A Learning-and-Tube-Based Robust Model Predictive Control Strategy for Plug-In Hybrid Electric Vehicle.
IEEE Trans. Intell. Veh.(2024)
摘要
The advancement of electromechanical coupling technologies has fostered the development of four-wheel-drive plug-in hybrid electric vehicles (4WD PHEVs) with multiple power sources, potentially enhancing travel efficiency. Moreover, sophisticated energy management strategies (EMSs) not only augment the efficacy of energy-saving control but also ensure adaptability under varied driving conditions. In this paper, a learning-and-tube-based robust model predictive control (LTRMPC) strategy is proposed for a 4-wheel-drive PHEV (4WD PHEV). The suggested strategy enhances the economic efficiency of the intricate powertrain while preserving control robustness across diverse driving scenarios. Firstly, instead of utilizing a mathematical state predictive model to mirror state changes, a novel state observer is proposed, which uses a deep learning technique named Gated Recurrent Unit (GRU). The novel state observer boasts an enhanced feature fusion ability, thereby reflecting state changes accurately within the predictive horizon. Secondly, to mitigate the negative effects of state observation errors on control outcomes, a tube-based cost function is integrated into the learning-MPC framework to restrain the state changes into a certain range to further reinforce the control robustness. Finally, a simulation evaluation and hardware-in-the-loop (HIL) test validate that the proposed method can improve economic performance across various lengths of the predictive horizon and the energy-saving capability is stable compared with other baselines, showcasing its promising performance.
更多查看译文
关键词
Plug-in hybrid electric vehicles (PHEVs),learning-and-tube-based robust model predictive control (LTRMPC),gated recurrent unit (GRU),state observation,tube-based cost function
AI 理解论文
溯源树
样例
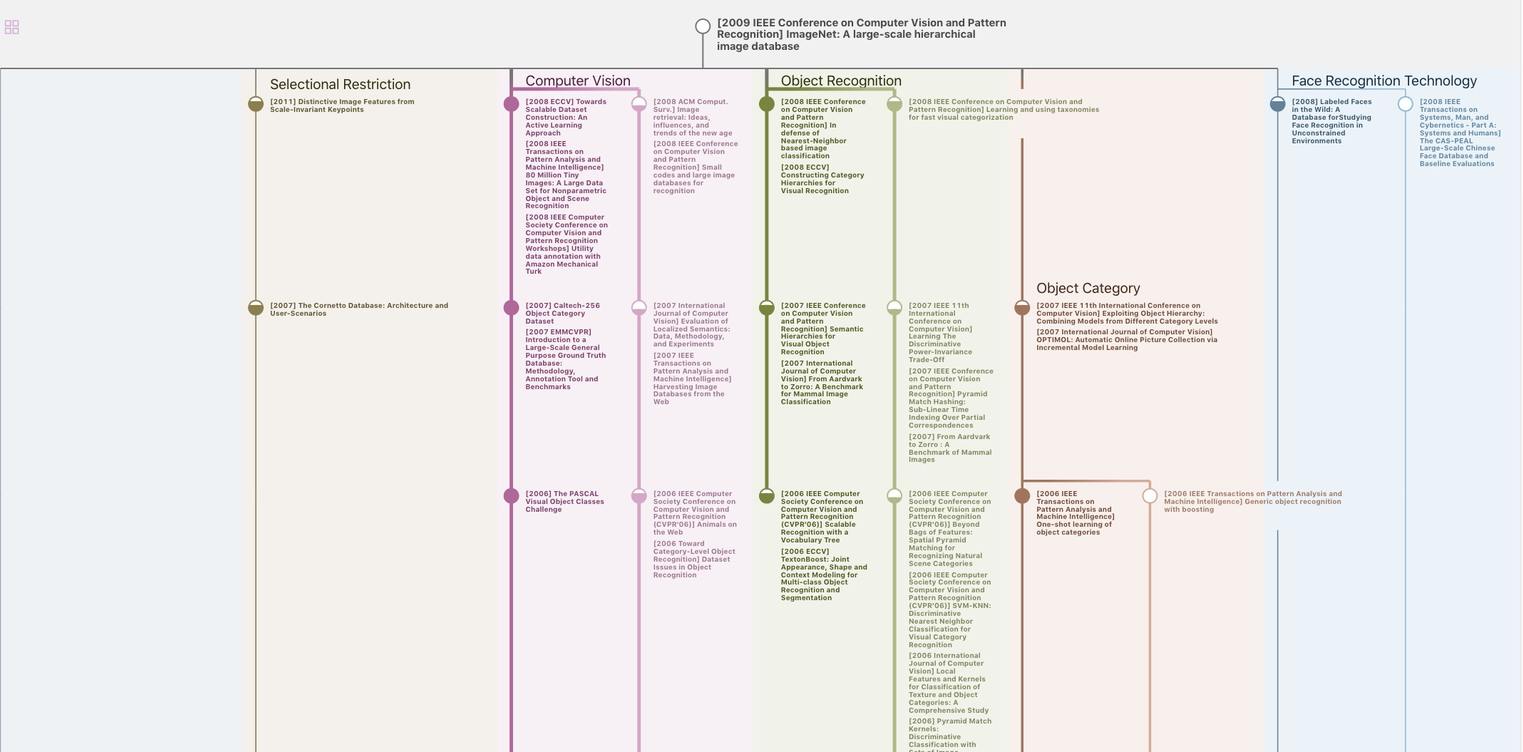
生成溯源树,研究论文发展脉络
Chat Paper
正在生成论文摘要