A Tutorial on Bayesian Modeling of Change Across Time, Individuals, and Groups
Computational Brain & Behavior(2023)
摘要
Abstract Psychological theories often incorporate dynamic processes, but it can be difficult to accurately represent these processes with standard statistical tests. As such, there tends to be a misalignment between theory and statistical analysis. We provide a tutorial on a flexible Bayesian approach to developing and analyzing discrete dynamic models that overcomes many challenges associated with conventional methods. This approach can be used to analyze models of virtually any functional form, including models with feedback loops and dynamic (i.e., stock or level) variables. It allows one to quantify uncertainty in components of a dynamic process. This approach also provides a natural way to examine variation in a process between individuals, known groups, or latent subgroups. This framework has the flexibility to capture the dynamism inherent in many theories, which we believe will facilitate theory testing, and ultimately, cumulative theoretical progress.
更多查看译文
关键词
bayesian modeling,individuals,groups,change
AI 理解论文
溯源树
样例
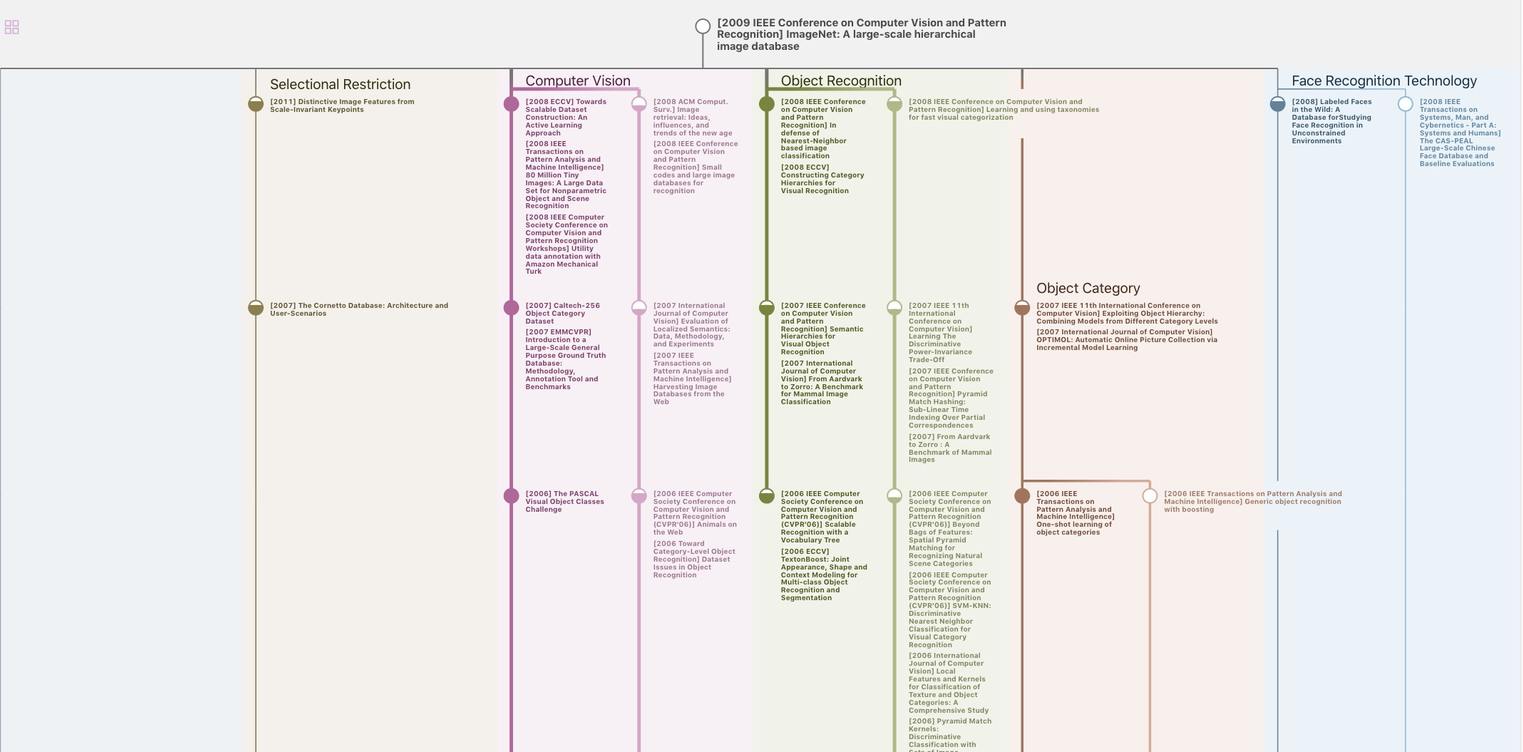
生成溯源树,研究论文发展脉络
Chat Paper
正在生成论文摘要