Constructing neural networks with pre-specified dynamics
bioRxiv (Cold Spring Harbor Laboratory)(2023)
摘要
Abstract A main goal in neuroscience is to understand the computations carried out by neural populations that give animals their cognitive skills. Neural network models allow to formulate explicit hypothesis regarding the algorithms instantiated in the dynamic of a neural population, its firing statistics, and the underlying connectivity. Neural networks can be defined by a small set of parameters, carefully chosen to procure specific capabilities, or by a large set of free parameters, fitted with optimization algorithms that minimize a given loss function. In this work we alternatively propose a method to make a detailed adjustment of the network dynamic and firing statistic to better answer questions that link dynamic, structure and function. Our algorithm – termed generalized Firing-to-Parameter (gFTP) – provides a way to construct binary recurrent neural networks whose dynamic strictly follows a user pre-specified transition graph that details the transitions between population firing states triggered by stimulus presentations. Our main contribution is a procedure that detects when a transition graph is not realizable in terms of a neural network, and makes the necessary modifications in order to obtain a new transition graph that is realizable and preserves all the information encoded in the transitions of the original graph. With a realizable transition graph, gFTP assigns values to the network firing states associated with each node in the graph, and finds the synaptic weight matrices by solving a set of linear separation problems. We test gFTP performance by constructing networks with random dynamics, continuous attractor-like dynamics that encode position in 2-dimensional space, and discrete attractor dynamics. We then show how gFTP can be employed as a tool to explore the broad dependencies between structure and function, and the specific dependencies subserving the algorithms instantiated in the network activity.
更多查看译文
关键词
neural networks,dynamics,pre-specified
AI 理解论文
溯源树
样例
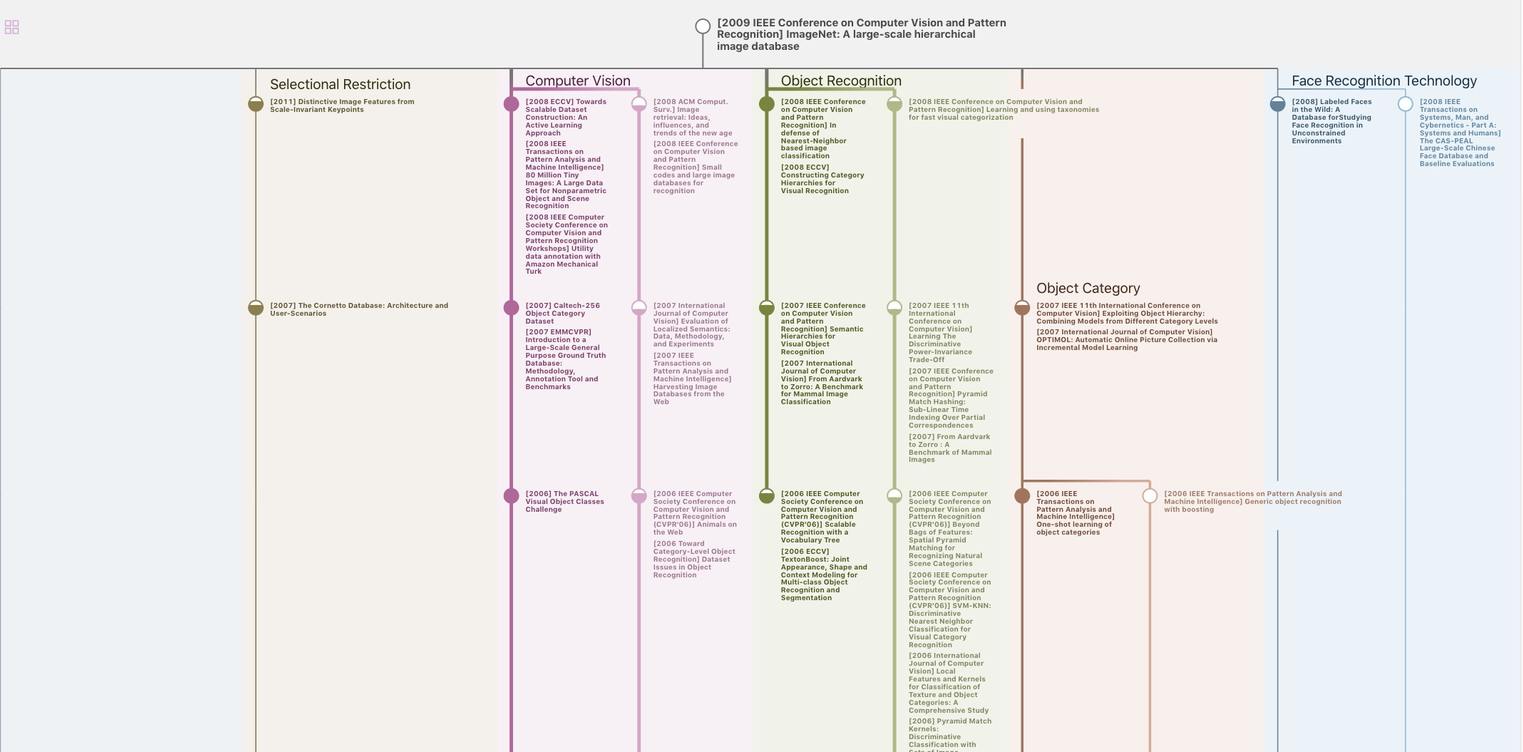
生成溯源树,研究论文发展脉络
Chat Paper
正在生成论文摘要