P703: the aipss-mds machine learning model predicts overall survival and leukemic transformation in cmml: an analysis of the spanish registry of mds
HemaSphere(2023)
摘要
Background: Chronic myelomonocytic leukemia (CMML) is a type of hematological stem cell disorder characterized by dysplasia, myeloid proliferation, cytopenias and increased risk of acute leukemia. CMML evolution is greatly heterogeneous, and treatment options vary from observation to allogeneic stem cell transplantation. Therefore, a precise disease risk prognostication is critical for treatment decision making. Recently, we developed a machine learning model (Artificial Intelligence Prognostic Scoring System for MDS; AIPSS-MDS) to risk stratify MDS patients based on 8 simple variables: age, sex, hemoglobin, platelet counts, leukocyte counts, proportion of neutrophils in peripheral blood, proportion of blast cells in bone marrow and cytogenetic risk group. This model was superior than the revised International Prognostic Score System (IPSS-R) score in Spanish patients, achieving higher c-indexes for the prediction of overall survival (OS) and leukemia-free survival (LFS). Aims: In the present report, we aimed to evaluate the performance of the AIPSS-MDS prognostic system in patients diagnosed with CMML. Methods: Registry data from a total of 1,343 CMML patients in 90 Spanish institutions were retrieved. A cohort of 75 patients from Taiwan that was also evaluated and used for comparison with the CPSS and CPSS-Mol scores. The AIPSS-MDS random forests model was used for prediction of the cumulative hazard function and the survival function. Parameter imputation was performed on missing data using a random forests algorithm developed by Ishawarian et al (2008). Then we used cox regression to calculate the bootstrapped areas under the curve (AUCs) of the AIPSS-MDS predictors and the IPSS-R score. Bootstrapping was performed with 500 cycles. The c-indexes were computed on the basis of the predicted cumulative hazard function. Results: The AIPSS-MDS algorithm achieved a c-index for OS prediction of 0.712, and a c-index for LFS of 0.767 in the Spanish cohort. Time-dependent AUCs for the AIPSS-MDS and the IPSS-R confirmed the superiority of the AIPSS-MDS system compared with the IPSS-R score at all the evaluated time points. This difference was particularly notorious in the case of the OS prediction. For example, the AIPSS-MDS and the IPSS-R score obtained a 5-year AUC of 78.65% and 66.79% for OS prediction, respectively (Figure 1 A). In the case of LFS, the difference in 5-year AUCs was also favourable to the machine learning approach: 76.36% and 72.34% for the AIPSS-MDS and IPSS-R score, respectively (Figure 1 B). Finally, we evaluated the performance of the AIPSS-MDS score in a cohort of 75 patients from Taiwan. In this cohort, the c-index of the AIPSS-MDS for OS was 0.694, which was superior to those of the IPSS (0.608), CPSS (0.657) and CPSS-Mol (0.629). In the case of the LFS prediction, the AIPSS-MDS achieved a c-index of 0.726, which was also superior to those of the IPSS (0.597), CPSS (0.576) and CPSS-Mol (0.566). Summary/Conclusion: The AIPSS-MDS is a machine learning model based exclusively on clinical and cytogenetic variables that are readily available in any healthcare facility. This simple model has been trained on data from patients diagnosed with MDS, and it can also be used to risk stratify patients with CMML, outperforming the accuracy of other well-established prognostic scores. An interactive web calculator of this model can be accessed in the following link: https://www.gesmd.es/aipss-mds/Keywords: CMML, MDS, Acute leukemia, Prognosis
更多查看译文
关键词
leukemic transformation,overall survival,cmml,aipss-mds
AI 理解论文
溯源树
样例
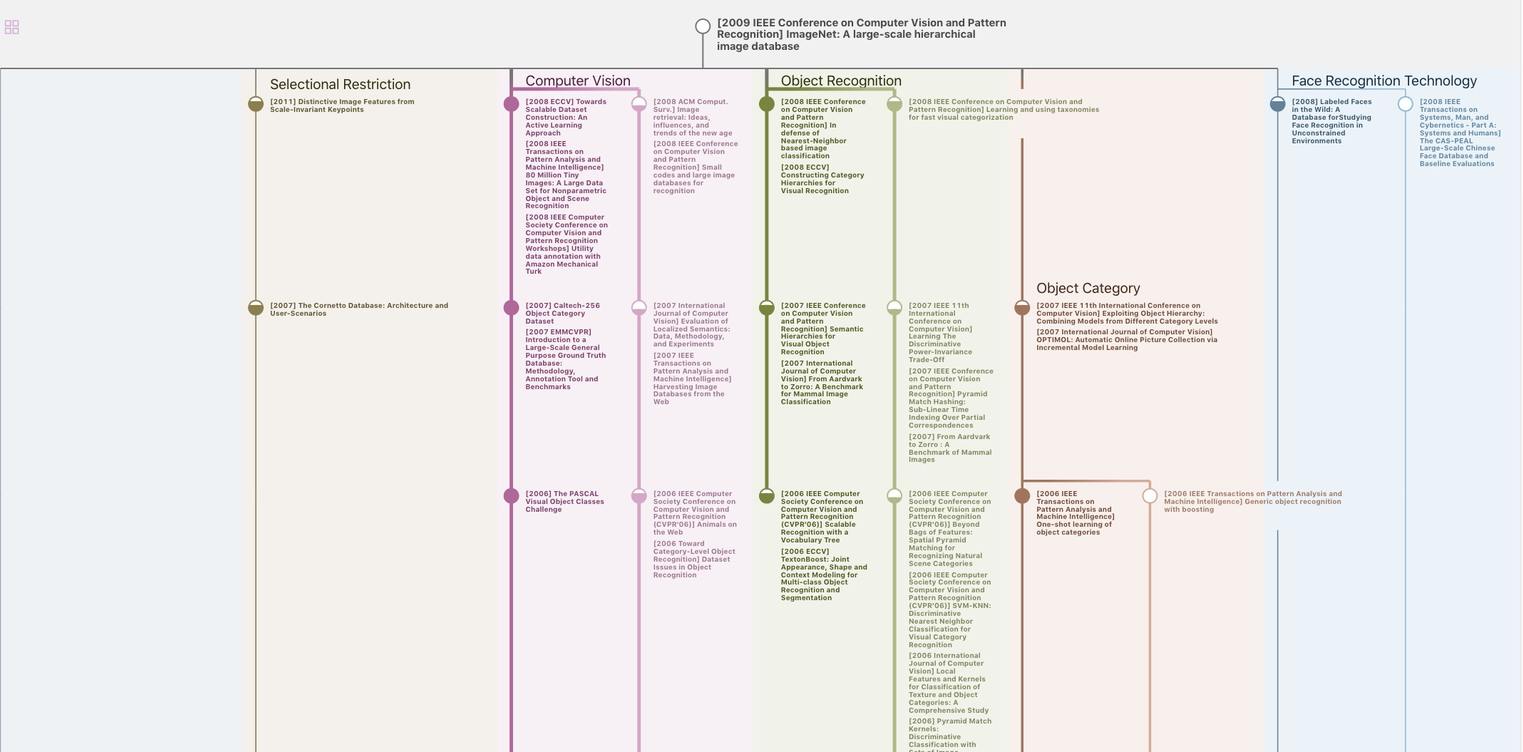
生成溯源树,研究论文发展脉络
Chat Paper
正在生成论文摘要