CCANet: A Collaborative Cross-Modal Attention Network for RGB-D Crowd Counting
IEEE TRANSACTIONS ON MULTIMEDIA(2024)
摘要
Presently, to obtain a more accurate density map and crowd number, existing methods often count by combining training RGB images and depth images. However, these methods are not ideal for capturing and fusing complementary features in RGB-D. Therefore, to solve the above problems, we propose a collaborative cross-modal attention network named CCANet for accurate RGB-D crowd counting. CCANet is mainly composed of the collaborative cross-modal attention module (CCAM) and the collaborative cross-modal fusion module (CCFM). Specifically, CCAM focuses on adaptive, interleaved RGB-D information through channel and spatial cross-modal attentions to fully capture complementary features in different modes. CCFM can adaptively integrate these features by weighing the importance of the above complementary features. A large number of experiments on the ShanghaiTechRGBD and MICC benchmarks have proven the effectiveness of CCANet in RGB-D crowd counting. In addition, our CCANet is generally applicable to multimodal crowd counting and has achieved superior counting performance on the RGBT-CC benchmark.
更多查看译文
关键词
Collaborative cross-modal attention,collaborative cross-modal fusion,crowd counting,RGB-D
AI 理解论文
溯源树
样例
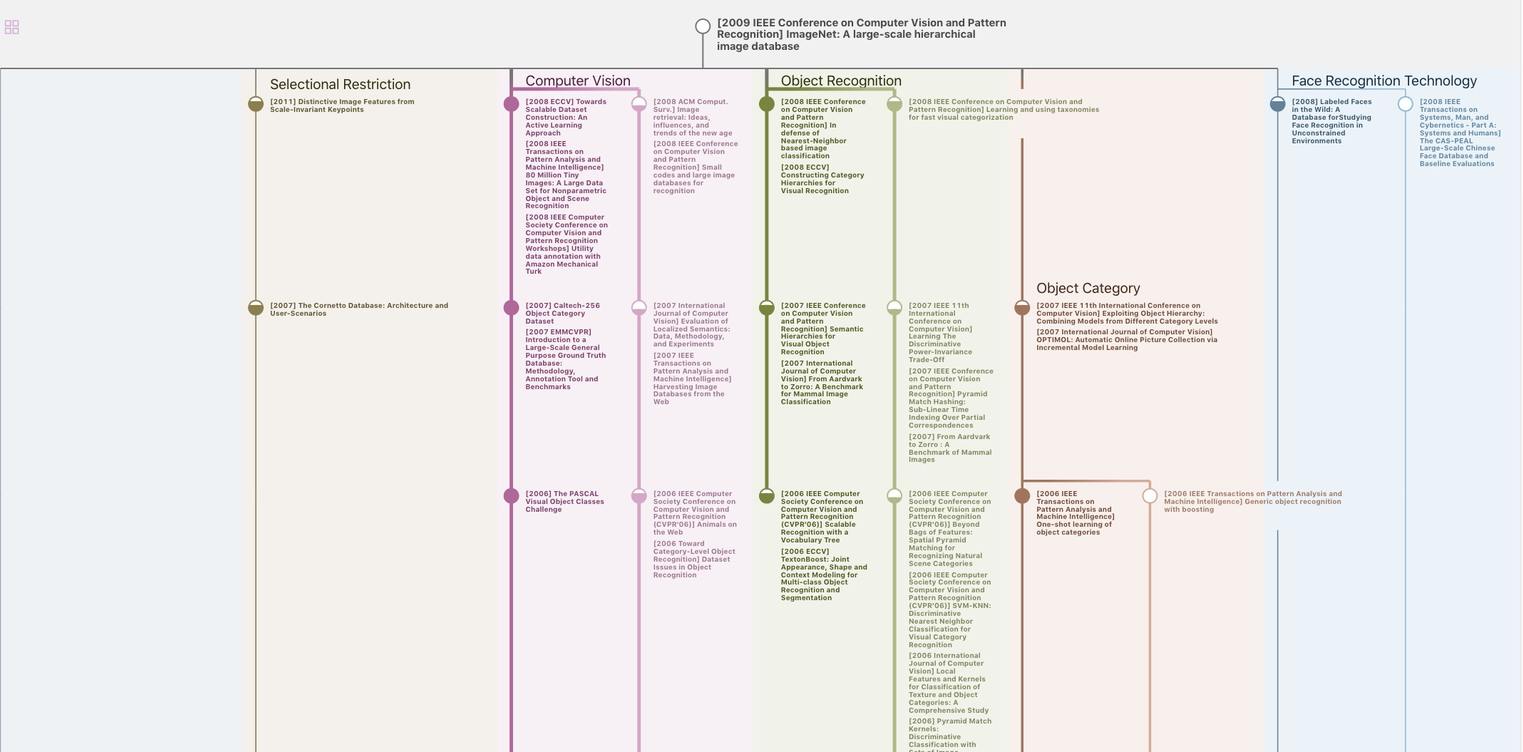
生成溯源树,研究论文发展脉络
Chat Paper
正在生成论文摘要