RandMixAugment: A Novel Unified Technique for Region- and Image-Level Data Augmentations
IEEE ACCESS(2024)
摘要
Deep learning models learn powerful representational spaces required for handling complex tasks. Recently, data augmentation techniques, region-level, and image-level augmentation have proved effective in significantly improving deep learning models' generalization performance. Nevertheless, such methods may lose some critical features or are still computationally heavy (or inefficient) due to additional computation burdens. To address this issue, in this paper, we present a novel unified data augmentation method for deep learning models, namely, RandMixAugment, which effectively combines the intrinsic properties of region-level augmentation and image-level augmentation. Specifically, the proposed RandMixAugment employs automated augmentation with masking and mixing operations. Experiments are conducted on well-known CIFAR datasets (CIFAR-10 and CIFAR-100) to verify the effectiveness of the proposed scheme compared to state-of-the-art augmentation techniques. The experimental results demonstrate that the proposed RandMixAugment yields superior performance over state-of-the-art techniques on image classification tasks and further improves the performance of the baseline deep learning model by 1.2% and 2.4% on CIFAR-10 and CIFAR-100 datasets, respectively.
更多查看译文
关键词
Classification,data augmentation,deep learning,image processing,supervised learning
AI 理解论文
溯源树
样例
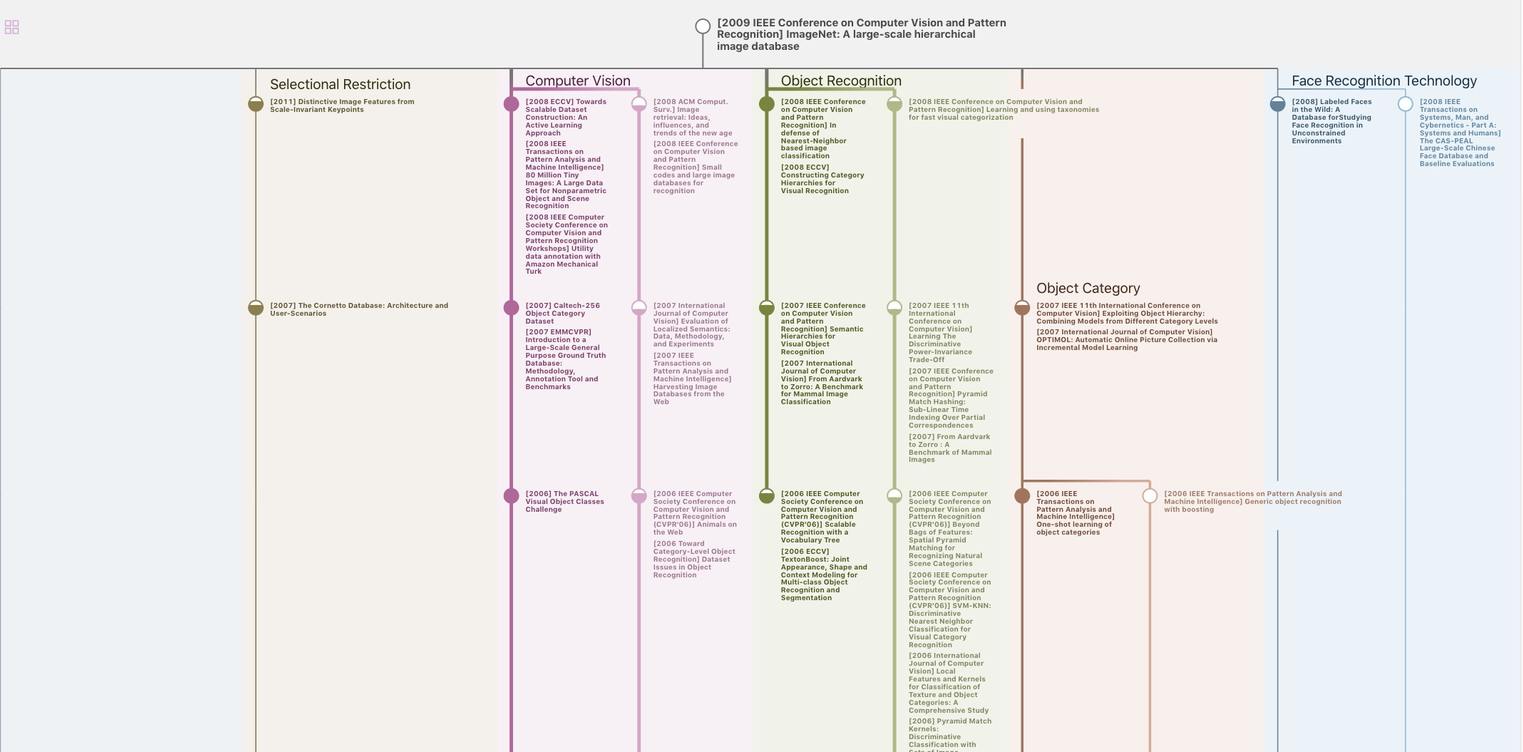
生成溯源树,研究论文发展脉络
Chat Paper
正在生成论文摘要