Metro Station functional clustering and dual-view recurrent graph convolutional network for metro passenger flow prediction
EXPERT SYSTEMS WITH APPLICATIONS(2024)
摘要
The metro system is indispensable for alleviating traffic congestion in the urban transportation system. Precise metro passenger flow (MPF) prediction is crucial in ensuring smooth operations of the metro system. Recently, the graph convolutional network (GCN), which is effective in the spatial feature extraction, has been applied in traffic prediction. However, most existing GCN-based methods construct the empirical graphs based on distance and adjacency, which cannot fully express the correlations of metro stations. This paper proposes a novel MPF prediction method consisting of three parts: K -means -based metro station functional clustering (KMSFC), external feature fusion, and dual -view recurrent GCN (DVRGCN). The KMSFC identifies the metro stations both having similar MPF changing tendencies and being located in similar urban functional areas. Furthermore, the DVRGCN is designed to simultaneously capture the spatiotemporal and external features. The dual -view GCN module in the DVRGCN captures both explicit and implicit spatial features of the metro traffic network. To demonstrate the capability for making accurate MPF predictions, the experiments using a real -world metro traffic dataset are conducted. The ablation experiments are also performed to prove the contribution of each module in the proposed method. The experimental results show that the proposed method outperforms other state-of-the-art traffic prediction methods.
更多查看译文
关键词
Metro station,Functional clustering,K-means,Passenger flow prediction,Graph convolutional network
AI 理解论文
溯源树
样例
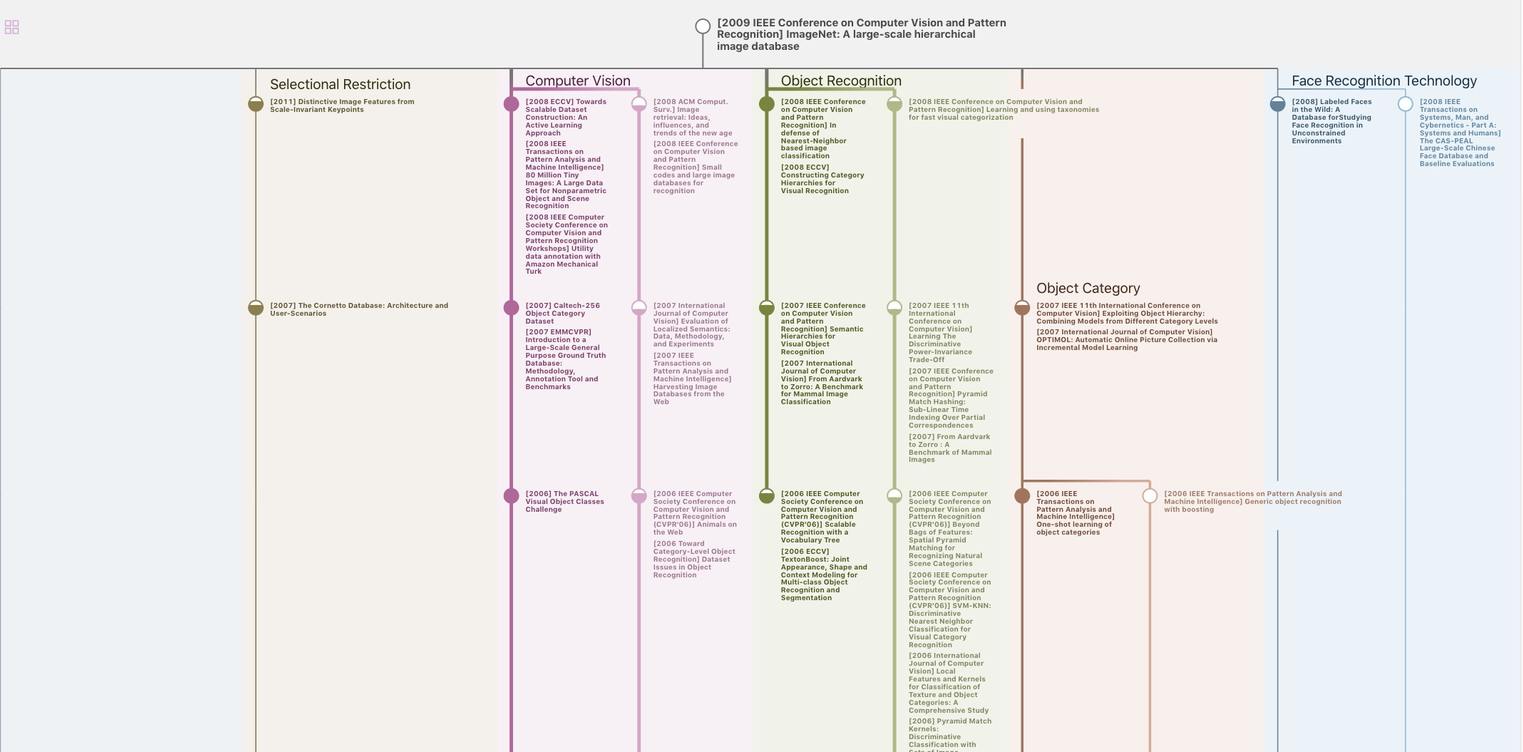
生成溯源树,研究论文发展脉络
Chat Paper
正在生成论文摘要