Embedding-Space Explanations of Learned Mixture Behavior
Springer eBooks(2023)
摘要
Abstract Data-driven machine learning (ML) models are attracting increasing interest in chemical engineering and already partly outperform traditional physical simulations. Previous work in this field has mainly focused on improving the models’ statistical performance while the thereby imparted knowledge has been taken for granted. However, also the structures learned by the model during the training are fascinating yet non-trivial to assess as they are usually high-dimensional. As such, the interpretable communication of the relationship between the learned model and domain knowledge is vital for its evaluation by applying engineers. Specifically, visual analytics enables the interactive exploration of data sets and can thus reveal structures in otherwise too large-scale or too complex data. This chapter focuses on the thermodynamic modeling of mixtures of substances using the so-called activity coefficients as exemplary measures. We present and apply two visualization techniques that enable analyzing high-dimensional learned substance descriptors compared to chemical domain knowledge. We found explanations regarding chemical classes for most of the learned descriptor structures and striking correlations with physicochemical properties.
更多查看译文
关键词
mixture,behavior,embedding-space
AI 理解论文
溯源树
样例
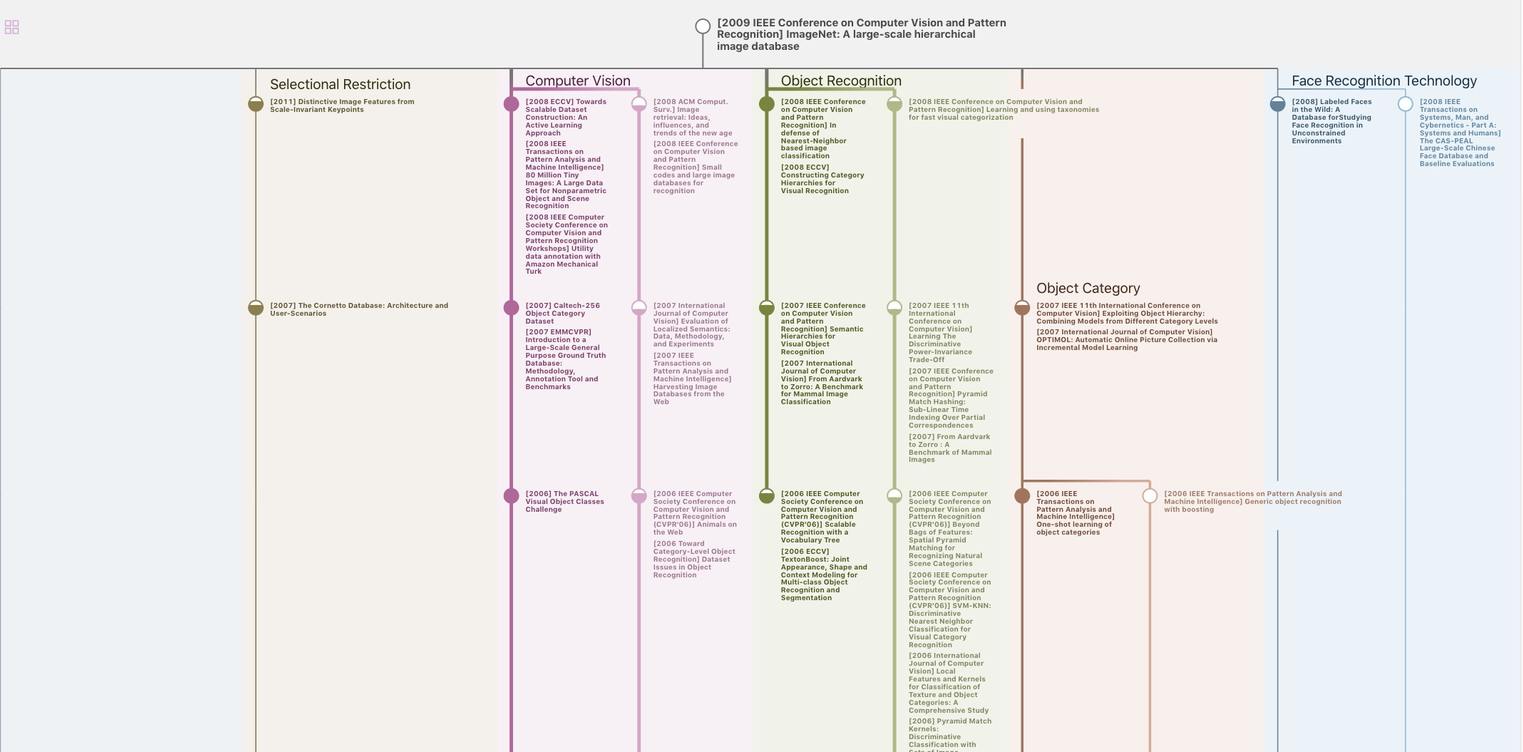
生成溯源树,研究论文发展脉络
Chat Paper
正在生成论文摘要