Multi-Granularity Interaction for Multi-Person 3D Motion Prediction
IEEE TRANSACTIONS ON CIRCUITS AND SYSTEMS FOR VIDEO TECHNOLOGY(2024)
摘要
Multi-person 3D motion prediction is an emerging task that involves predicting the future 3D motion of multiple individuals based on current observations. In contrast to motion prediction for a single person, this task requires a strong emphasis on learning the interacting dynamics among multiple individuals. Broadly speaking, current methods can be categorized into two groups: The first group involves the straightforward adaptation of models originally developed for single-person scenarios to multi-person scenarios, which is evidently suboptimal. The second group focuses on utilizing off-the-shelf tools like graph convolutional networks to model interactions. While this approach has shown improved results, the interactions primarily consider entire human identities rather than finer details. This motivates the introduction of our novel solution to address this limitation and enhance the task's performance. In this work, we strive to craft a novel framework that can effectively address two key issues ignored in previous works, namely the multi-granularity interaction and time-varying inter-person dynamics. In implementation in accord with above aims, the proposed model has mainly comprised two modules: a person-level interaction module and a part-level interaction module. The former is designed to learn the holistic and dynamic interaction among multiple persons in a coarse-grained sense. Critically, we would emphasize that a unique trait of the former module is learning temporal dynamics. For example, it recognizes that two individuals exhibit a strong correlation during handshaking but less correlation after parting ways. The latter part-level interaction module learns the interaction between the body joints of different persons. This module operates at a more fine-grained level, distinguishing it from existing approaches. By aggregating information from both granularities, our model enables accurate motion prediction. To validate the effectiveness of the proposed model, we conducted comprehensive experiments on three benchmark datasets: 3DPW, CMU-Mocap, and MuPoTS-3D. The results of these evaluations unequivocally demonstrate the empirical superiority of our model compared to previous state-of-the-art methods.
更多查看译文
关键词
Human 3D motion prediction,neural networks,multi-granularity interaction
AI 理解论文
溯源树
样例
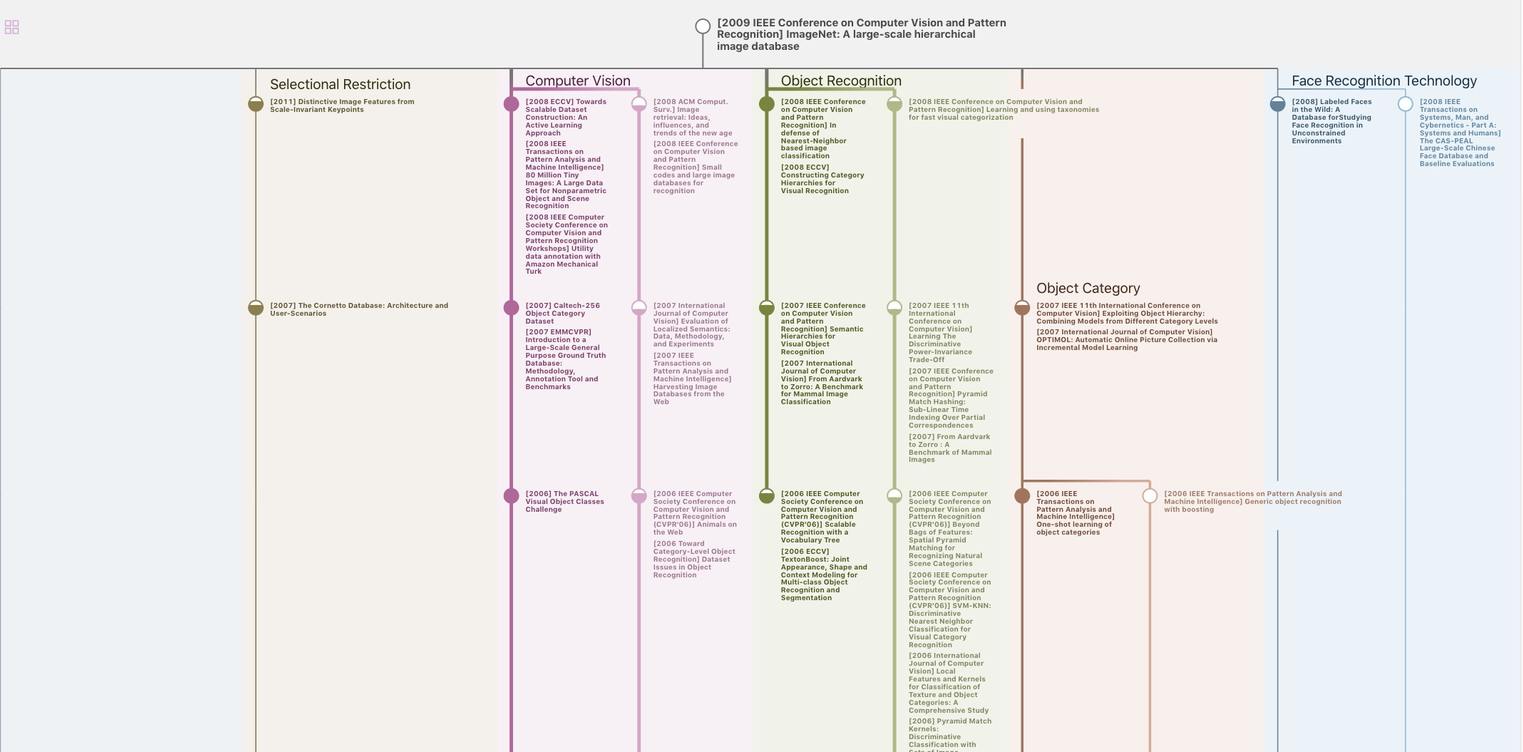
生成溯源树,研究论文发展脉络
Chat Paper
正在生成论文摘要