DMH-CL: Dynamic Model Hardness Based Curriculum Learning for Complex Pose Estimation
IEEE TRANSACTIONS ON MULTIMEDIA(2024)
摘要
When dealing with crowds, occlusions, and truncations in complex scenes, existing solutions for multi-person pose estimation remain challenging. This is because all examples are randomly organized and equally treated by previous methods during training, which ignores that examples vary significantly in their difficulty levels. Once trained, hard examples are underutilized due to the high proportion of simple training examples, resulting in poor model robustness for complex scenes. To tackle this, we propose a novel training strategy termed DMH-CL for complex pose estimation, brought from curriculum learning (CL) which mainly addresses easy examples in the early training stage and hard ones in the later stage. Different from typical CL methods, we define easy/hard examples via mining both the dataset-specific statistical difficulty and the multi-model evaluated difficulty. After that, we adopt an annealing arrangement strategy to construct learning courses from easy to hard. Furthermore, we introduce a model learning feedback indicator, i.e., Dynamic Model Hardness (DMH) to conduct course scheduling, and to explicitly explore hard poses and utilize the knowledge learned from easy poses to better handle complex scenes as well. Our DMH-CL is model-agnostic and can be easily applied to various pose estimators including single-stage models and two-stage models, and achieves significant improvements on two challenging benchmarks especially for complex scenes. Notably, it achieves substantial performance gains of 2.6% and 4.6% for hard poses compared to the strong single-stage model PETR on CrowdPose and COCO datasets, respectively. Source codes and models are publicly available online.
更多查看译文
关键词
Training,Pose estimation,Computational modeling,Dynamic scheduling,Task analysis,Robustness,Training data,Multi-person Pose Estimation,Dynamic Model Hardness,Curriculum Learning
AI 理解论文
溯源树
样例
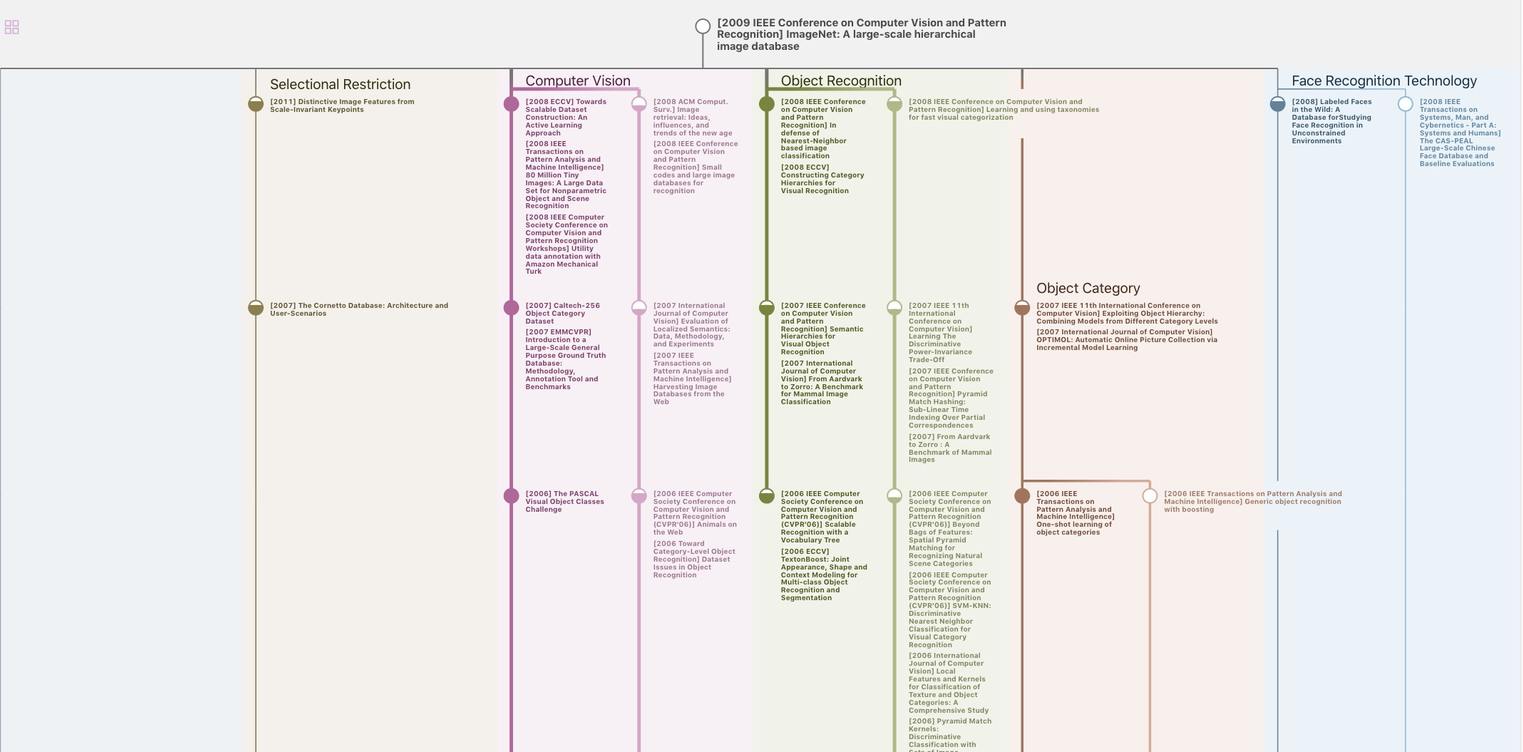
生成溯源树,研究论文发展脉络
Chat Paper
正在生成论文摘要