Contextual learning improves forest above-ground biomass estimates from UAV-LiDAR: use of tree trait associations.
EarthArXiv (California Digital Library)(2023)
摘要
Forest structure analysis and biomass prediction systems are key tools for advancing forest trait-based ecology and ecosystem stewardship. The combination of near-field remote sensing techniques---e.g. Unmanned Aerial Vehicles (UAV) and Light Detection and Ranging (LiDAR) systems---with machine learning (ML) methods enhances the accuracy of tree trait prediction and above ground-biomass~(AGB) estimates. In this study, we utilized a UAV-LiDAR system to map the 3D architecture of a Norway spruce forest in Davos, Switzerland, where a field-based inventory served as ground truth data. The objectives of this study were (i) to gain insights into variation and gradients of tree height and (ii) to evaluate whether this knowledge of community structure may prove useful as contextual information to improve predictions of AGB at the individual tree level. To investigate the local association of structural traits, we segmented the point cloud data scene into individual trees and treated tree height as the morphological variable of interest. We then used local indicators of spatial association to determine the extent of significant local context, and defined tree neighborhoods within the forest. Then, we extracted metrics from the tree neighborhoods and introduced them in a ML regression pipeline to evaluate predictions of individual tree diameter. We set up a regression experiment where the focus is on comparing performance of predictions of tree diameter between the exact same models, either considering neighborhood metrics (i.e. context-aware models), or not. Next, AGB is estimated from tree height derived from UAV-LiDAR, predicted tree diameter and allometry. The benefits of context awareness are assessed in terms of accuracy gained in predicting AGB. For the task of DBH regression, we obtained results of different machine learning methods (i.e. AdaBoost, Lasso and Random Forest) and evaluated these based on nested cross-validation. We applied this approach to two separate tree data sets within the same site, one being clustered and continuous, the other discontinuous and scattered in separate sampling plots. In both cases, we found evidence of enhanced AGB prediction performance in context-aware regressions---RMSE was reduced by 4.0\% and by 9.1\%, respectively. These findings indicate that gradients in morphological tree traits across the ecosystem proxy for unveiled ecological information that influence tree growth, which can be leveraged to enhance predictions of AGB.
更多查看译文
关键词
forest,tree trait associations,contextual learning,above-ground,uav-lidar
AI 理解论文
溯源树
样例
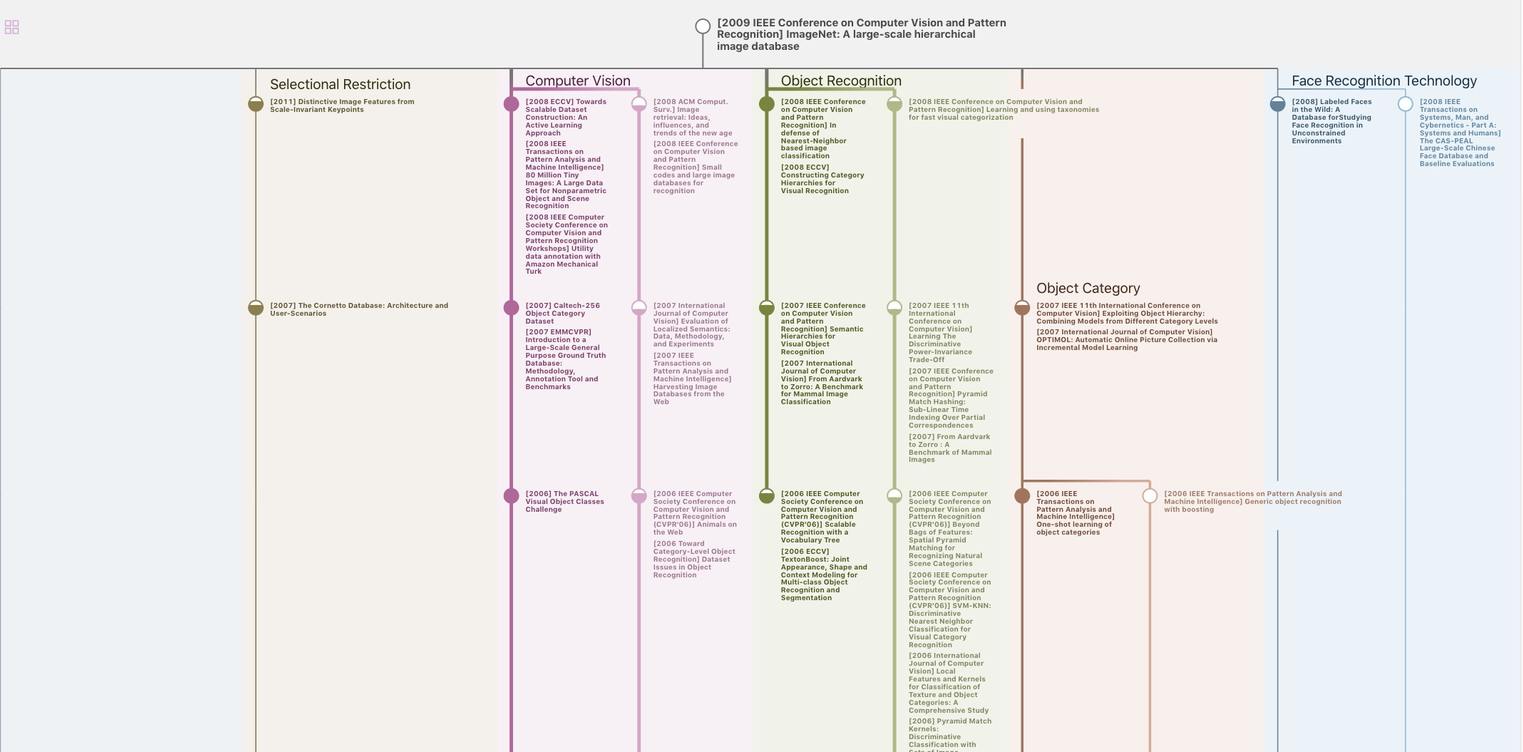
生成溯源树,研究论文发展脉络
Chat Paper
正在生成论文摘要