A Total-Body Ultra-Low Dose PET Reconstruction Method via Image Space Shuffle U-Net and Body Sampling
IEEE transactions on radiation and plasma medical sciences(2023)
摘要
Low dose PET reconstruction algorithms manage to reduce the injected dose and/or scanning time in PET examination while maintaining the image quality, and thus has been extensively studied. In this paper, we proposed a novel ultra-low dose reconstruction method for total-body PET. Specifically, we developed a deep learning model named ISS-Unet based on U-Net and introduced 3D PixelUnshuffle/PixelShuffle pair in image space to reduce the training time and GPU memory. We then introduced two body sampling methods in the training patch preparation step to improve the training efficiency and local metrics. We also reported the misalignment artifacts that were often neglected in 2D training. The proposed method was evaluated on the MICCAI 2022 Ultra-low Dose PET Imaging Challenge dataset and won the first prize in the first-round competition according to the comprehensive score combining global and local metrics. In this paper, we disclosed the implementation details of the proposed method followed by the comparison results with three typical methods.
更多查看译文
关键词
tomographic image reconstruction,image analysis,positron emission tomography,low dose,deep learning
AI 理解论文
溯源树
样例
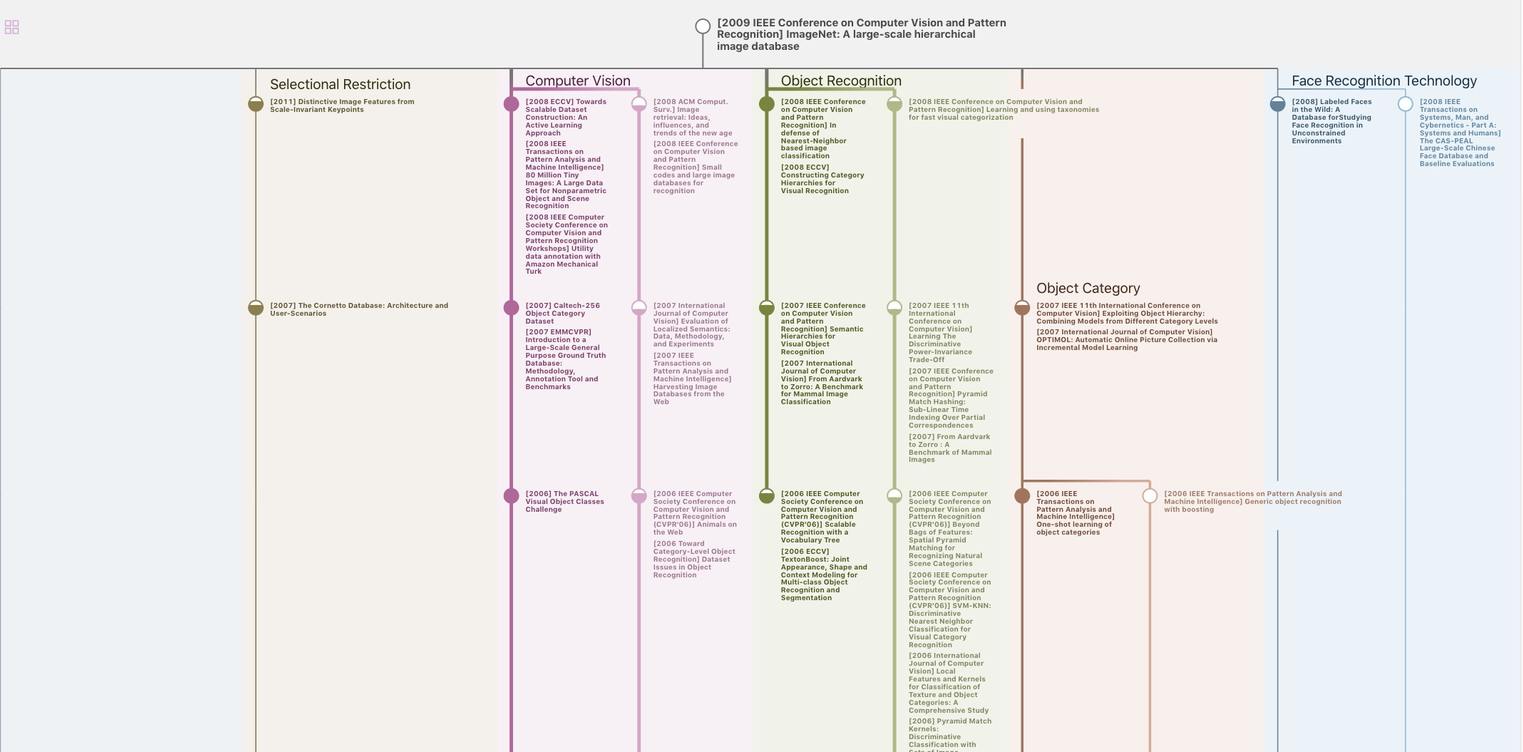
生成溯源树,研究论文发展脉络
Chat Paper
正在生成论文摘要