Fusion-Based Deep Learning Model for Automated Forest Fire Detection
CMC-COMPUTERS MATERIALS & CONTINUA(2023)
摘要
Earth resource and environmental monitoring are essential areas that can be used to investigate the environmental conditions and natural resources supporting sustainable policy development, regulatory measures, and their implementation elevating the environment. Large-scale forest fire is considered a major harmful hazard that affects climate change and life over the globe. Therefore, the early identification of forest fires using automated tools is essential to avoid the spread of fire to a large extent. Therefore, this paper focuses on the design of automated forest fire detection using a fusion-based deep learning (AFFD-FDL) model for environmental monitoring. The AFFD-FDL technique involves the design of an entropy-based fusion model for feature extraction. The combination of the handcrafted features using histogram of gradients (HOG) with deep features using SqueezeNet and Inception v3 models. Besides, an optimal extreme learning machine (ELM) based classifier is used to identify the existence of fire or not. In order to properly tune the parameters of the ELM model, the oppositional glowworm swarm optimization (OGSO) algorithm is employed and thereby improves the forest fire detection performance. A wide range of simulation analyses takes place on a benchmark dataset and the results are inspected under several aspects. The experimental results highlighted the betterment of the AFFD-FDL technique over the recent state of art techniques.
更多查看译文
关键词
Environment monitoring,remote sensing,forest fire detection,deep learning,machine learning,fusion model
AI 理解论文
溯源树
样例
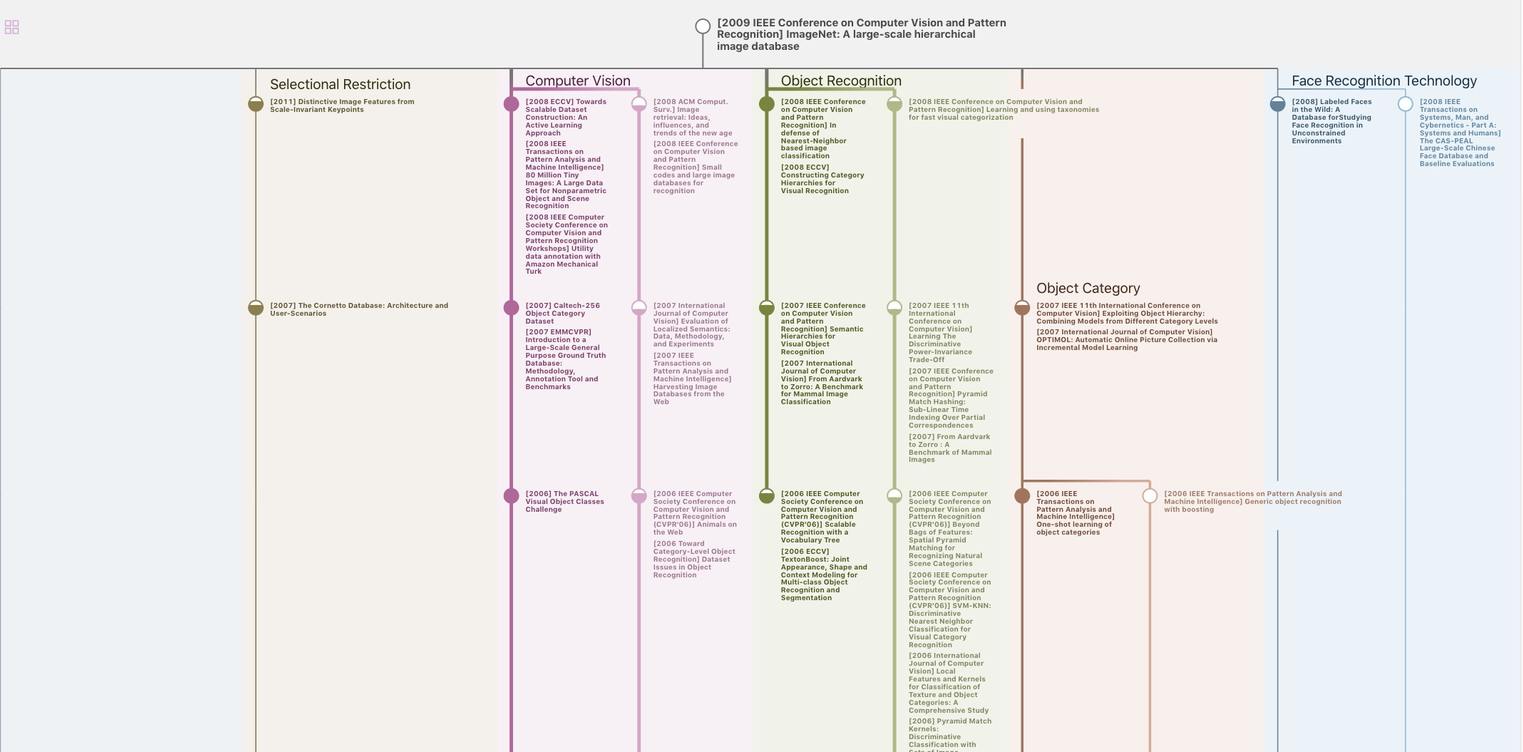
生成溯源树,研究论文发展脉络
Chat Paper
正在生成论文摘要