MERRC: A Memristor-Enabled Reconfigurable Low-Power Reservoir Computing Architecture at the Edge
IEEE TRANSACTIONS ON CIRCUITS AND SYSTEMS I-REGULAR PAPERS(2024)
摘要
The massive growth in the Internet of Things (IoT) has led to an increase in demand for devices receiving and transmitting data to and from the cloud during operations. Edge computing has been developed in an attempt to bring computations in proximity to the devices to overcome latency and cost overhead. IoT applications heavily reliant on machine learning (ML) tasks such as image or voice recognition can benefit from edge devices that facilitate real-time operations without costly data transmission back and forth from memory. In this work, we develop MERRC, a memristor-enabled reconfigurable architecture that incorporates processing-in-memory and reservoir computing to carry out ML tasks of image classification at the edge. This design uses a novel masking circuit to allow for image segmentation, which is combined with a delay-based reservoir to form a recurrent neural network. We further implement the final stage of classification using a fabricated memristor crossbar. Our hardware measurement results on the MNIST dataset of the delay-based reservoir with memristor crossbar arrays provide a recognition accuracy of 98%. On a more complex image classification dataset of CIFAR-10, MERRC shows a high accuracy of 88%, further highlighting the edge computing capabilities of the architecture.
更多查看译文
关键词
Reservoirs,Computer architecture,Deep learning,Task analysis,Memristors,Training,Image edge detection,Edge device,memristor,image recognition,reservoir computing,processing-in-memory,analog integrated circuit design
AI 理解论文
溯源树
样例
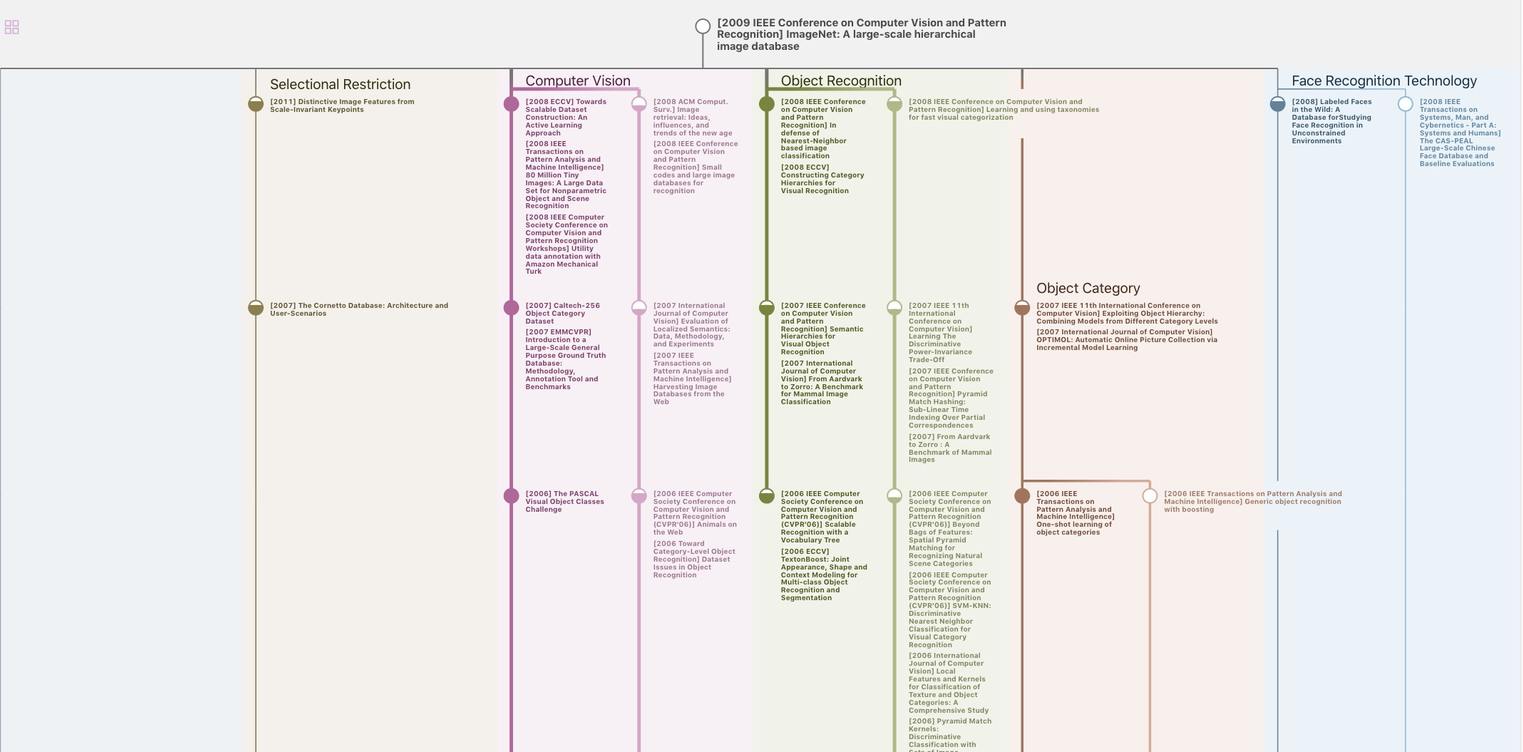
生成溯源树,研究论文发展脉络
Chat Paper
正在生成论文摘要