Multi-Level Transitional Contrast Learning for Personalized Image Aesthetics Assessment
IEEE TRANSACTIONS ON MULTIMEDIA(2024)
摘要
Personalized image aesthetics assessment (PIAA) is aimed at modeling the unique aesthetic preferences of individuals, based on which personalized aesthetic scores are predicted. People have different standards for image aesthetics, and accordingly, images rated at the same aesthetic level by different users explicitly reveal their aesthetic preferences. However, previous PIAA models treat each individual as an isolated optimization target, failing to take full advantage of the contrastive information among users. Further, although people's aesthetic preferences are unique, they still share some commonalities, meaning that PIAA models could be built on the basis of generic aesthetics. Motivated by the above facts, this article presents a Multi-level Transitional Contrast Learning (MTCL) framework for PIAA by transiting features from generic aesthetics to personalized aesthetics via contrastive learning. First, a generic image aesthetics assessment network is pre-trained to learn the common aesthetic features. Then, image sets rated to have the same aesthetic levels by different users are employed to learn the differentiated aesthetic features through multiple level-wise contrast learning based on the generic aesthetic features. Finally, a target user's PIAA model is built by integrating generic and differentiated aesthetic features. Extensive experiments on four benchmark PIAA databases demonstrate that the proposed MTCL model outperforms the state-of-the-arts.
更多查看译文
关键词
Personalized image aesthetics assessment,contrast learning,aesthetic preferences
AI 理解论文
溯源树
样例
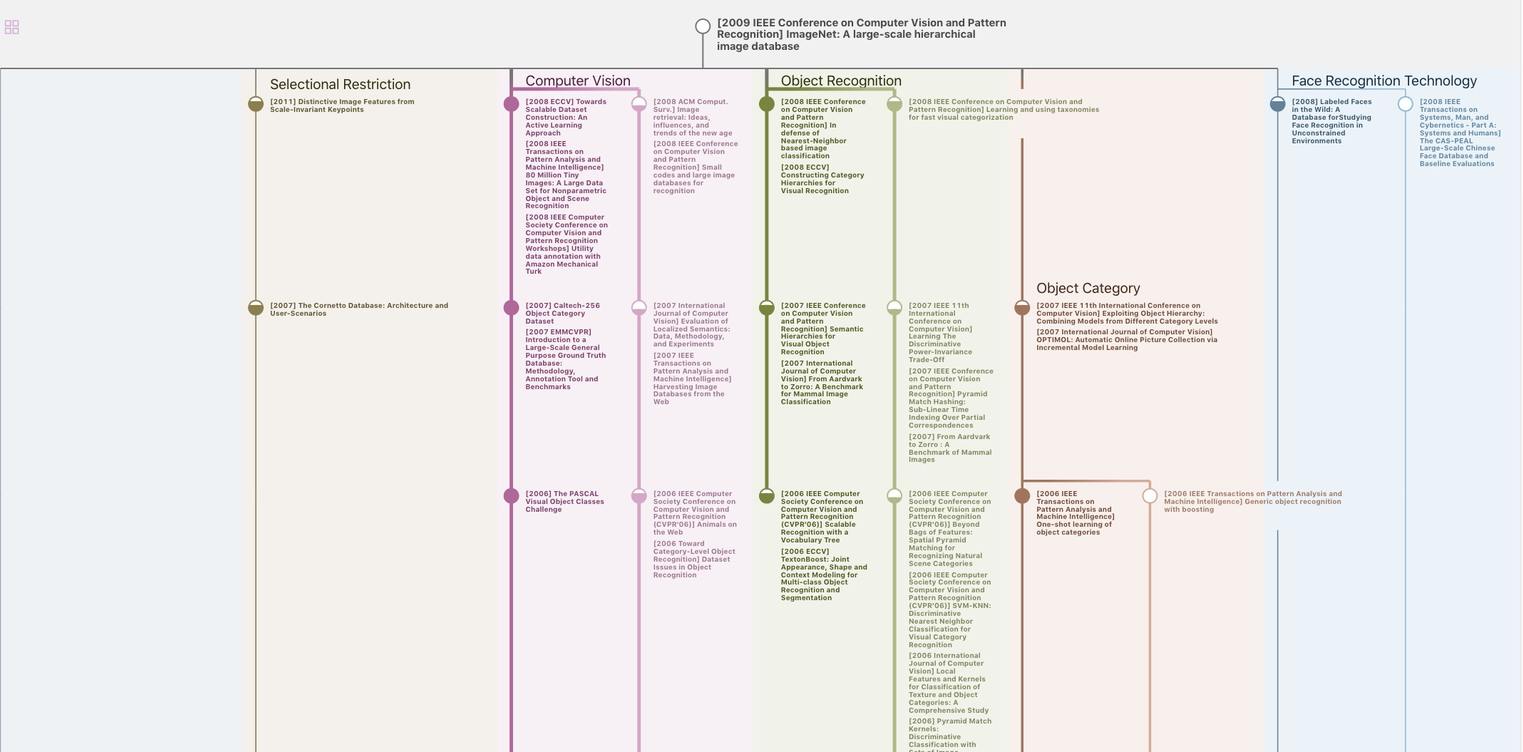
生成溯源树,研究论文发展脉络
Chat Paper
正在生成论文摘要