Predicting Standardized Uptake Value of Brown Adipose Tissue from CT scans using neural networks
Research Square (Research Square)(2023)
摘要
Abstract The standard method for identifying active Brown Adipose Tissue (BAT) is [ 18 F]-Fluorodeoxyglucose ([ 18 F]-FDG) PET/CT imaging, which is costly and exposes patients to radiation, making it impractical for population studies. These issues can be addressed with computational methods that predict [ 18 F]-FDG uptake by BAT from CT; earlier population studies pave the way for developing such methods by showing some correlation between the Hounsfield Unit (HU) of BAT in CT and the corresponding [ 18 F]-FDG uptake in PET. In this study, we propose training convolutional neural networks (CNNs) to predict [ 18 F]-FDG uptake by BAT from unenhanced CT scans in the restricted regions which are likely to contain BAT. We performed experiments on datasets from four different cohorts, the largest study to date. We segmented the BAT regions using the predicted [ 18 F]-FDG uptake values and evaluated the accuracy of the segmentations, showing 23% to 40% improvement over the conventional CT thresholding-based method. Additionally, we showed that BAT volumes computed from the segmentations could be used to distinguish subjects with and without active BAT with an AUC of 0.8, whereas the CT thresholding-based method achieved 0.6. Our findings suggest that CNNs, when trained on cold-stimulated cohorts, can be effectively used to create stratified cohorts from only CT, thereby having the potential for facilitating large-scale imaging studies with greater efficiency and lower costs.
更多查看译文
关键词
brown adipose tissue,adipose tissue,predicting scans,standardized uptake value
AI 理解论文
溯源树
样例
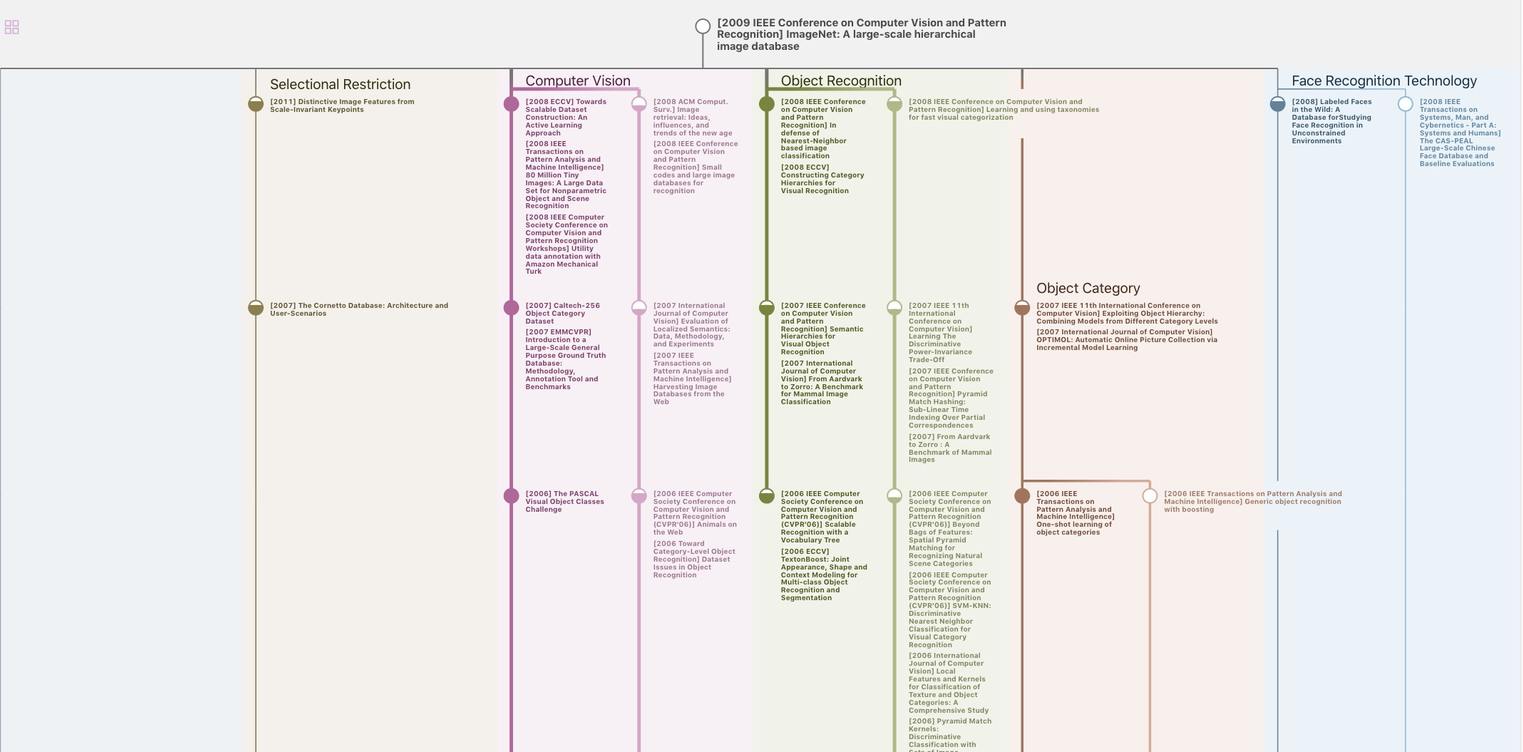
生成溯源树,研究论文发展脉络
Chat Paper
正在生成论文摘要