Abstract 5392: Universal immunohistochemistry positivity classification of cancer cells across multiple cancer types and antibodies using artificial intelligence
Cancer Research(2023)
摘要
Abstract Background: Novel cancer therapeutic antibodies targeting tumor-specific antigens are in development. However, precise quantification of target protein expression on Tumor Cells (TC) from immunohistochemistry (IHC) is challenging. Here, we developed an artificial intelligence powered image analyzer, called Universal IHC (UIHC), which robustly detects and quantifies targets of interest expressed in multiple untrained cancer types and antibodies. Methods: UIHC model can detect TCs and classify IHC positivity across many antibody-cancer pairs, called “domains”. UIHC is trained on four domains: lung cancer (569K/107K) (No. of negative TC/positive TC), bladder cancer (270K/116K), and breast cancer stained with PD-L1 22C3 pharmDx (270K/96K), and breast cancer stained with HER2 4B5 (307K/256K); all annotated by board-certified pathologists. For comparison, we developed four AI models using only a single domain (SD). All models were evaluated on hold-out test sets divided in three groups: “in-domain” included the four training domains; “near-out-domain” contained PD-L1 SP142 and SP263 on lung cancer; “far-out-domain” contained 7 antibodies on 15 cancer types. Results: For cell detection, the harmonic means of the precision and recall (F1 score) averaged on TC- and TC+ (mF1), formatted as (UIHC; avg-SD [min, max]) were: “in-domain” (72.5%; 68.9% [67.1%-69.9%]), “near-out-domain” (64.8%; 61.6% [59.7%-65.2%]), and “far-out-domain” (70.3%; 62.5% [59.0%-65.9%]). At image level, cutoffs at 1% and 50% of TPS are applied for PD-L1 22C3 and SP263, thus 3-way accuracy is computed*. In far-out-domain, the mean TPS per antibody is used as a cutoff. UIHC outperforms SD on most antibodies (Table 1). Conclusion: We demonstrated that a UIHC, built by merging multiple domains during development, can be effective on untrained domains. UIHC can be applied to novel targets in future clinical research with minimal or no adaptation needed. Model performance of the proposed UIHC model and four SD models. *3-way acc 1%-50% TPS cutoff Hold-out test-set Cell detection performance(mF1) Tumor Proportion Score (TPS) classification performance(accuracy) Test set group Staining(antibodies) Cancer type Number of negative TC/positive TC UIHC model SD modelsAverage[min-max] UIHC model SD modelsAverage[min-max] In-domain test set PD-L1 22C3 Lung 37716/13792 76.7% 71.5%*[65.6%-75.9%] 80.8%* 77.3%*[75.7%-78.7%] Near-out-domaintest set PD-L1 SP263 Lung 524/485 64.1% 64.0%[57.6%-72.6%] 80.0%* 72.5%*[60.0%-80.0%] Far-out-domain test set TROP2 Pan-cancer 1243/3289 78.9% 70.7%[63.7%-78.4%] 94.0% 87.8%[87%-90%] MET 3493/1134 80.5% 77.7%[75.1%-80.4%] 96.0% 96.0%[92.0%-100.0%] Claudin18.2 2927/74 72.7% 63.4%[55.1%-73.3%] 94.0% 93.8%[81.3%-100.0%] DLL3 2973/3 68.9% 42.5%[40.3%-43.8%] 100% 92.4%[87.5%-94.0%] HER3 2768/138 60.9% 54.2%[44.0%-71.3%] 94.0% 93.9%[87.5%-88.0%] FGFR2 2358/438 50.8% 58.9%[52.8%-58.9%] 81.0% 87.9%[87.5%-88.0%] Ecadherin 443/912 79.2% 74.6%[72.1%-76.7%] 90.0% 82.5%[80.0%-90.0%] Citation Format: Biagio Brattoli, Mohammad Mostafavi, Sangjoon Choi, Taebum Lee, Seokhwi Kim, Wonkyung Jung, Soo Ick Cho, Jinhee Lee, Keunhyung Chung, Jeongun Ryu, Seonwook Park, Sergio Pereira, Seunghwan Shin, Chan-Young Ock. Universal immunohistochemistry positivity classification of cancer cells across multiple cancer types and antibodies using artificial intelligence. [abstract]. In: Proceedings of the American Association for Cancer Research Annual Meeting 2023; Part 1 (Regular and Invited Abstracts); 2023 Apr 14-19; Orlando, FL. Philadelphia (PA): AACR; Cancer Res 2023;83(7_Suppl):Abstract nr 5392.
更多查看译文
关键词
universal immunohistochemistry positivity classification,multiple cancer types,cancer cells,antibodies
AI 理解论文
溯源树
样例
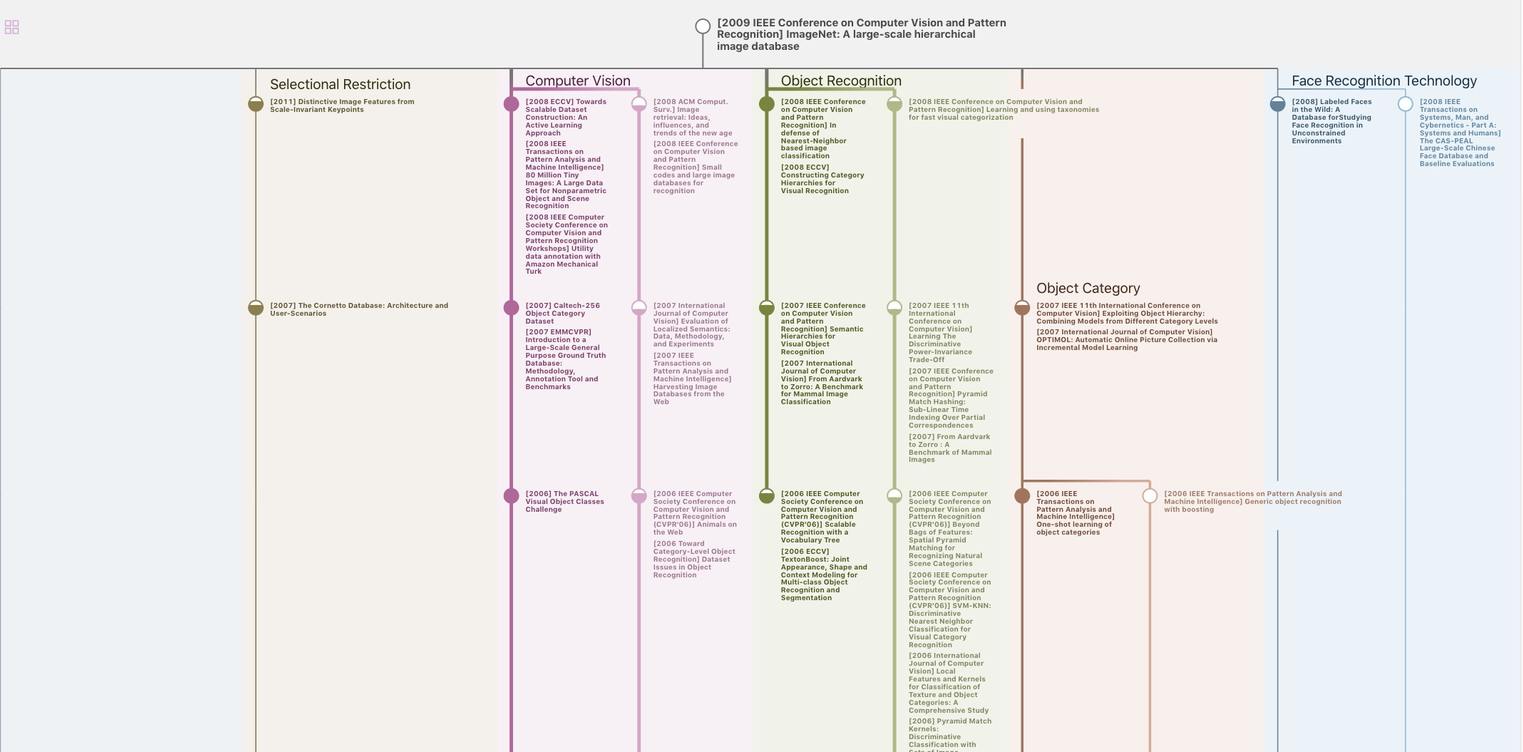
生成溯源树,研究论文发展脉络
Chat Paper
正在生成论文摘要