A Unified Innovized Progress Operator for Performance Enhancement in Evolutionary Multi- and Many-objective Optimization
IEEE Transactions on Evolutionary Computation(2023)
摘要
This paper proposes a machine learning (ML) based unified innovized progress (UIP) operator to simultaneously enhance the convergence and diversity capabilities of reference vector based evolutionary multi-and many-objective optimization algorithms, namely, RV-EMâOAs. Recent studies have demonstrated that ML intervention could help enhance convergence of RV-EMâOAs by capturing efficient search directions, through mapping of inter-generational solutions along the different reference vectors (RVs). This paper first demonstrates that ML intervention can also help enhance the diversity capability of RV-EMâOAs through mapping of intra-generational solutions across the RVs. Subsequently, the UIP operator integrates the convergence and diversity enhancement capabilities in a manner that is generic -applicable to different RV-EMâOAs, and practicable -not requiring any extra solution evaluations over the base RV-EMâOAs. Based on 24,056 experimental runs on multi-and many-objective problems, the UIP operator, when integrated with different RV-EMâOAs, has provided statistically better performance in about 36% instances, and better or equivalent in about 92% instances, compared to the respective base RV-EMâOAs.
更多查看译文
关键词
Multiobjective Optimization,Learning-assisted Optimization,Innovized Progress,Machine Learning,Innovization,Online Innovization
AI 理解论文
溯源树
样例
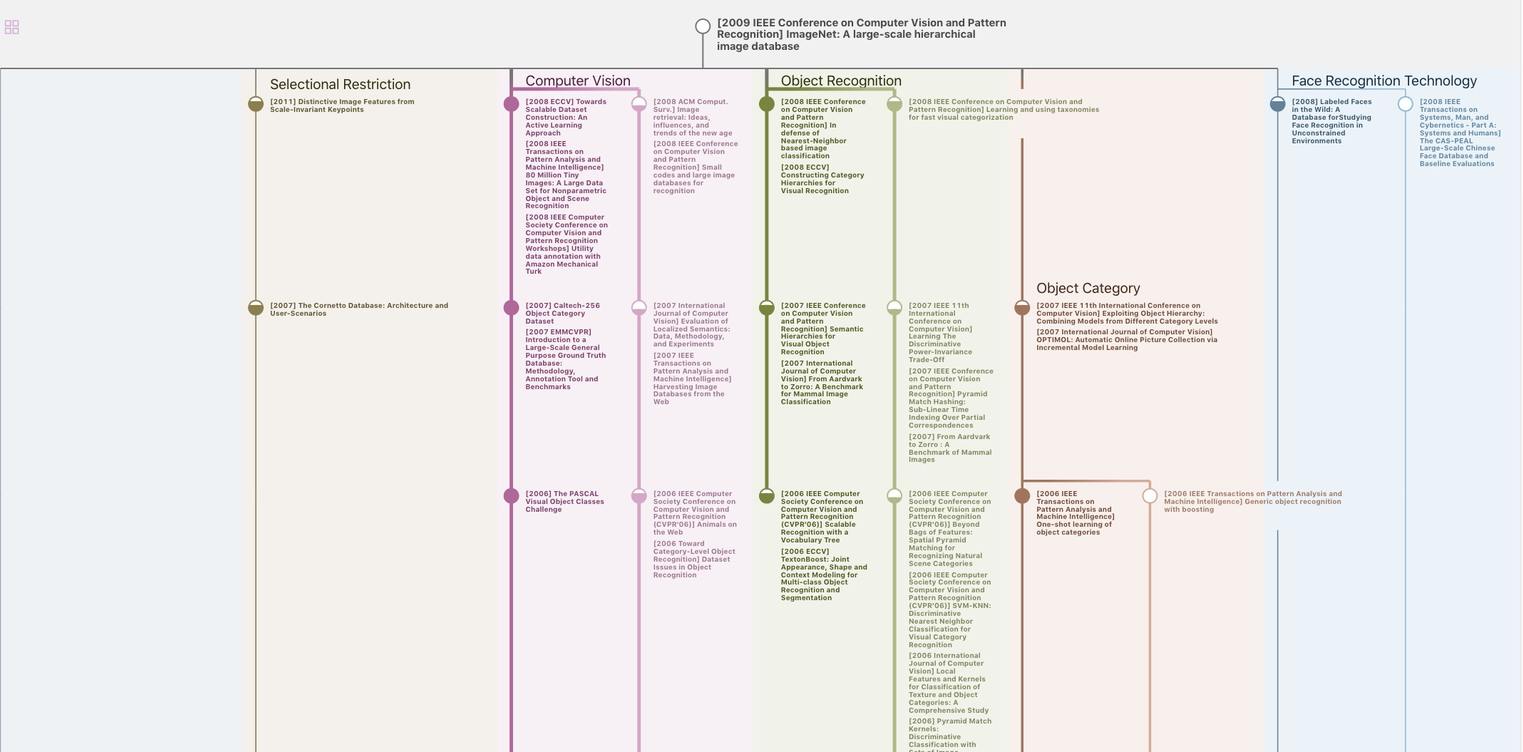
生成溯源树,研究论文发展脉络
Chat Paper
正在生成论文摘要