Progressive Source-Aware Transformer for Generalized Source-Free Domain Adaptation
IEEE TRANSACTIONS ON MULTIMEDIA(2024)
摘要
Source-free domain adaptation (SFDA) tends to forget the source domain, suffering from limitations in real-world scenarios. Recently, generalized source-free domain adaptation (GSFDA) problem naturally emerges, aiming for good performance on both target and source domains. The existing methods attempt to retain model parameters associated with the source domain to prevent such forgetting. However, this strategy is not conducive to improving cross-domain performance on the target domain, prioritizing mitigating forgetting on the source domain. This article introduces a Progressive Source-Aware Transformer approach for GSFDA, dubbed PSAT-GDA. Our core idea is to enforce the domain adaptation process to remember the source domain by imposing source guidance, offering a target domain-centric anti-forgetting mechanism. Specifically, for each epoch, a Transformer-based deep network is adapted to do domain alignment like the traditional SFDA method, because the transformer working on the image patch sequence helps to reduce image noise caused by domain shift. Meanwhile, another Transformer is designed to generate source guidance supervising domain alignment. By augmenting target sample and mining the source information from the historical models before current epoch, source injected feature group is constructed. Based on the Transformer mechanism, the attention block can select useful source information for each target sample. From it, we devise neighbour-based and augmentation-based regularizations to shape the source guidance. Experiments on three challenging datasets show that our method can achieve evident cross-domain improvement on the target domains. Also, it can mitigate forgetting on all domains after adapting to single or multiple target domains.
更多查看译文
关键词
Transformers,Adaptation models,Trajectory,Task analysis,Semantics,Self-supervised learning,Data models,Domain adaptation,mitigating forgetting,historical global attention,denoising,object cognition
AI 理解论文
溯源树
样例
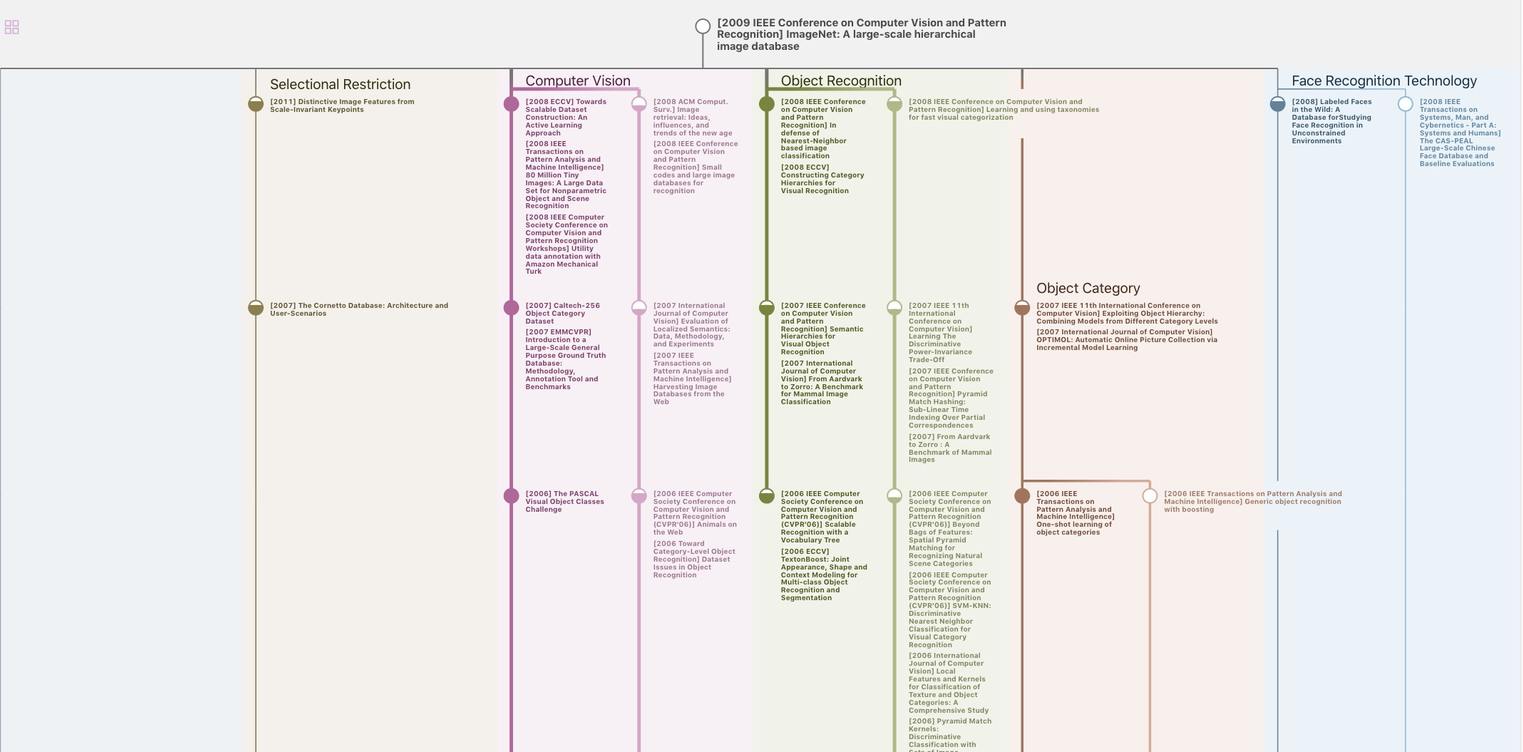
生成溯源树,研究论文发展脉络
Chat Paper
正在生成论文摘要