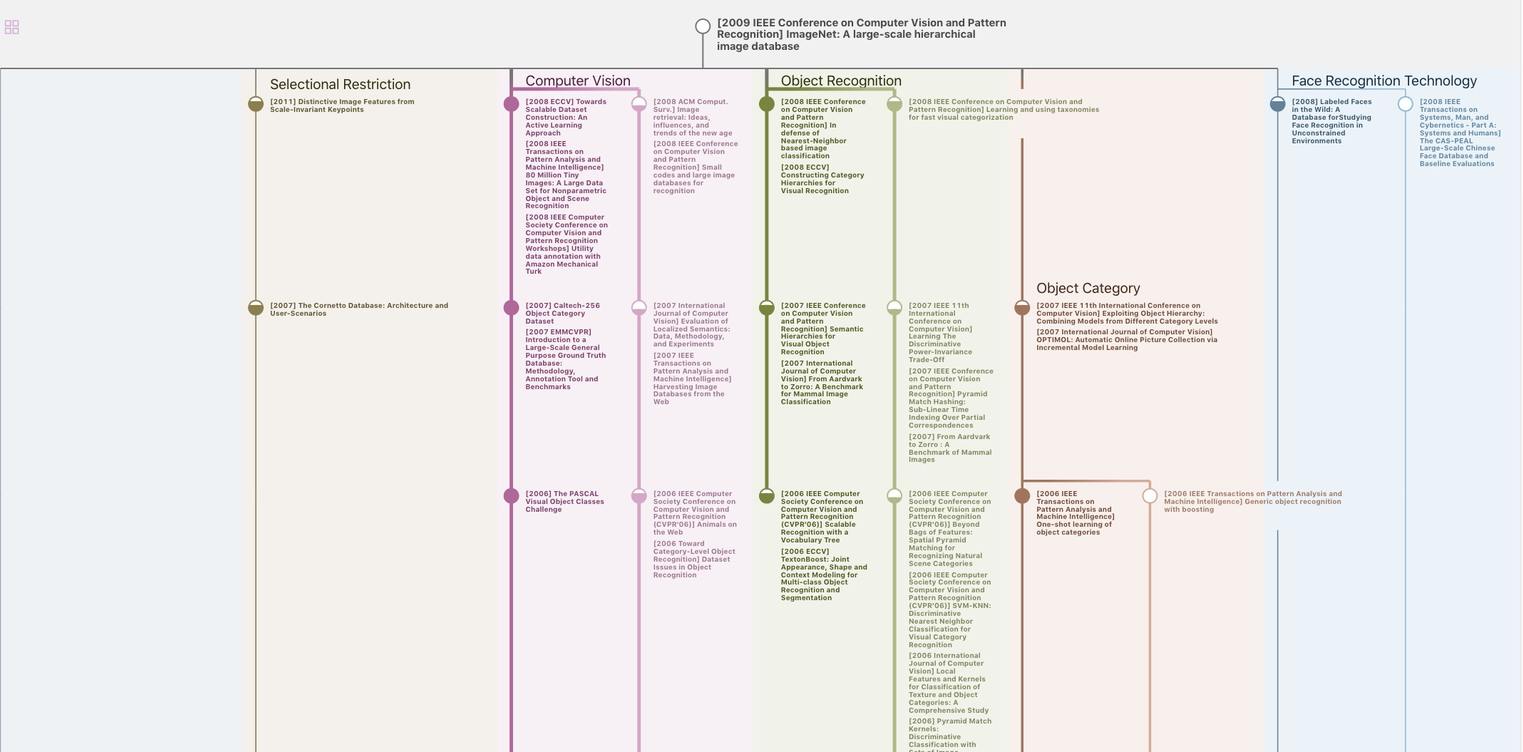
生成溯源树,研究论文发展脉络
Chat Paper
正在生成论文摘要