Using Computer Vision To Automate Direct Observation Of Physical Behavior
MEDICINE & SCIENCE IN SPORTS & EXERCISE(2023)
摘要
PURPOSE: Direct observation is a widely accepted ground-truth measure for multiple aspects of physical behavior, but the high cost to collect and annotate data makes it cost prohibitive for many studies. The purpose of this study was to develop and test machine learning methods to recognize aspects of physical behavior and location from still images extracted from videos of human movement. METHODS: Twenty-five adults (age 18-57y, 61.6% female) were video-recorded in their natural environment for two, 2-hour sessions. Videos were annotated by trained research assistants using commercially available software. For this analysis, the taxonomies included are 1) sedentary vs not sedentary; 2) activity intensity (sedentary, light, moderate, vigorous); 3) location (indoor, outdoor, driving); 4) detailed activity type (i.e., posture/movement, 6 categories); 5) detailed location (10 categories). The inter-rater reliability (ICC = 0.97) was excellent in a random sample of 10% of videos that were dual-coded. Multiple machine learning models were trained and evaluated for each taxonomy including the use of convolutional neural networks pre-trained on large open-source image dataset for analysis of raw pixel image data, skeleton modeling using alphaPose models, and a variety of neural network architectures combining these approaches. Models were trained on 80% of the videos, validated on 10%, with the final accuracy (% frames on which the model recognized the correct taxonomy label) reported on the remaining 10% of the videos that were unseen in training. RESULTS: Validation accuracy was 98.5% for Sedentary/Non-sedentary, 93% for activity intensity, 95% for basic (3-class) location, 89% for activity type and 71% for the 10-class activity location. CONCLUSIONS: This proof-of-concept study shows it is possible to use computer vision to annotate aspects of physical behavior, dramatically speeding up the time and reducing labor required for direct observation. Future research should test these machine learning models in larger, independent datasets and take advantage of analysis of video fragments, rather than individual still images. Supported by NIH/NCI contract #75N91021C00040
更多查看译文
AI 理解论文
溯源树
样例
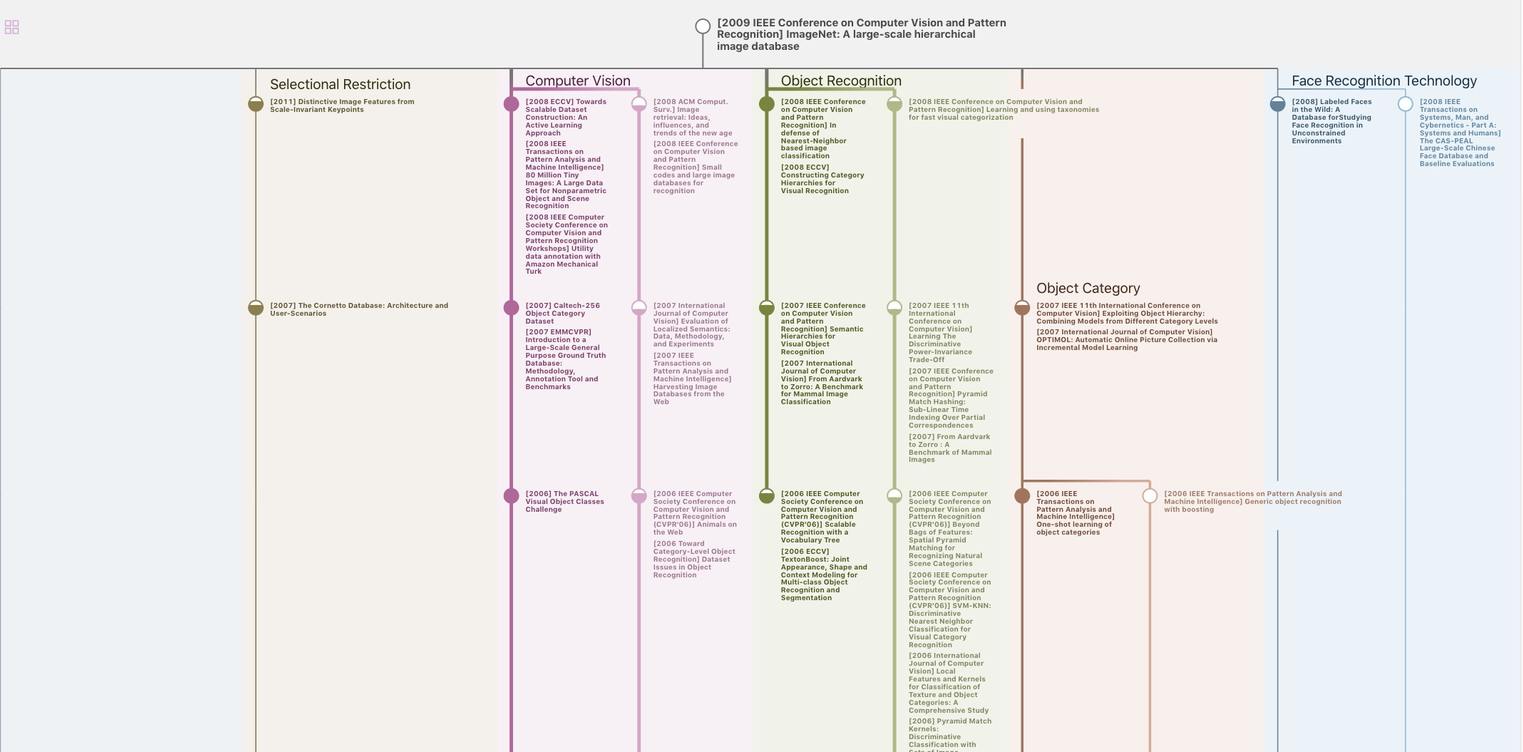
生成溯源树,研究论文发展脉络
Chat Paper
正在生成论文摘要