Deep learning-based fast denoising of Monte Carlo dose calculation in carbon ion radiotherapy.
Medical Physics(2023)
摘要
BACKGROUND:Plan verification is one of the important steps of quality assurance (QA) in carbon ion radiotherapy. Conventional methods of plan verification are based on phantom measurement, which is labor-intensive and time-consuming. Although the plan verification method based on Monte Carlo (MC) simulation provides a more accurate modeling of the physics, it is also time-consuming when simulating with a large number of particles. Therefore, how to ensure the accuracy of simulation results while reducing simulation time is the current difficulty and focus.
PURPOSE:The purpose of this work was to evaluate the feasibility of using deep learning-based MC denoising method to accelerate carbon-ion radiotherapy plan verification.
METHODS:Three models, including CycleGAN, 3DUNet and GhostUNet with Ghost module, were used to denoise the 1 × 10
68RESULTS:The three end-to-end trained models could be used for denoising the 1 × 10
688CONCLUSION:The end-to-end deep network GhostUNet outperforms the CycleGAN, 3DUNet models in denoising MC dose distributions for carbon ion radiotherapy. The network requires less than 5 s to denoise a sample of MC simulation with few particles to obtain a qualitative and quantitative result comparable to the dose distribution simulated by MC with relatively large number particles, offering a significant reduction in computation time.
更多查看译文
关键词
monte carlo dose calculation,radiotherapy,deep learning‐based
AI 理解论文
溯源树
样例
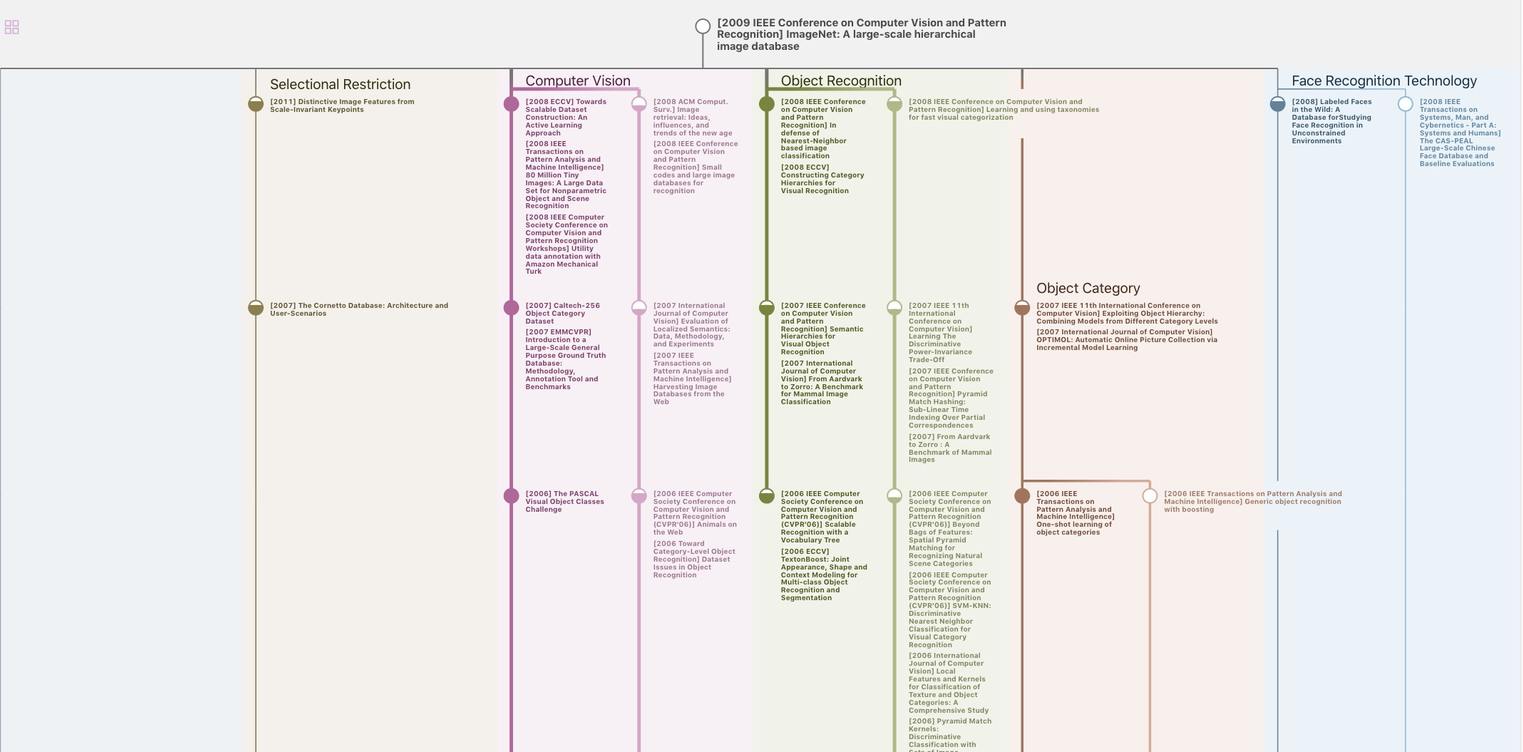
生成溯源树,研究论文发展脉络
Chat Paper
正在生成论文摘要