Intelligent Event-Triggered Control Supervised by Mini-Batch Machine Learning and Data Compression Mechanism for T-S Fuzzy NCSs Under DoS Attacks
IEEE TRANSACTIONS ON FUZZY SYSTEMS(2024)
摘要
This article presents a comprehensive solution to mitigate network congestion in T-S fuzzy networked control systems caused by denial-of-service (DoS) attacks and quality-of-service (QoS) queuing mechanisms. We develop a novel data compression mechanism to alleviate network congestion and use a mini-batch descent gradient algorithm to optimize trigger thresholds, thereby reducing bandwidth usage. In addition, we introduce asymmetric Lyapunov-Krasovskii functions to decrease the number of decision variables, which improves the reliability and robustness of the control algorithm. Finally, we propose an intelligent event-triggered controller supervised by mini-batch machine learning and validate it on the joint CarSim-Simulink platform. Experimental results demonstrate that our approach reduces the sensitivity of autonomous vehicle systems to network fluctuations while ensuring system stability under network congestion caused by DoS attacks.
更多查看译文
关键词
Reliability,Stability criteria,Security,Protocols,Delays,Asymptotic stability,Sun,Cyber security,event-triggered control,Lyapunov-Krasovskii function (LKF),machine learning,T-S fuzzy networked control system
AI 理解论文
溯源树
样例
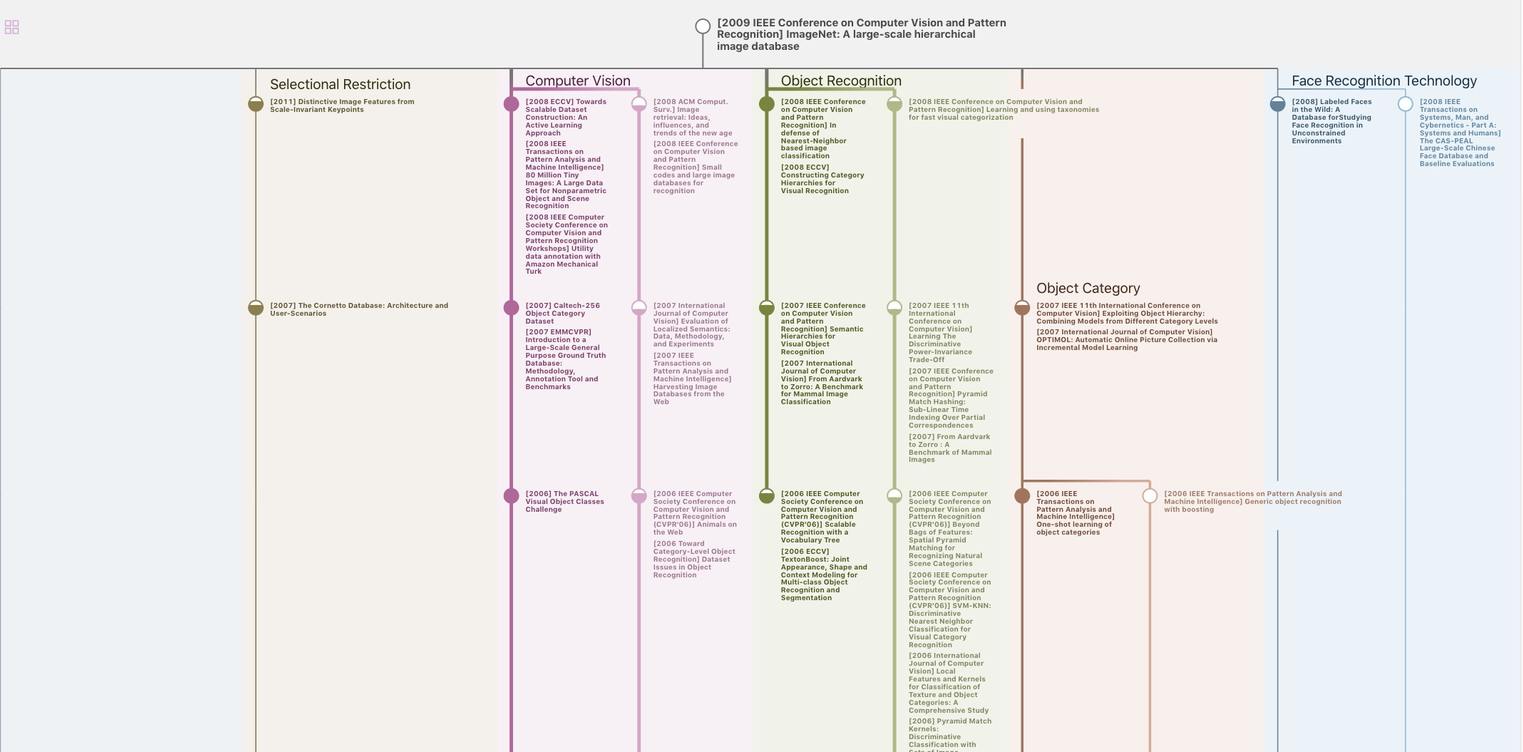
生成溯源树,研究论文发展脉络
Chat Paper
正在生成论文摘要