Accelerating Attentional Generative Adversarial Networks with Sampling Blocks
Research Square (Research Square)(2023)
摘要
Abstract Synthesizing text-to-image models for high-quality images by guiding generative models through text descriptions is an innovative and challenging task. In recent years, AttnGAN has been proposed based on the Attention mechanism to guide GAN training, which improves the details and quality of images by stacking multiple generators and discriminators. However, the combination of multiple enhancements in GAN architecture introduces redundancy, hindering the practical application of the model. These redundancies adversely affect its performance, resulting in increased inference time and space complexity. In this paper, we propose an accelerated AttnGAN (AccAttnGAN) to optimize the structure and training efficiency of AttnGAN by (1) removing redundant structures and improving the backbone network of AttnGAN; (2) integrating and reconstructing multiple losses for the training of deep attention model. Experimental results show that AccAttnGAN significantly reduces the space complexity and time complexity of the model during inference while maintaining performance. Code is available at https://github.com/jmyissb/SEAttnGAN.
更多查看译文
关键词
attentional generative adversarial networks,generative adversarial networks,sampling blocks
AI 理解论文
溯源树
样例
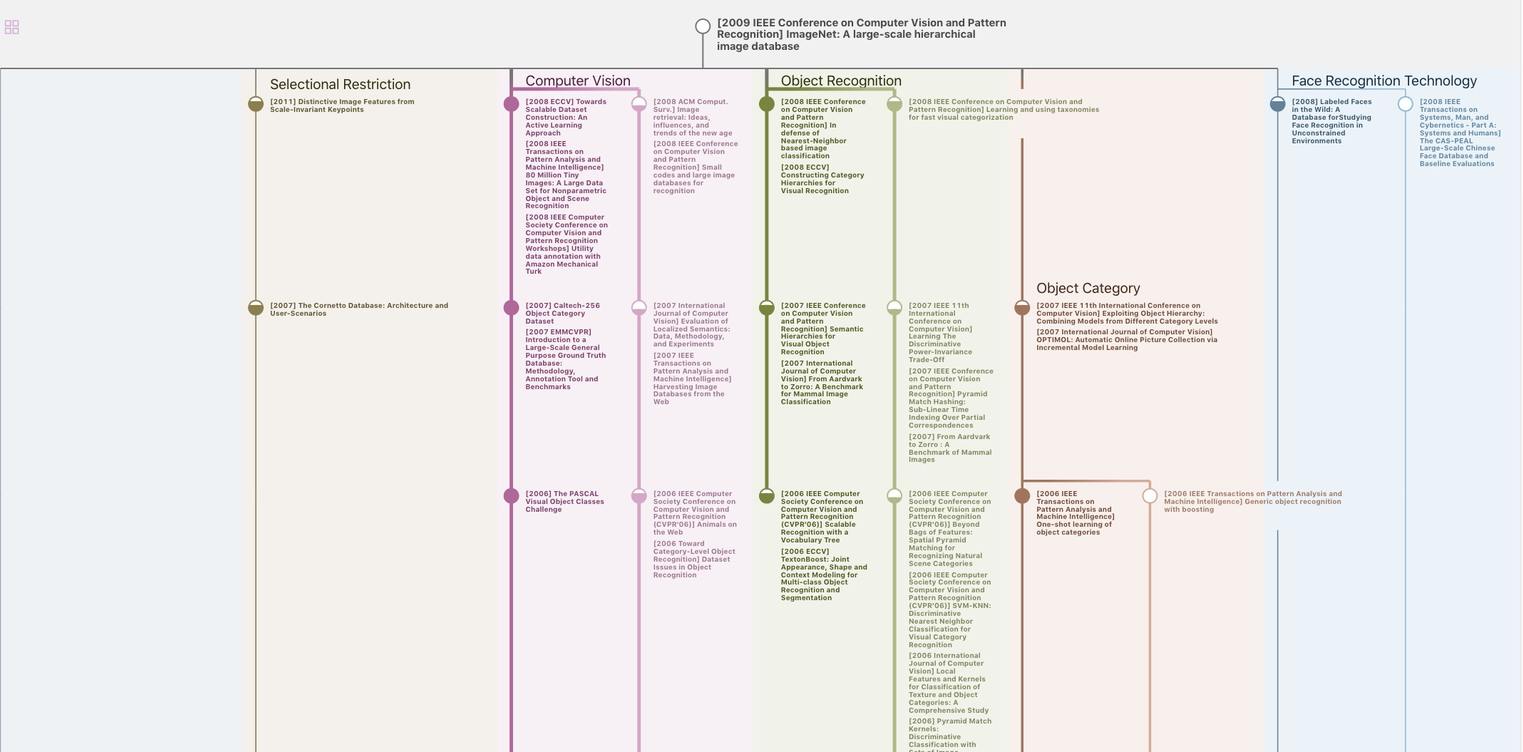
生成溯源树,研究论文发展脉络
Chat Paper
正在生成论文摘要