Deconfounding Causal Inference for Zero-Shot Action Recognition
IEEE TRANSACTIONS ON MULTIMEDIA(2024)
摘要
Zero-shot action recognition (ZSAR) aims to recognize unseen action categories in the test set without corresponding training examples. Most existing zero-shot methods follow the feature generation framework to transfer knowledge from seen action categories to model the feature distribution of unseen categories. However, due to the complexity and diversity of actions, it remains challenging to generate unseen feature distribution, especially for the cross-dataset scenario when there is a potentially larger domain shift. This article proposes a Deconfounding Ca USAl GAN (DeCalGAN) for generating unseen action video features with the following technical contributions: 1) Our model unifies compositional ZSAR with traditional visual-semantic models to incorporate local object information with global semantic information for feature generation. 2) A GAN-based architecture is proposed for causal inference and unseen distribution discovery. 3) A deconfounding module is proposed to refine representations of local objects and global semantic information confounder in the training data. Action descriptions and random object features after causal inference are then used to discover unseen distributions of novel actions in different datasets. Our extensive experiments on Cross-Dataset Zero-Shot Action Recognition (CD-ZSAR) demonstrate substantial improvement over the UCF101 and HMDB51 standard benchmarks for this problem.
更多查看译文
关键词
Semantics,Training,Task analysis,Feature extraction,Visualization,Training data,Three-dimensional displays,Zero-shot learning,action recognition,causal inference
AI 理解论文
溯源树
样例
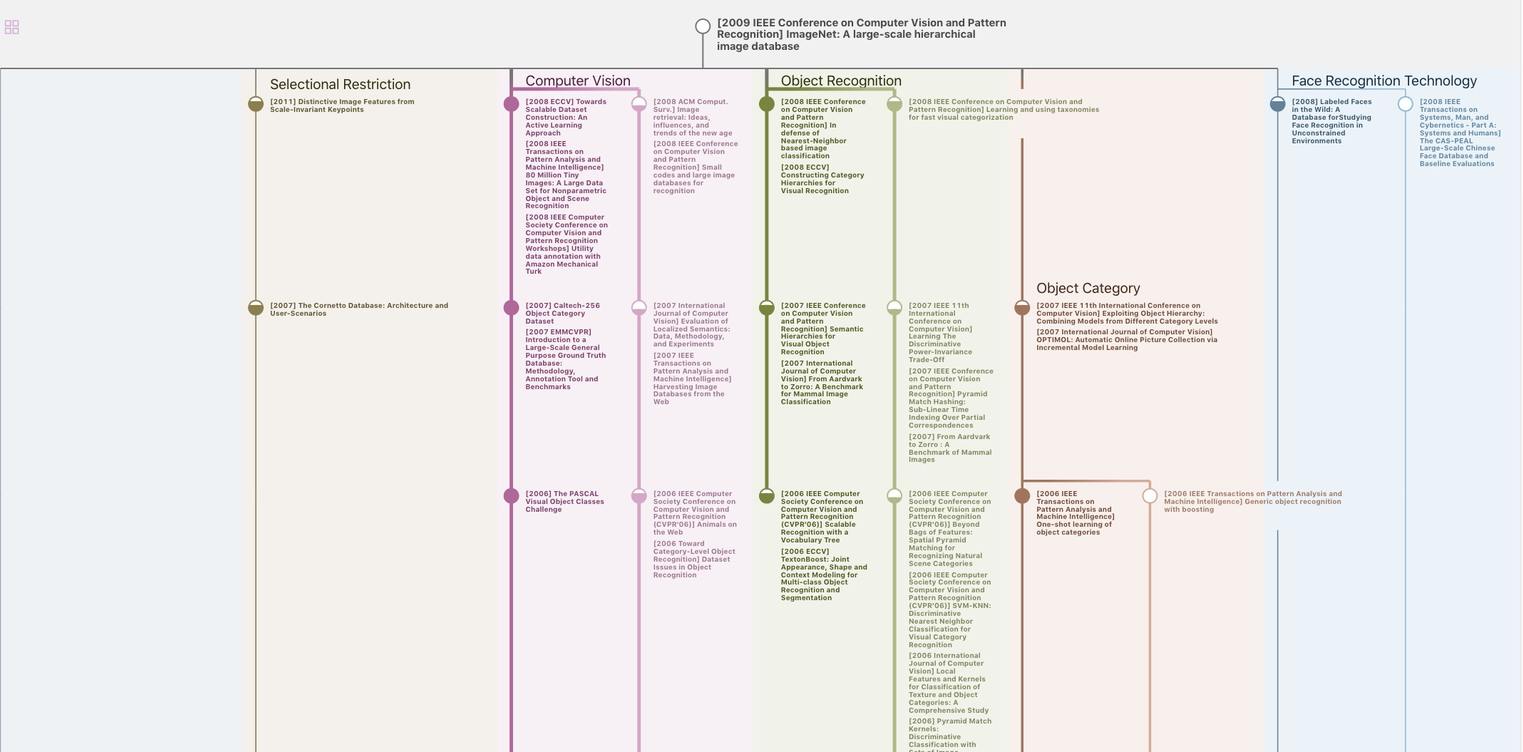
生成溯源树,研究论文发展脉络
Chat Paper
正在生成论文摘要