Effect of Microstructure on the Machinability of Natural Fiber Reinforced Plastic Composites: A Novel Explainable Machine Learning (XML) Approach
JOURNAL OF MANUFACTURING SCIENCE AND ENGINEERING-TRANSACTIONS OF THE ASME(2024)
摘要
Natural fiber-reinforced plastic (NFRP) composites are ecofriendly and biodegradable materials that offer tremendous ecological advantages while preserving unique structures and properties. Studies on using these natural fibers as alternatives to conventional synthetic fibers in fiber-reinforced materials have opened up possibilities for industrial applications, especially for sustainable manufacturing. However, critical issues reside in the machinability of such materials because of their multiscale structure and the randomness of the reinforcing elements distributed within the matrix basis. This paper reports a comprehensive investigation of the effect of microstructure heterogeneity on the resultant behaviors of cutting forces for NFRP machining. A convolutional neural network (CNN) links the microstructural reinforcing fibers and their impacts on changing the cutting forces (with an estimated R-squared value over 90%). Next, a model-agnostic explainable machine learning approach is implemented to decipher this CNN black-box model by discovering the underlying mechanisms of relating the reinforcing elements/fibers' microstructures. The presented xml approach extracts physical descriptors from the in-process monitoring microscopic images and finds the causality of the fibrous structures' heterogeneity to the resultant machining forces. The results suggest that, for the heterogeneous fibers, the tightly and evenly bounded fiber elements (i.e., with lower aspect ratio, lower eccentricity, and higher compactness) strengthen the material and thereafter play a significant role in increasing the cutting forces during NFRP machining. Therefore, the presented framework of the explainable machine learning approach opens an opportunity to discover the causality of material microstructures on the resultant process dynamics and accurately predict the cutting behaviors during material removal processes.
更多查看译文
关键词
model-agnostic explanations,microstructural feature,machine behaviors,composites,machine tool dynamics,machining processes,sensing,monitoring and diagnostics
AI 理解论文
溯源树
样例
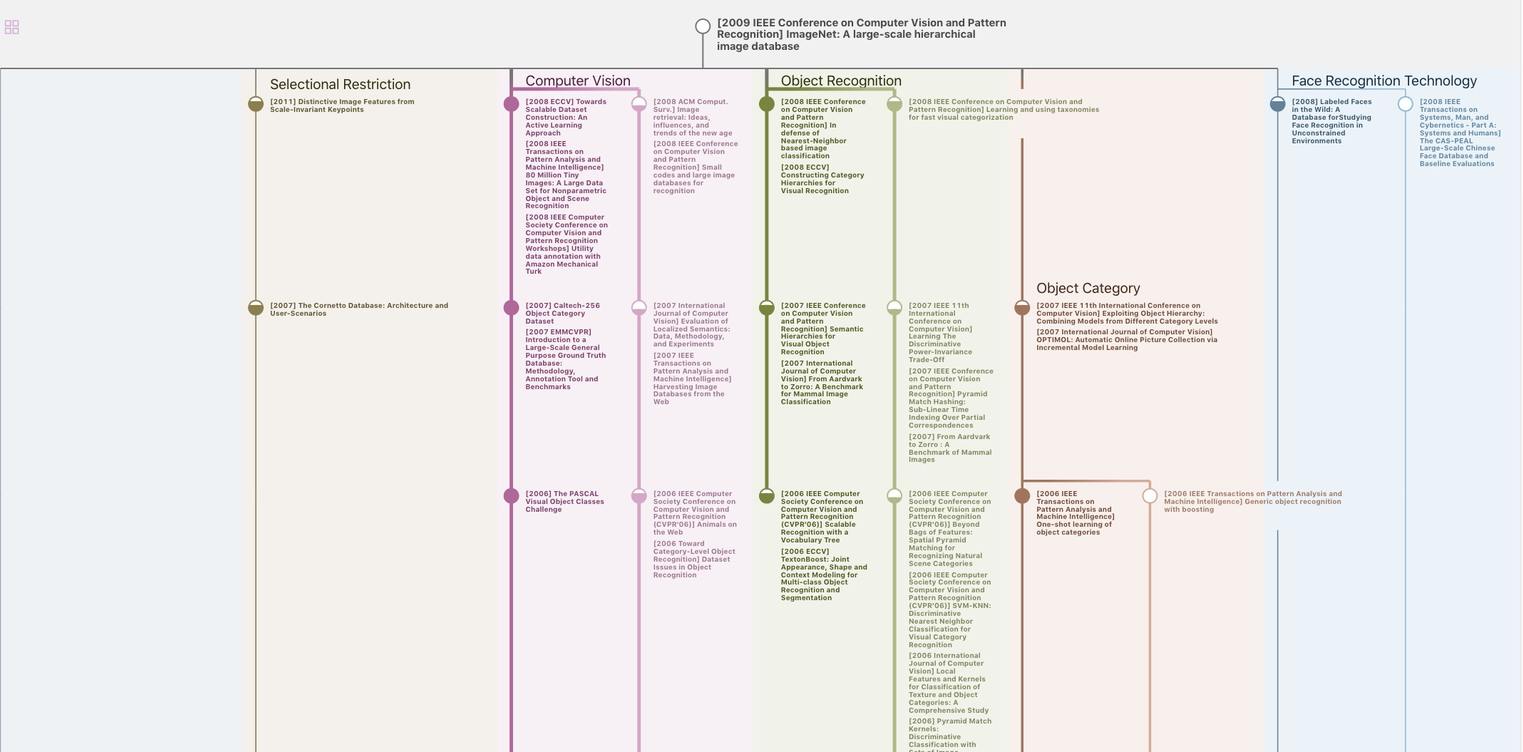
生成溯源树,研究论文发展脉络
Chat Paper
正在生成论文摘要