Enhancing the shear-stress-transport turbulence model with symbolic regression: A generalizable and interpretable data-driven approach
Physical review fluids(2023)
摘要
Data-driven Reynolds averaged Navier-Stokes (RANS) turbulence models for separated flows based on black-box machine learning models have been widely researched in recent years. However, they often lack generalizability and interpretability. In this work, field inversion and symbolic regression (FISR) are used to develop an interpretable and generalizable data-driven RANS turbulence model. The proposed turbulence model shows good accuracy in various test cases that are completely distinct from its training set.
更多查看译文
关键词
symbolic regression,turbulence,shear-stress-transport,data-driven
AI 理解论文
溯源树
样例
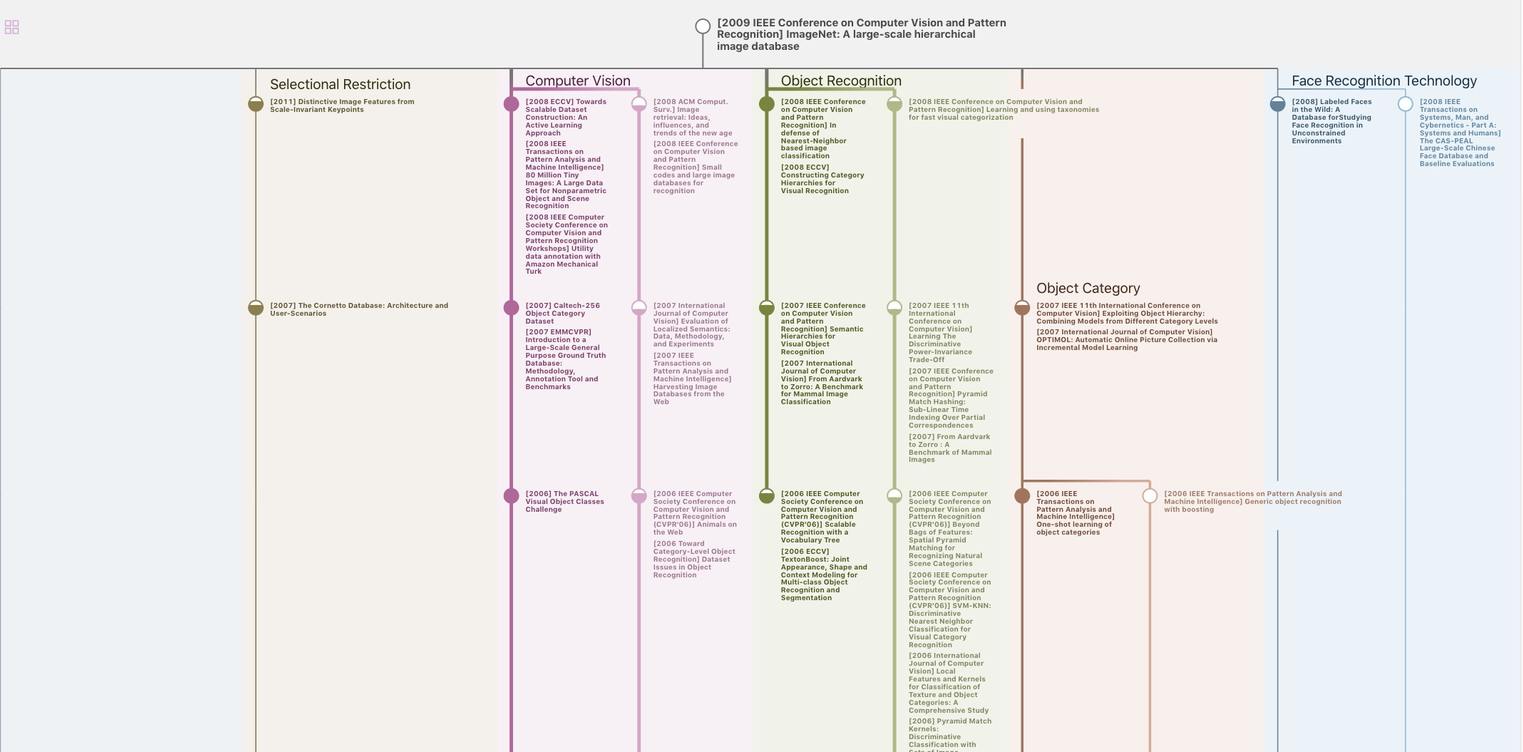
生成溯源树,研究论文发展脉络
Chat Paper
正在生成论文摘要