Radiomics-Based Machine Learning in Differentiation Between Primary Central Nervous System Lymphoma And Solitary Brain Metastasis
Research Square (Research Square)(2023)
摘要
Abstract Purpose: Primary central nervous system lymphoma (PCNSL) and solitary brain metastasis (SBM) are common malignant brain tumors. Due to distinct treatment approaches, accurately distinguishing between them preoperatively is crucial. However, conventional MRI techniques often face challenges in differentiating them due to their similar imaging appearances. This study aimed to develop and validate radiomics-derived nomogram models for distinguishing PCNSL from SBM based on conventional MRI. Methods: In this retrospective study, 324 patients diagnosed with either PCNSL (115 patients) or SBM (209 patients) from January 2011 to May 2021 were included. Conventional MRI data were processed to extract a comprehensive set of 1561 radiomics features. A two-step feature selection using LASSO regression narrowed down significant features. Twenty classification algorithms were applied to create predictive models, which were validated by receiver operating characteristic (ROC) curves. Results A total of 23 features were shortlisted by LASSO regression. Among the twenty classifiers evaluated, many displayed AUCs greater than 0.9 in both 10-fold cross-validation and independent testing. The SVMRBF classifier, in particular, showcased the highest AUC of 0.9310 in the independent test, followed closely by the RF, ANN, ELDA, and others. Conclusion Radiomics-based models, utilizing conventional MRI data, demonstrated promising potential in effectively distinguishing PCNSL from SBM. The SVMRBF classifier emerged as the most efficient in terms of diagnostic efficacy, suggesting its clinical utility in the differential diagnosis of these two entities.
更多查看译文
关键词
metastasis,machine learning,radiomics-based
AI 理解论文
溯源树
样例
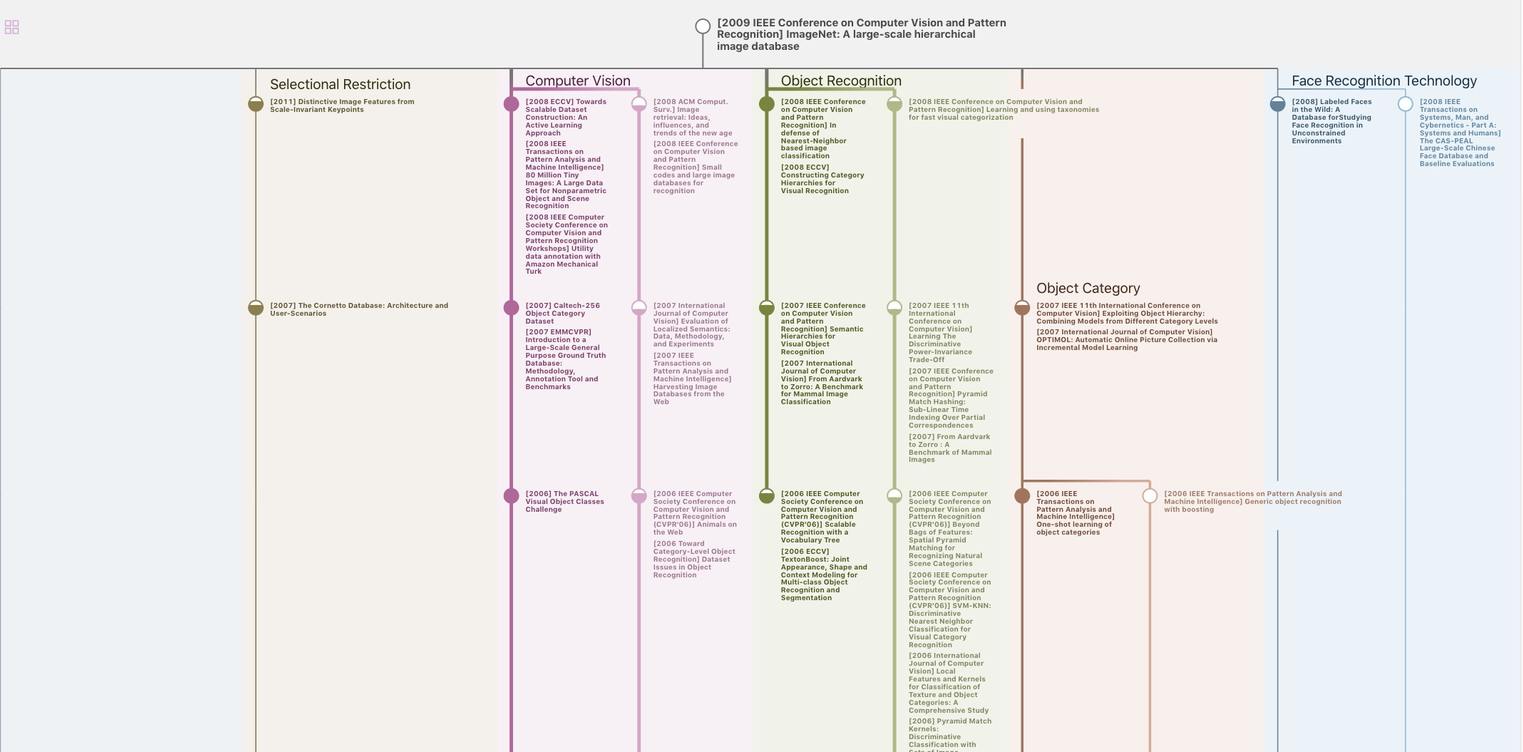
生成溯源树,研究论文发展脉络
Chat Paper
正在生成论文摘要