A New Incremental Learning for Bearing Fault Diagnosis Under Noisy Conditions Using Classification and Feature-Level Information
IEEE TRANSACTIONS ON INSTRUMENTATION AND MEASUREMENT(2024)
摘要
Deep learning has been widely used for fault diagnosis of complex mechanical equipment in recent years. However, fault types keep increasing with the change in the working state of mechanical equipment in practical scenarios, which causes the performance of traditional machine-learning models to degrade rapidly. Incremental learning can continuously learn new knowledge from incremental information while retaining previously learned knowledge, effectively enhancing the generalization performance of the model to meet the needs of fault diagnosis in actual industrial scenarios. Moreover, the noise environment will have a certain effect on the performance of the model. Therefore, this article proposes a new incremental learning method using classification and feature-level information, which aims to improve the robustness of the model under noisy conditions. First, an adaptive dual-branch residual network is constructed, and weights are assigned through an adaptive algorithm to enable the model to retain old knowledge while learning new knowledge. Then, an adversarial network is used to reduce the differences at the feature level after the samples pass through the model of different phases, which ensures the sustainable learning ability of the model and promotes knowledge transfer between different phases. Meanwhile, catastrophic forgetting is overcome through knowledge distillation loss. The comparative experimental results show that the proposed method in this article outperforms the current popular incremental learning method.
更多查看译文
关键词
Feature extraction,Fault diagnosis,Adaptation models,Data models,Knowledge engineering,Transfer learning,Task analysis,Adversarial learning,catastrophic forgetting,fault diagnosis,incremental learning,knowledge distillation
AI 理解论文
溯源树
样例
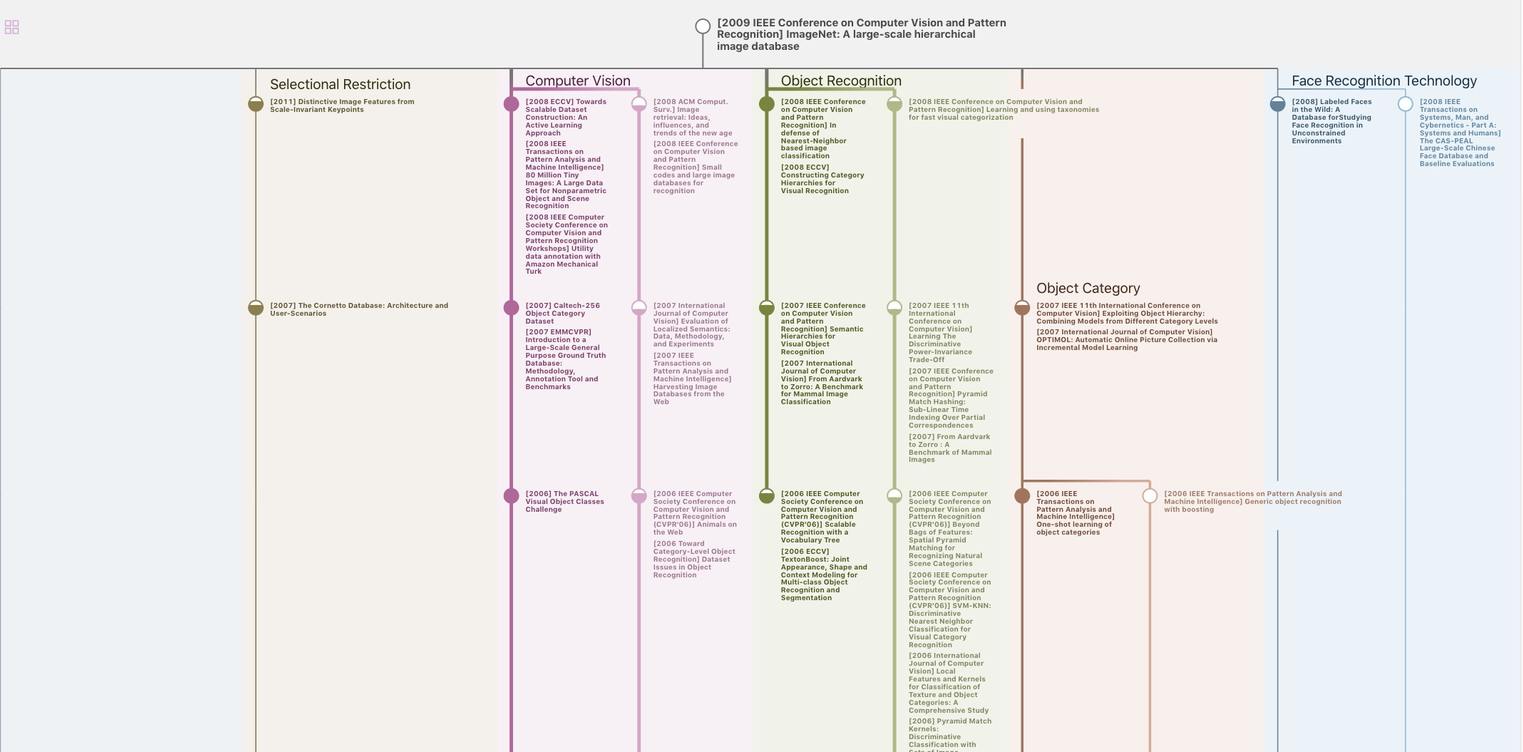
生成溯源树,研究论文发展脉络
Chat Paper
正在生成论文摘要