Tower Masking MIM: A Self-Supervised Pretraining Method for Power Line Inspection
IEEE TRANSACTIONS ON INDUSTRIAL INFORMATICS(2024)
摘要
For intelligent inspection of power lines, a core task is to detect components in aerial images. Currently, deep supervised learning, a data-hungry paradigm, has attracted great attention. However, considering real-world scenarios, labeled data are usually limited, and the utilization of abundant unlabeled data is rarely investigated in this field. This study deploys a pretrained model for power line component detection based on a self-supervised pretraining approach, which exploits useful information from unannotated data. Concretely, we design a new masking strategy based on the structural characteristic of power lines to guide the pretraining process with meaningful semantic content. Meanwhile, a Siamese architecture is proposed to extract complete global features by using dual reconstruction with semantic targets provided by the proposed masking strategy. Then, the knowledge distillation is utilized to enable the pretrained model to learn both domain-specific and general representations. Moreover, a feature pyramid mechanism is adopted to capture multiscale features, which can benefit the detection task. Experimental results show that the proposed approach can successfully improve the performance of a variety of detection frameworks for power line components, and outperforms other self-supervised pretraining methods.
更多查看译文
关键词
Component detection,deep learning,machine vision,power line inspection,self-supervised pretraining
AI 理解论文
溯源树
样例
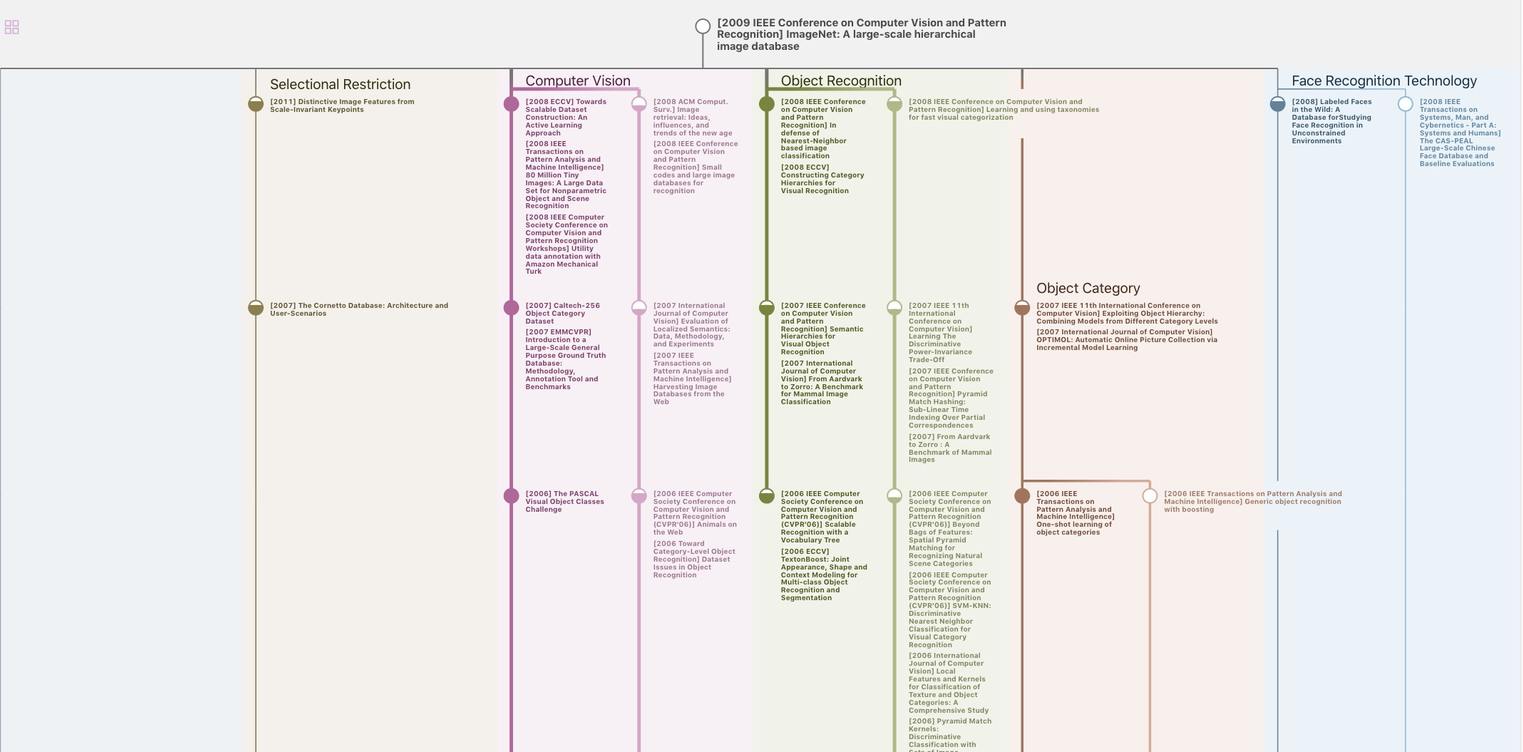
生成溯源树,研究论文发展脉络
Chat Paper
正在生成论文摘要