Leverage Learning Behaviour Data for Students' Learning Performance Prediction and Influence Factor Analysis
IEEE transactions on artificial intelligence(2023)
摘要
Online education has become increasingly significant for university students and faculty, especially in the context of the modern remote education landscape. However, the inherent space-time separation in online education can create communication delays between teachers and students, making it challenging to monitor students' behaviors effectively. In an effort to understand the connection between students' online engagement and their learning performance, data annotation has been implemented to address the issue of accurately representing students' learning behaviors. Taking the online teaching data of Shanghai Normal University platform as the research object, the primary online education problem is explored through data mining, which includes correlation analysis, Gini importance ranking and principal component analysis (PCA). Then, constructing the learning performance prediction model using random forest (RF) based on PCA by comparing various machine learning algorithms. As a consequence, the most influential online learning behaviors are course duration time, document learning time, test average score and video completion rate. The overall classification accuracy of the learning performance prediction model is 87.45%, and the highest prediction accuracy for a single category is 96.52%.
更多查看译文
关键词
Classification prediction,correlation analysis,data mining,learning behavior representation,random forest
AI 理解论文
溯源树
样例
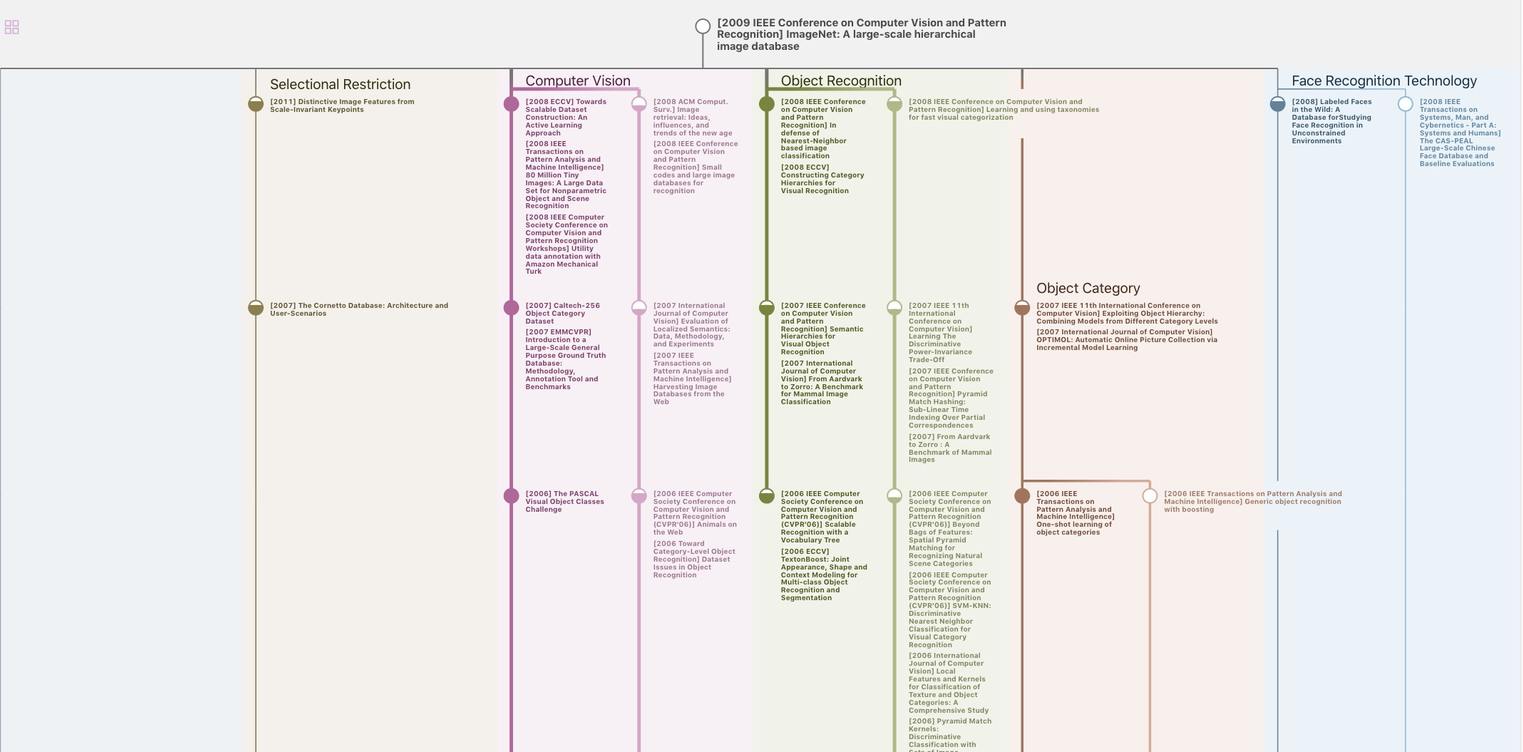
生成溯源树,研究论文发展脉络
Chat Paper
正在生成论文摘要