Online Multi-Agent Reinforcement Learning for Multiple Access in Wireless Networks
IEEE COMMUNICATIONS LETTERS(2023)
摘要
Next-generation wireless networks face a variety of challenges, including fairness problems and high access efficiency demand. The Media Access Control (MAC) layer plays a key role in improving access efficiency and ensure fairness. In this letter, we propose a new MAC protocol that utilizes multi-agent reinforcement learning (MARL) algorithm based on the multi-agent proximal policy optimization (MAPPO) to address these challenges. However, implementing a centralized training with decentralized execution (CTDE) paradigm in a MAC protocol can lead to signaling overhead issues. Therefore, we designed a joint action estimation method and periodic updating parameters scheme that effectively alleviates the communication overhead associated with CTDE. For comparison, we adopt a fully decentralized framework with low signaling overhead based on independent PPO (IPPO) algorithm. The simulation results indicate that our proposed MAPPO-MAC can outperform CSMA/CA and IPPO-MAC in both throughput and fairness with reduced communication overhead.
更多查看译文
关键词
Multiple access control protocol,online dis-tributed learning,multi-agent deep reinforcement learning
AI 理解论文
溯源树
样例
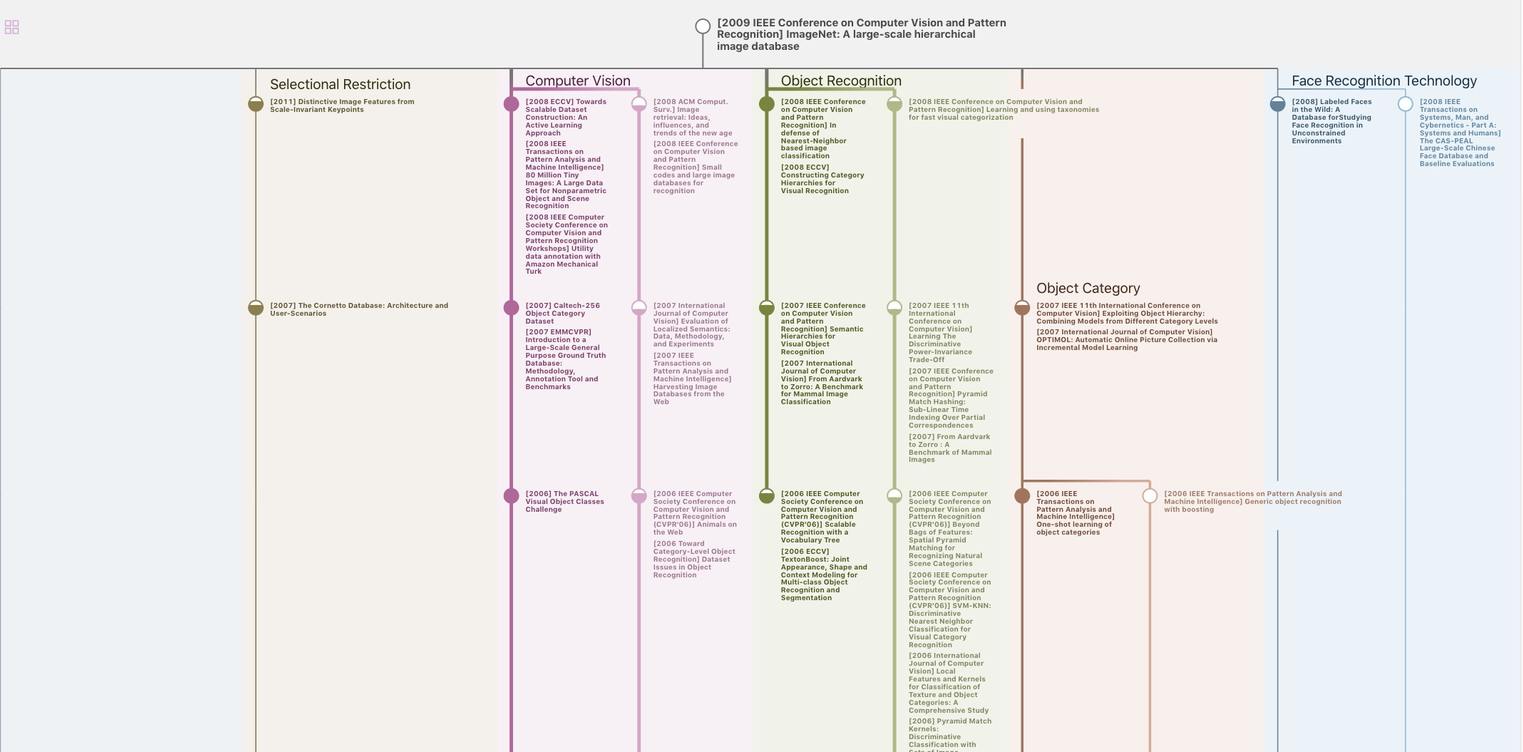
生成溯源树,研究论文发展脉络
Chat Paper
正在生成论文摘要