Leveraging Real-World Data Sets for QoE Enhancement in Public Electric Vehicles Charging Networks.
IEEE Trans. Netw. Serv. Manag.(2024)
摘要
This work targets enhancing the quality of charging experience in Electric Vehicle (EV) Public Charging Infrastructure (PCI) networks. The estimation uncertainty of waiting times at charging stations (CSs) hinders the proliferation of such networks and, hence, decelerates EV adoption. Currently, most EV owners prefer to use private chargers; thus, overloading the energy distribution network leaving PCIs under-utilized. Consequently, it becomes important for PCI operators to provide customers with accurate waiting time estimates at various CSs; therefore, allowing them to make more informed CS selections. The per-CS EV waiting times reveal possible CS overloads, which, when frequently repetitive, indicate the need for PCI up-scaling to satisfy increasing demands; hence, ensuring elevated customer QoE. This paper leverages recent real-world data to unveil the statistical properties of EV charging times that, unlike existing studies, are found to be best captured by an Erlang-k distribution. Also, the per-CS charging request arrival processes are characterized under various scheduling policies. It is established hereafter that CSs can be accurately modelled as single-server queuing systems. Finally, extensive simulations are conducted to verify the accuracy of the proposed models and provide further insights into the waiting time performance achieved by each of the adopted scheduling policies.
更多查看译文
关键词
Charging,Stations,Electric Vehicles,Infrastructure,Modelling,Performance,Waiting Time
AI 理解论文
溯源树
样例
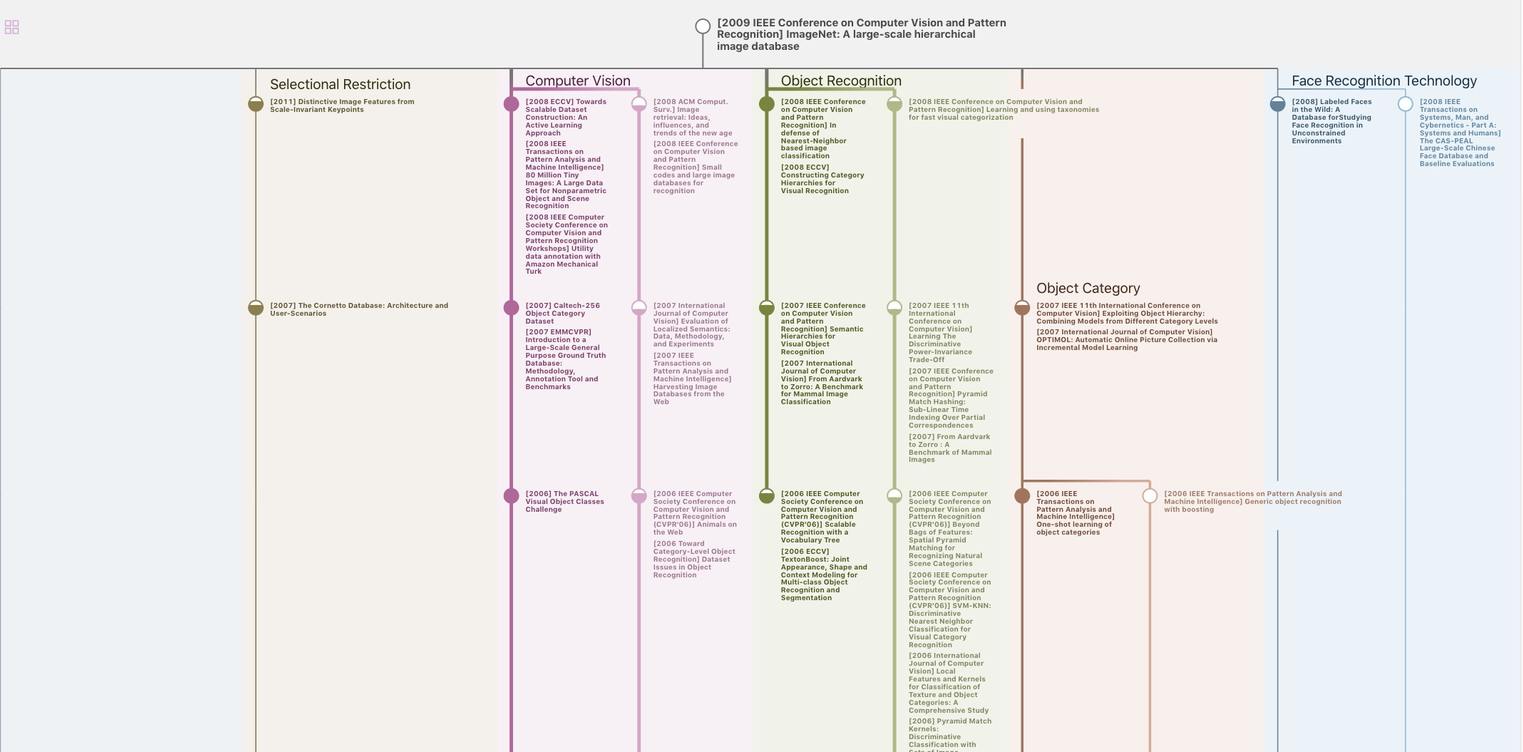
生成溯源树,研究论文发展脉络
Chat Paper
正在生成论文摘要