Noninvasive Cuffless Blood Pressure Monitoring. How Mechanism-Driven and Data-Driven Models Can Help in Clinical Practice
Journal of cardiology and cardiovascular medicine(2023)
摘要
Continuous noninvasive cuffless blood pressure (BP) monitoring is essential for early detection and treatment of hypertension. In this paper, we provide an overview of the recent advancements in cuffless BP sensors. These include contact wearable sensors such as electrocardiography (ECG), photoplethysmography (PPG), contact non-wearable sensors such as ballistocardiography (BCG), and contactless sensors such as video plethysmography (VPG). These sensors employ different measuring mechanisms such as pulse arrival time (PAT), pulse transit time (PTT), and pulse wave analysis (PWA) to estimate BP. However, challenges exist in the effective use and interpretation of signal features to obtain clinically reliable BP measurements. The correlations between signal features and BP are obtained by mechanism-driven models which use physiological principles to identify mathematical correlations, and data-driven models which use machine learning algorithms to analyze observational data to identify multidimensional correlations. On the one hand, applying mechanism-driven models to non-linear scenarios and incomplete or noisy data is challenging On the other hand, data-driven models require a large amount of data in order to prevent physically inconsistent predictions, resulting in poor generalization. From this perspective, this paper proposes to combine the strengths of mechanism-driven and data-driven approaches to obtain a more comprehensive approach, the physiology-informed machine-learning approach, with the goal of enhancing the accuracy, interpretability, and scalability of continuous cuffless BP monitoring. This holds promise for personalized clinical applications and the advancement of hypertension management.
更多查看译文
关键词
blood pressure,mechanism-driven,data-driven
AI 理解论文
溯源树
样例
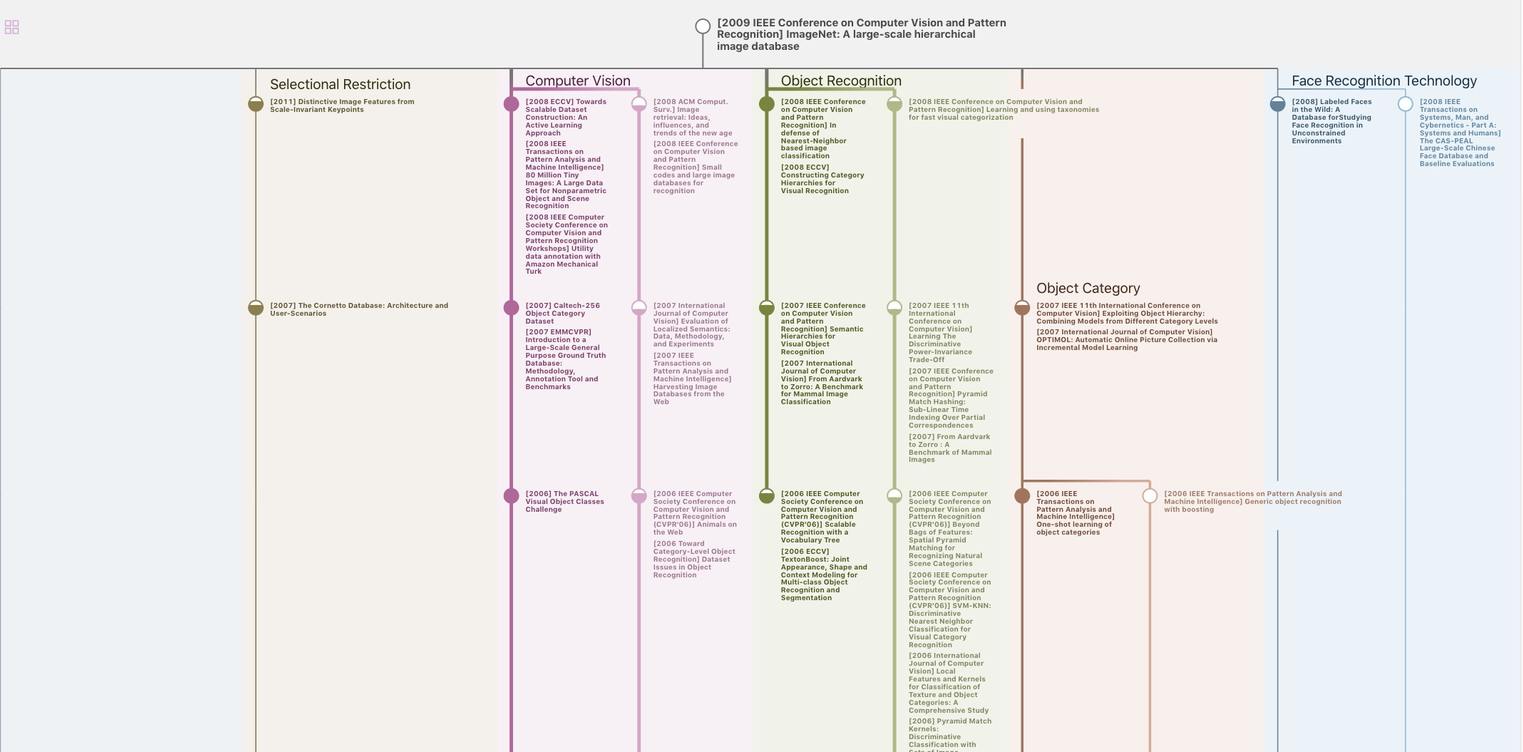
生成溯源树,研究论文发展脉络
Chat Paper
正在生成论文摘要